FedTweet: Two-fold Knowledge Distillation for non-IID Federated Learning
COMPUTERS & ELECTRICAL ENGINEERING(2024)
摘要
Federated Learning (FL) is a distributed learning approach that allows each client to retain its original data locally and share only the parameters of the local updates with the server. While FL can mitigate the problem of "data islands", the training process involving nonindependent and identically distributed (non-IID) data still faces the formidable challenge of model performance degradation due to "client drift"in practical applications. To address this challenge, in this paper, we design a novel approach termed "Two-fold Knowledge Distillation for non-IID Federated Learning"(FedTweet), meticulously designed for the personalized training of both local and global models within various heterogeneous data contexts. Specifically, the server employs global pseudo -data for fine-tuning the initial aggregated model through knowledge distillation and adopts dynamic aggregation weights for local generators based on model similarity to ensure diversity in global pseudo -data. Clients freeze the received global model as a teacher model and conduct adversarial training between the local model and local generator, thus preserving the personalized information in the local updates while correcting their directions. FedTweet enables both global and local models to serve as teacher models for each other, ensuring bidirectional guarantees for personalization and generalization. Finally, extensive experiments conducted on benchmark datasets demonstrate that FedTweet outperforms several previous FL methods on heterogeneous datasets.
更多查看译文
关键词
Federated learning,Non-IID data,Knowledge distillation,Adversarial training
AI 理解论文
溯源树
样例
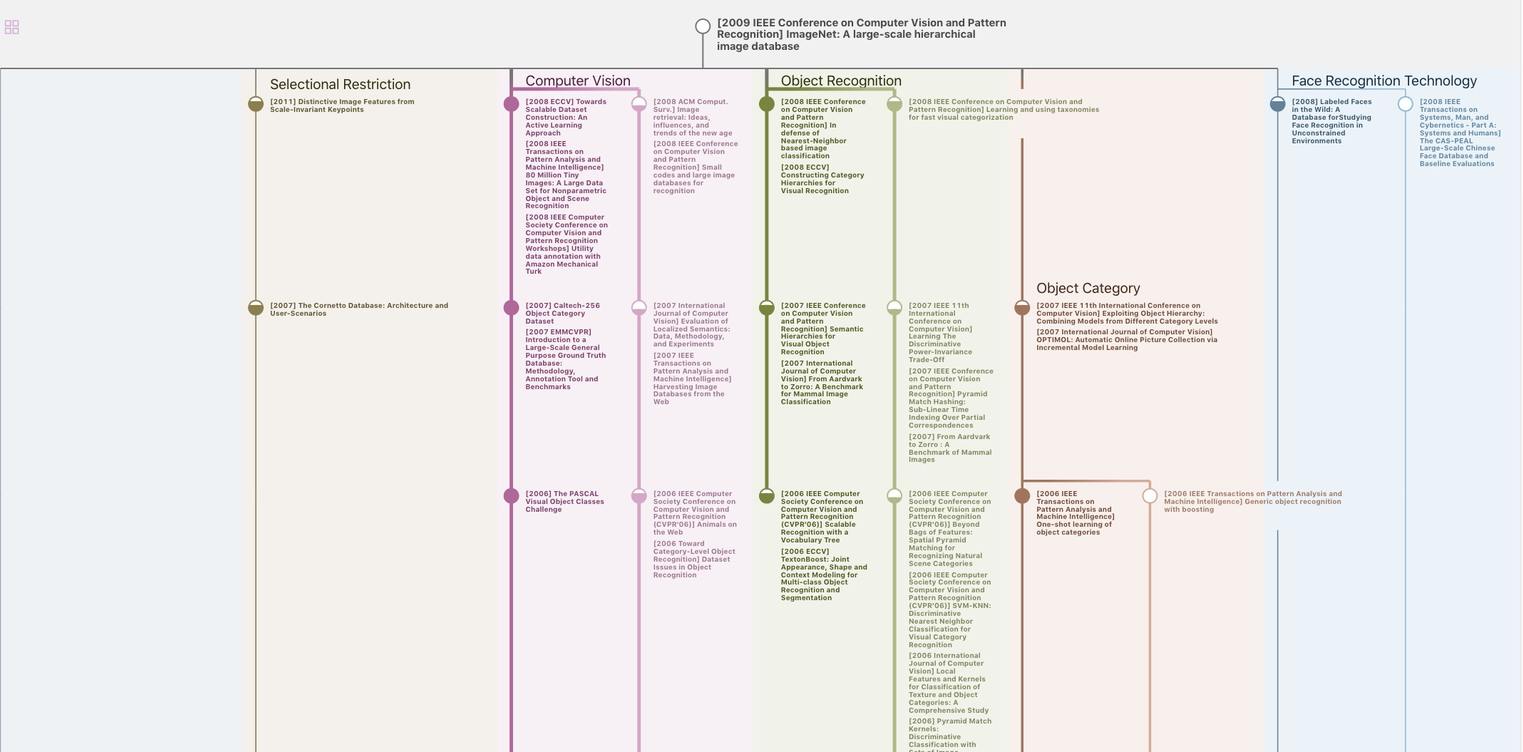
生成溯源树,研究论文发展脉络
Chat Paper
正在生成论文摘要