Machine learning based modeling for predicting the compressive strength of solid waste material-incorporated Magnesium Phosphate Cement
JOURNAL OF CLEANER PRODUCTION(2024)
摘要
In this study, a compressive strength prediction model for Magnesium Phosphate Cement containing solid waste materials (SW-MPC) was developed using machine learning (ML) techniques. Firstly, a database of SW-MPC compressive strength was established through the selection of solid waste materials based on their chemical compositions. Subsequently, various ML algorithms were employed to construct predictive models for SW-MPC compressive strength, and the predictive accuracy of these models was compared. Furthermore, Shapley Additive Explanations (SHAP) analysis was utilized to assess the impact of various parameters on SW-MPC compressive strength. The results revealed that the Extreme Gradient Boosting (XGB) model exhibited the highest predictive accuracy and generalization ability. Among numerous feature parameters, the curing age (Age) and phosphate type (Type_PO4) were identified as the most critical factors influencing SW-MPC compressive strength. SW-MPC compressive strength exhibited a positive correlation with the Si content (Con_Si) and Al content (Con_Al) in the solid waste materials, while it generally displayed a negative correlation with Fe content (Con_Fe). The optimal Magnesium-to-Phosphorus ratio (M/P) for SW-MPC preparation ranged from 4 to 6. Furthermore, the influence of the dosage of solid waste materials (Per_sw) on the compressive strength of SW-MPC was complex, with the optimal content being around 10% and 35%. This complexity arose from the significant impact of the interactive effects between the content of different elements in solid waste materials and Per_sw on the compressive strength of SW-MPC.
更多查看译文
关键词
Machine learning,Magnesium phosphate cement,Solid waste materials,Compressive strength prediction
AI 理解论文
溯源树
样例
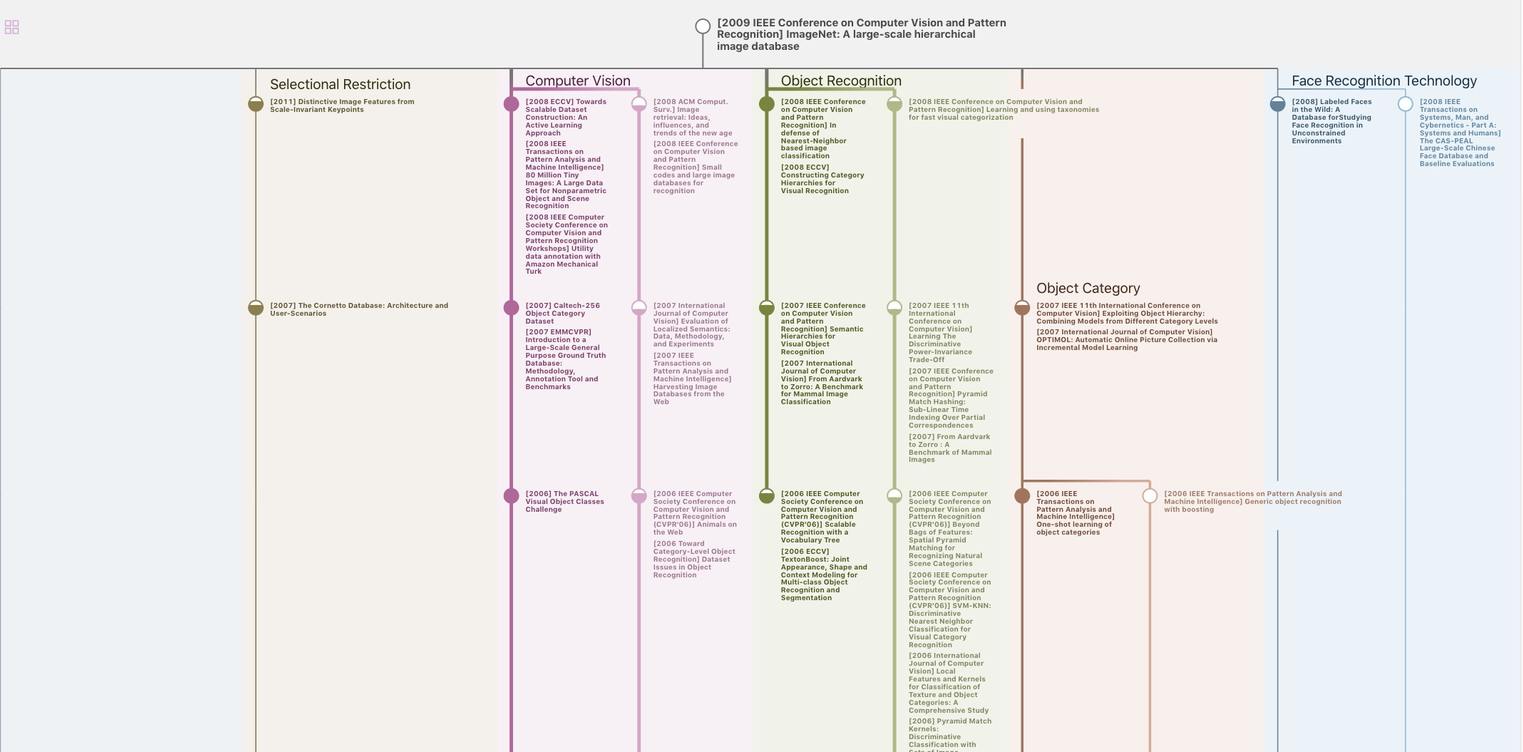
生成溯源树,研究论文发展脉络
Chat Paper
正在生成论文摘要