Robust prediction for CH4/CO2 competitive adsorption by genetic algorithm pruned neural network
GEOENERGY SCIENCE AND ENGINEERING(2024)
摘要
Capturing the competitive adsorption behavior of CH4/CO2 in shale reservoirs is essential for estimating the original gas-in-place and enhancing the shale gas recovery. This study leverages the neural network with sparsity, trained on a comprehensive dataset that includes crucial parameters such as clay, total organic carbon, specific surface area, pressure, and initial composition. Sparsity diverges from Dropout by selectively pruning unnecessary connections to enhance the model's robustness. Specifically, previous attempts suffer from overoptimistic performance due to unsuitable random dataset sampling. Hierarchical clustering and permutation importance algorithm indicate that petrophysical properties including clay, total organic carbon and specific surface area. By optimizing the sparsity through a genetic algorithm and connection pruning strategy, an accuracy improvement from 0.90 to 0.95 in terms of R-squared compared to conventional fully connected networks is witnessed. Additionally, the results show that removing up to 40% of the connections without compromising accuracy. Therefore, a heterogeneous configuration of activation functions within the neural network further amplifies its predictive capability. In addition, compared to the Dropout strategy, sparsity is more advantageous for generalizability in scenarios with limited datasets.
更多查看译文
关键词
CH4/CO2 competitive adsorption,Sparsely connected neural network,Robust prediction,Genetic algorithm
AI 理解论文
溯源树
样例
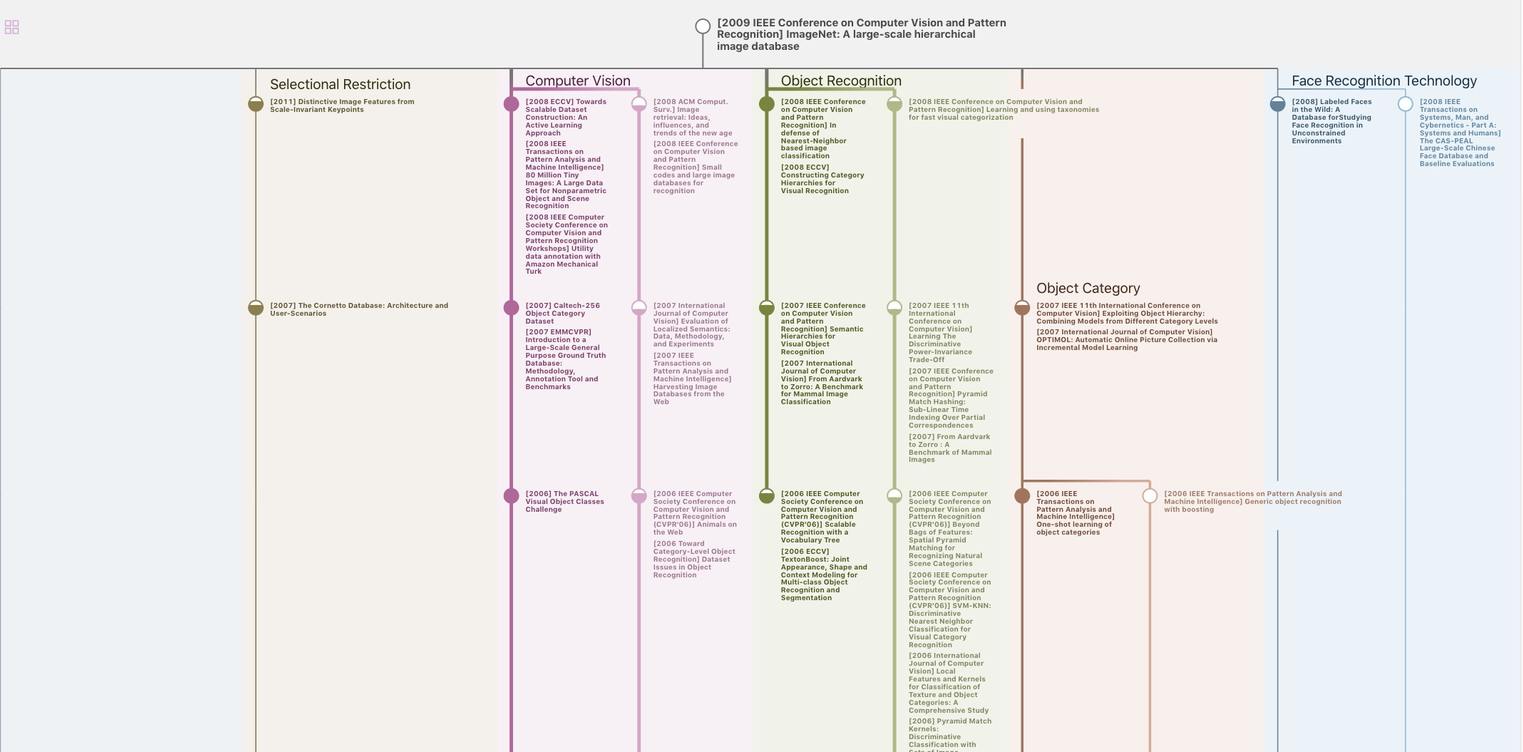
生成溯源树,研究论文发展脉络
Chat Paper
正在生成论文摘要