FAWA: Fast Adversarial Watermark Attack
IEEE TRANSACTIONS ON COMPUTERS(2024)
摘要
Recently, adversarial attacks have shown to lead the state-of-the-art deep neural networks (DNNs) to misclassification. However, most adversarial attacks are generated according to whether they are perceptual to human visual system, measured by geometric metrics such as the l(2)-norm, which ignores the common watermarks in cyber-physical systems. In this article, we propose a fast adversarial watermark attack (FAWA) method based on fast differential evolution technique, which optimally superimposes a watermark on an image to fool DNNs. We also attempt to explain the reason why the attack is successful and propose two hypotheses on the vulnerability of DNN classifiers and the influence of the watermark attack on higher-layer features extraction respectively. In addition, we propose two countermeasure methods against FAWA based on random rotation and median filtering respectively. Experimental results show that our method achieves 41.3 percent success rate in fooling VGG-16 and have good transferability. Our approach is also shown to be effective in deceiving deep learning as a service (DLaaS) systems as well as the physical world. The proposed FAWA, hypotheses, and the countermeasure methods, provide a timely help for DNN designers to gain some knowledge of model vulnerability while designing DNN classifiers and related DLaaS applications.
更多查看译文
关键词
Adversarial attacks,watermark,differential evolution,DLaaS security
AI 理解论文
溯源树
样例
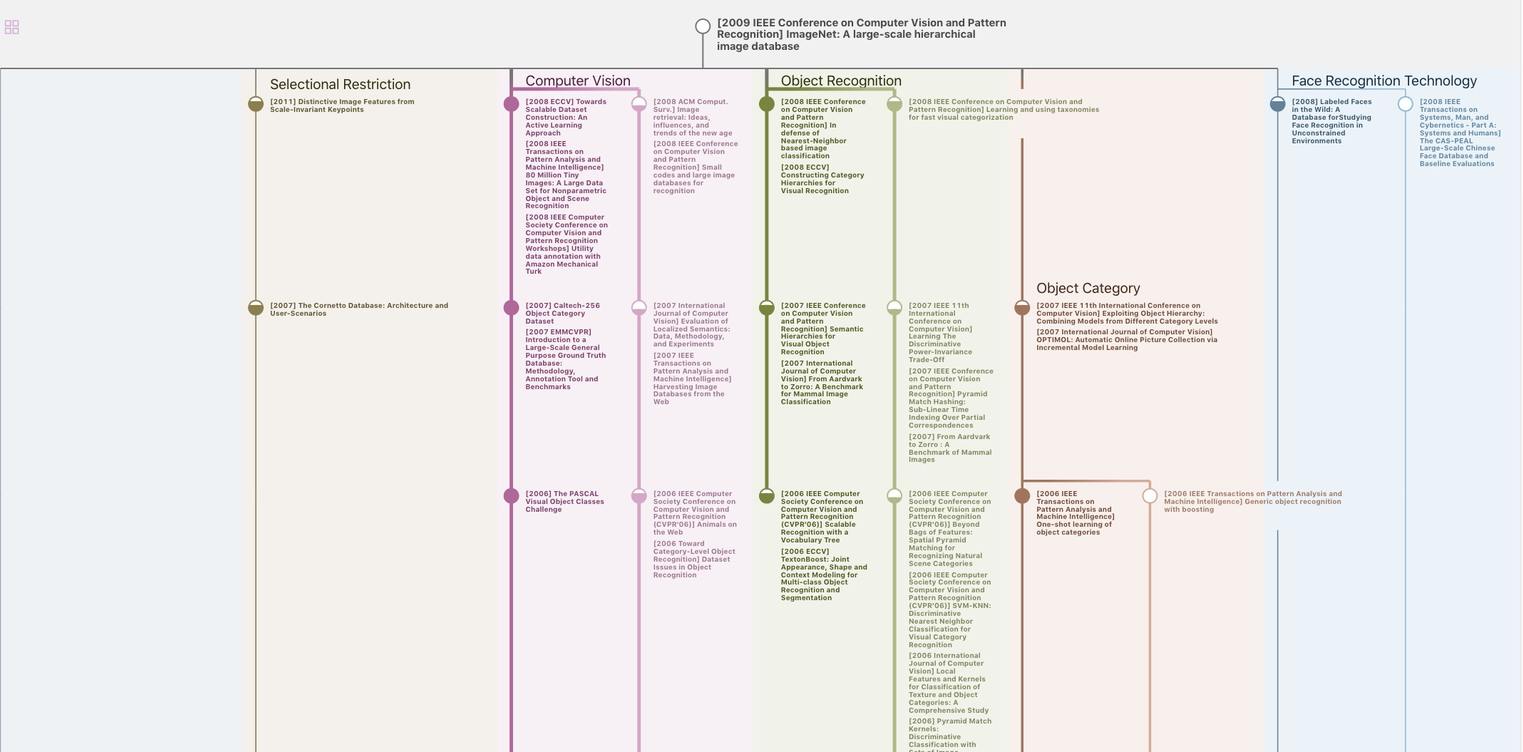
生成溯源树,研究论文发展脉络
Chat Paper
正在生成论文摘要