Efficient Uncertainty Mitigation in Automated Vehicles: Combining MPC with Model Reference Adaptive Control
IFAC PAPERSONLINE(2023)
摘要
Automated vehicles must be equipped with the ability to plan and execute trajectories in the presence of uncertainties for safe and effective navigation in both on- and off-road environments. Model predictive control (MPC) is a powerful and popular optimal control solution for both planning and control tasks, but even robust MPC solutions still suffer from decreased performance and the curse of dimensionality in the optimization problem when subject to model errors. We propose an adaptive control scheme that combines a fast, nominal MPC with our previously developed Model Reference Adaptive Control strategy in a cascaded architecture. The effectiveness of the resulting framework is demonstrated via robust and computationally efficient trajectory tracking under severe uncertainties. This study includes detailed tuning considerations and numerical evaluation with different fidelity vehicle models. Copyright (c) 2023 The Authors. This is an open access article under the CC BY-NC-ND license (https://creativecommons.org/licenses/by-nc-nd/4.0/)
更多查看译文
关键词
Automated vehicle stability,Adaptive control of automotive systems,Model reference adaptive control,Model predictive control
AI 理解论文
溯源树
样例
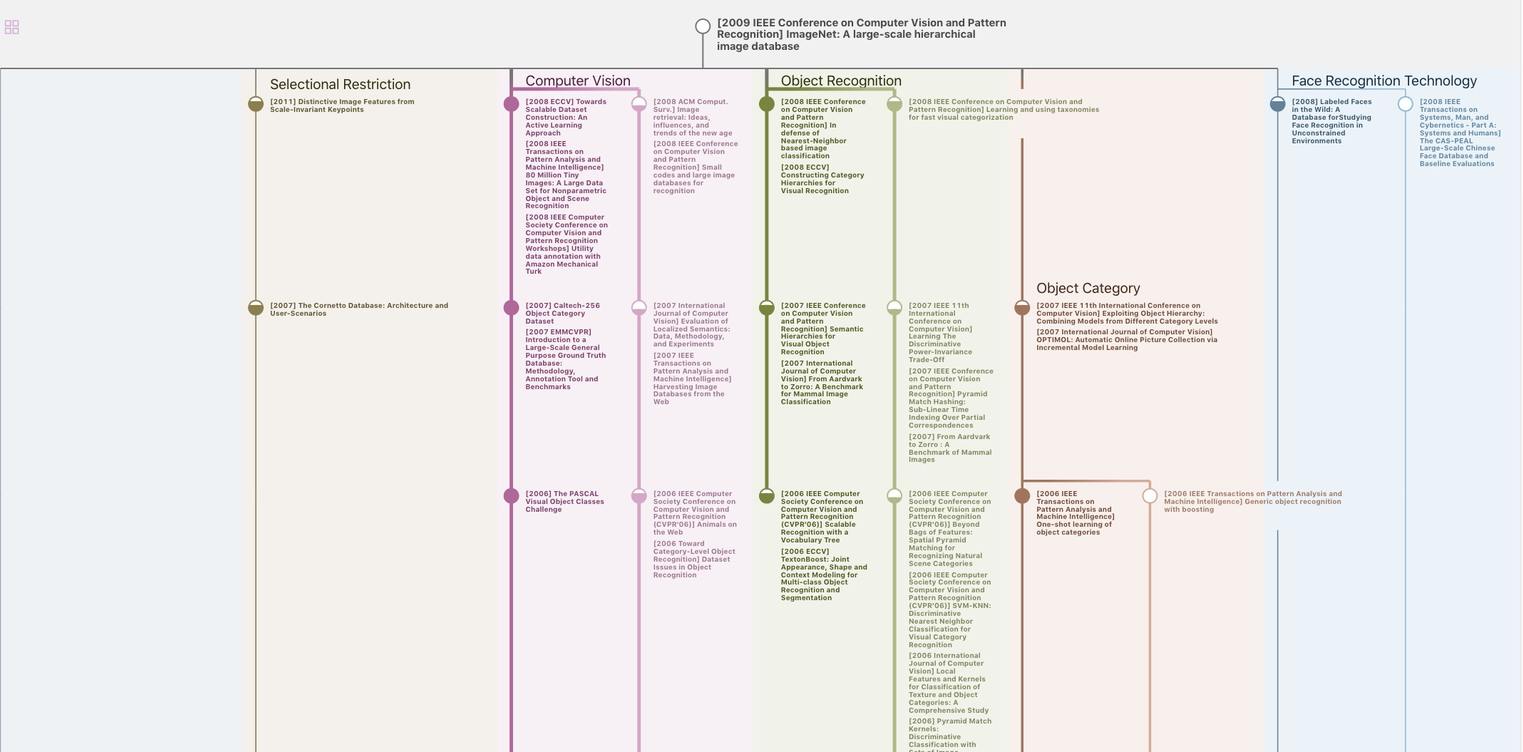
生成溯源树,研究论文发展脉络
Chat Paper
正在生成论文摘要