A multi-stage time series processing framework based on attention mechanism for early life prediction of lithium-ion batteries
JOURNAL OF ENERGY STORAGE(2024)
Abstract
Lithium -ion batteries play a vital role in powering portable electronic devices and electric vehicles. In order to shorten the research time, it is significant to accurately predict the life of lithium -ion batteries in the early cycle. However, due to the nonlinear degradation pattern with inconspicuous capacity variation in early cycles, predicting the life of the battery remains challenging when only the limited early cycle samples are available. In this paper, a multi -stage time series processing framework based on attention mechanism is proposed. To extract divergent features, category based intra-cycle sequence feature extraction network (CICSN) is designed for battery voltage, current and temperature (V/I/T) in the cycle, and inter -cycle global feature extraction network (ICGN) is proposed for discharge capacity across cycles. The cross -attention mechanism is adopted to fuse features, further improving the performance of prediction. A comprehensive evaluation of the proposed method is conducted through a lithium -ion battery dataset collected by MIT. The results show that the values of MAPE, MAE and RMSE decrease to 6.4%, 45 cycles and 67 cycles, which are better than existing research methods and demonstrate the effectiveness and validity of the proposed model.
MoreTranslated text
Key words
Lithium-ion battery,Cycle life early prediction,Attention mechanism,Stage feature extraction,Time series prediction
AI Read Science
Must-Reading Tree
Example
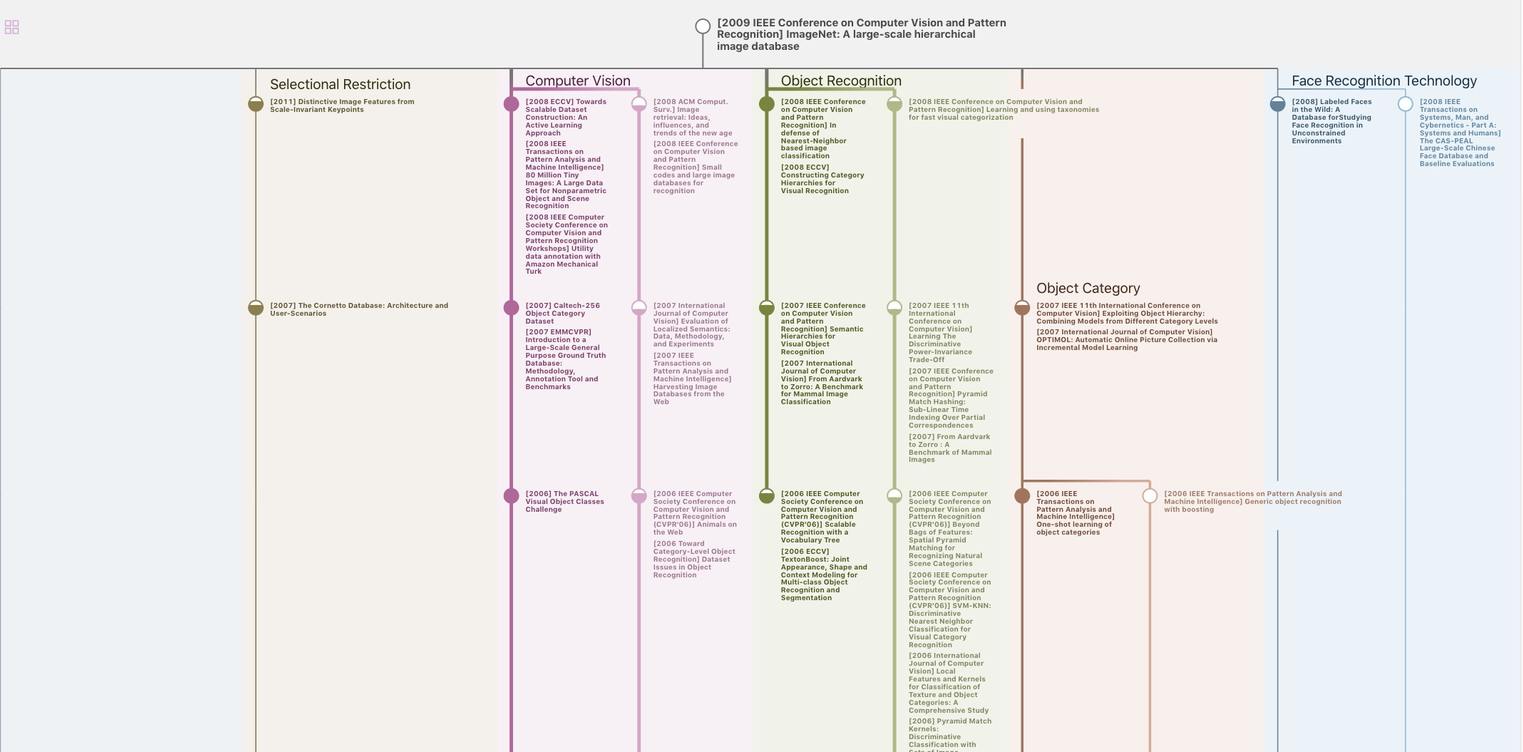
Generate MRT to find the research sequence of this paper
Chat Paper
Summary is being generated by the instructions you defined