Multi-View SpatialCTemporal Graph Convolutional Network for Traffic Prediction
IEEE TRANSACTIONS ON INTELLIGENT TRANSPORTATION SYSTEMS(2024)
摘要
Multi-step traffic speed prediction is a challenging issue due to the multiple spatial-temporal dependencies among roads. Some spatial dependencies, especially those formed by different traffic modes, are not fully exploited, and how to simultaneously consider spatial and temporal dependencies and effectively integrate them within a single prediction framework needs further exploration. To tackle the above issues, we propose a multi-view spatial-temporal graph convolutional framework MVSTG, which adequately exploits the multi-view spatial-temporal dependencies and their interactions to improve the accuracy of traffic prediction. Multi-view temporal learning captures the multiple temporal trends by temporal convolution from multi-granularity historical data, and multi-view spatial learning handles the multiple spatial correlations by graph convolution from multiple graphs. In addition, view-wise attention-based fusion is proposed to adaptively identify the importance of each upstream view, fuse the multi-view information, and generate integrated results for downstream views. The experiments on two real-world urban traffic datasets demonstrate that the multi-view data and the proposed model framework enhance performance on the accuracy of speed prediction, especially in mid-term and long-term prediction.
更多查看译文
关键词
Attention mechanism,multi-view learning,spatial-temporal graph convolutional network,traffic prediction
AI 理解论文
溯源树
样例
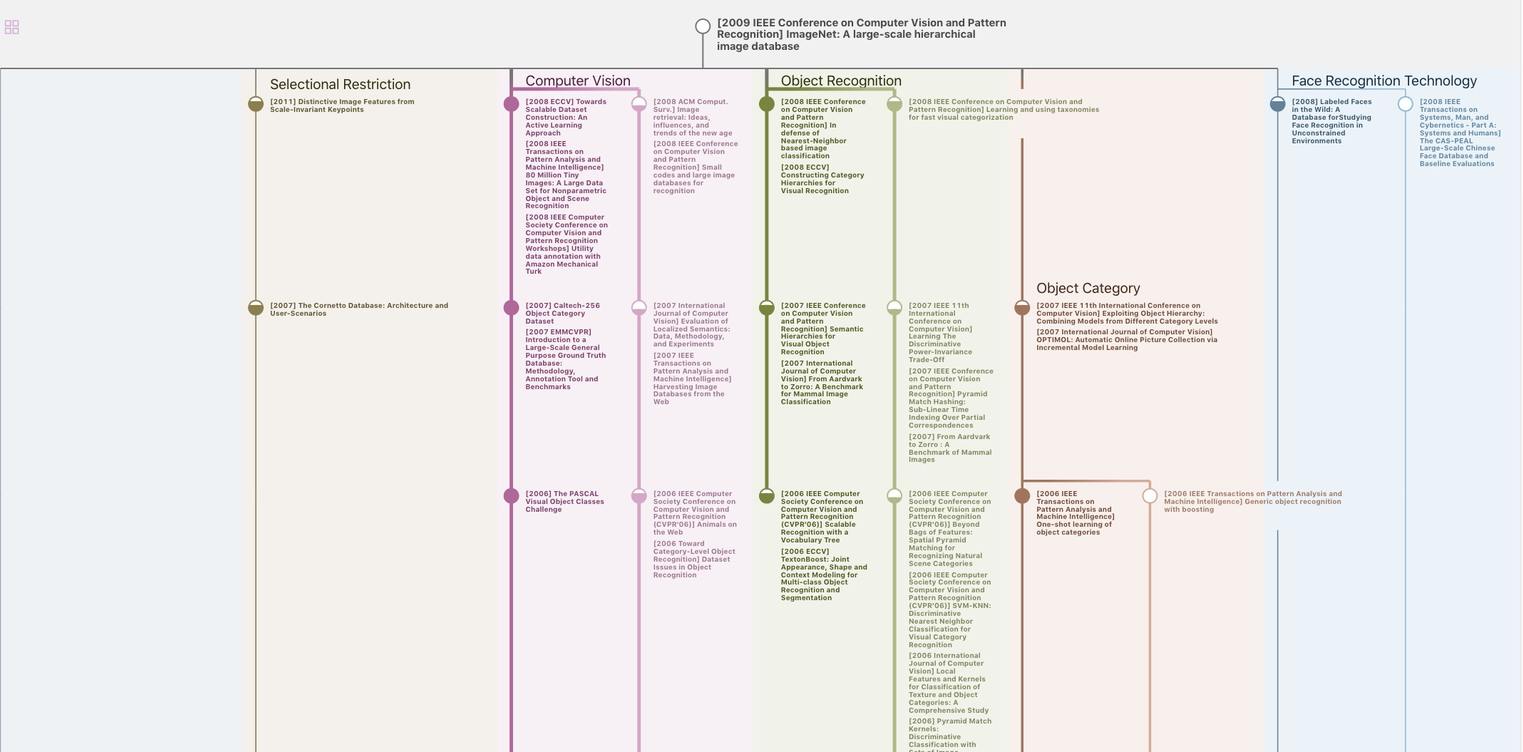
生成溯源树,研究论文发展脉络
Chat Paper
正在生成论文摘要