Privacy and Fairness in Federated Learning: On the Perspective of Tradeoff
ACM COMPUTING SURVEYS(2024)
摘要
Federated learning (FL) has been a hot topic in recent years. Ever since it was introduced, researchers have endeavored to devise FL systems that protect privacy or ensure fair results, with most research focusing on one or the other. As two crucial ethical notions, the interactions between privacy and fairness are comparatively less studied. However, since privacy and fairness compete, considering each in isolation will inevitably come at the cost of the other. To provide a broad view of these two critical topics, we presented a detailed literature review of privacy and fairness issues, highlighting unique challenges posed by FL and solutions in federated settings. We further systematically surveyed different interactions between privacy and fairness, trying to reveal how privacy and fairness could affect each other and point out new research directions in fair and private FL.
更多查看译文
关键词
Federated learning,data privacy,model fairness
AI 理解论文
溯源树
样例
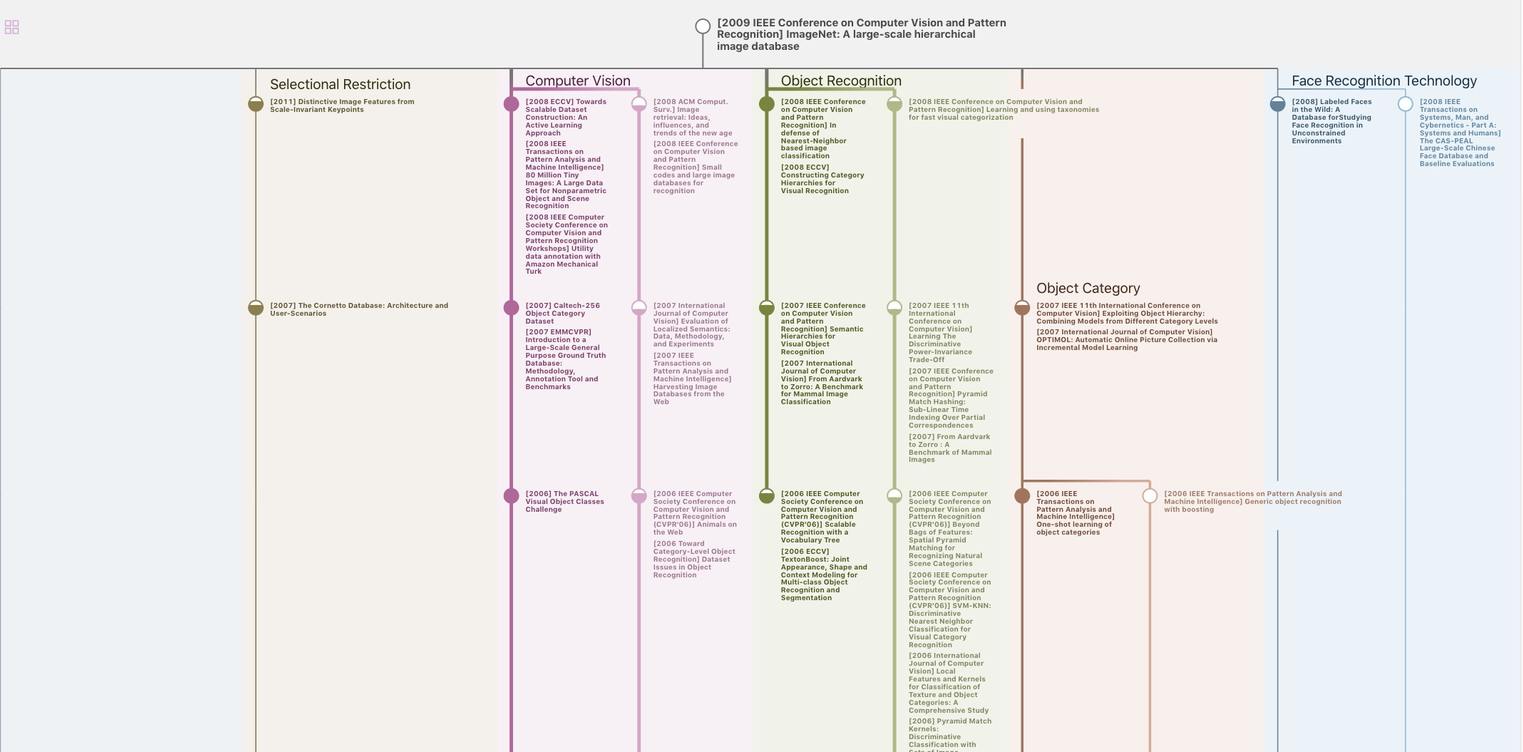
生成溯源树,研究论文发展脉络
Chat Paper
正在生成论文摘要