Identification of the aging state of lithium-ion batteries via temporal convolution network and self-attention mechanism
JOURNAL OF ENERGY STORAGE(2024)
摘要
Deep learning methods have been widely used for battery aging state estimation with either manual or automatic features, while the contribution of multi -source features is rarely considered. To solve this problem, a hybrid method is proposed to combine the manual and automatic features based on a temporal convolution network (TCN) and a self -attention mechanism (SA). Specifically, the local voltage, capacity, and incremental capacity are manually extracted as battery aging features. Then, for extracting automatic features, TCN employs dilated convolution to capture the capacity regeneration phenomenon during battery degradation. Considering the contribution of multi -source features, we use SA to fuse the obtained manual and automatic features. Finally, the available capacity and remaining useful life of the battery are predicted using a fully connected neural network on one dataset from our lab, the Oxford University dataset, and the MIT University dataset. The experimental results show that the proposed method exhibits a high accuracy of aging state identification.
更多查看译文
关键词
Lithium -ion batteries,Capacity,Remaining useful life,Temporal convolution network,Self -attention
AI 理解论文
溯源树
样例
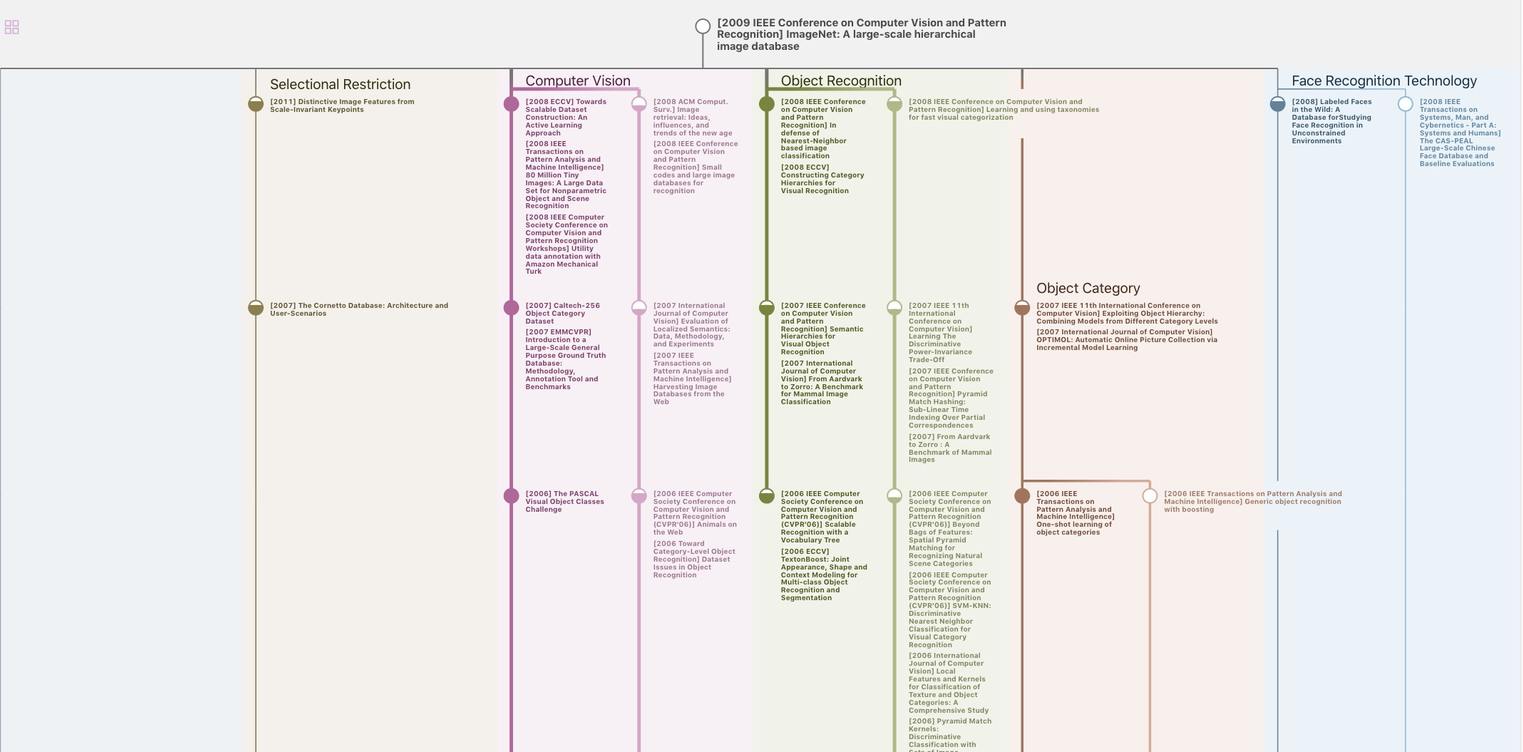
生成溯源树,研究论文发展脉络
Chat Paper
正在生成论文摘要