Fast reconstruction of boiler numerical physical field based on proper orthogonal decomposition and conditional deep convolutional generative adversarial networks
INTERNATIONAL JOURNAL OF GREEN ENERGY(2024)
摘要
Obtaining real-time physical field data inside the boiler furnace is crucial for combustion diagnostics and optimization. In this work, we propose a fast reconstruction technique for the physical fields in a tangentially-fired coal boiler. The method combines the Proper Orthogonal Decomposition (POD) and Conditional Deep Convolutional Generative Adversarial Networks (cDCGAN) based on the boiler physical field obtained by computational fluid dynamics (CFD). Firstly, CFD simulations are performed using data from the boiler Distributed Control System (DCS). After verifying the CFD results, we expanded the simulation to 105 operational condition cases sample sets. Then, the physical fields of the sample set undergo POD to determine the appropriate number of POD bases, thereby ensuring the accuracy of the reconstruction. Finally, the cDCGAN method is employed to establish the correlation between the POD bases and the operational conditions of the boiler. Results show that the POD-cDCGAN method achieves rapid and accurate reconstruction of temperature and velocity fields, with mean relative error (MRE) below 1.5% and root relative squared error (RRSE) below 5.7%. The reconstruction time is reduced to 10.57s, significantly faster than direct CFD methods. The POD-cDCGAN method offers an efficient approach for online operational diagnosis and digital twin construction of boiler equipment.
更多查看译文
关键词
Boiler physical field,CFD,proper orthogonal decomposition(POD),cDCGAN,reduced order modeling
AI 理解论文
溯源树
样例
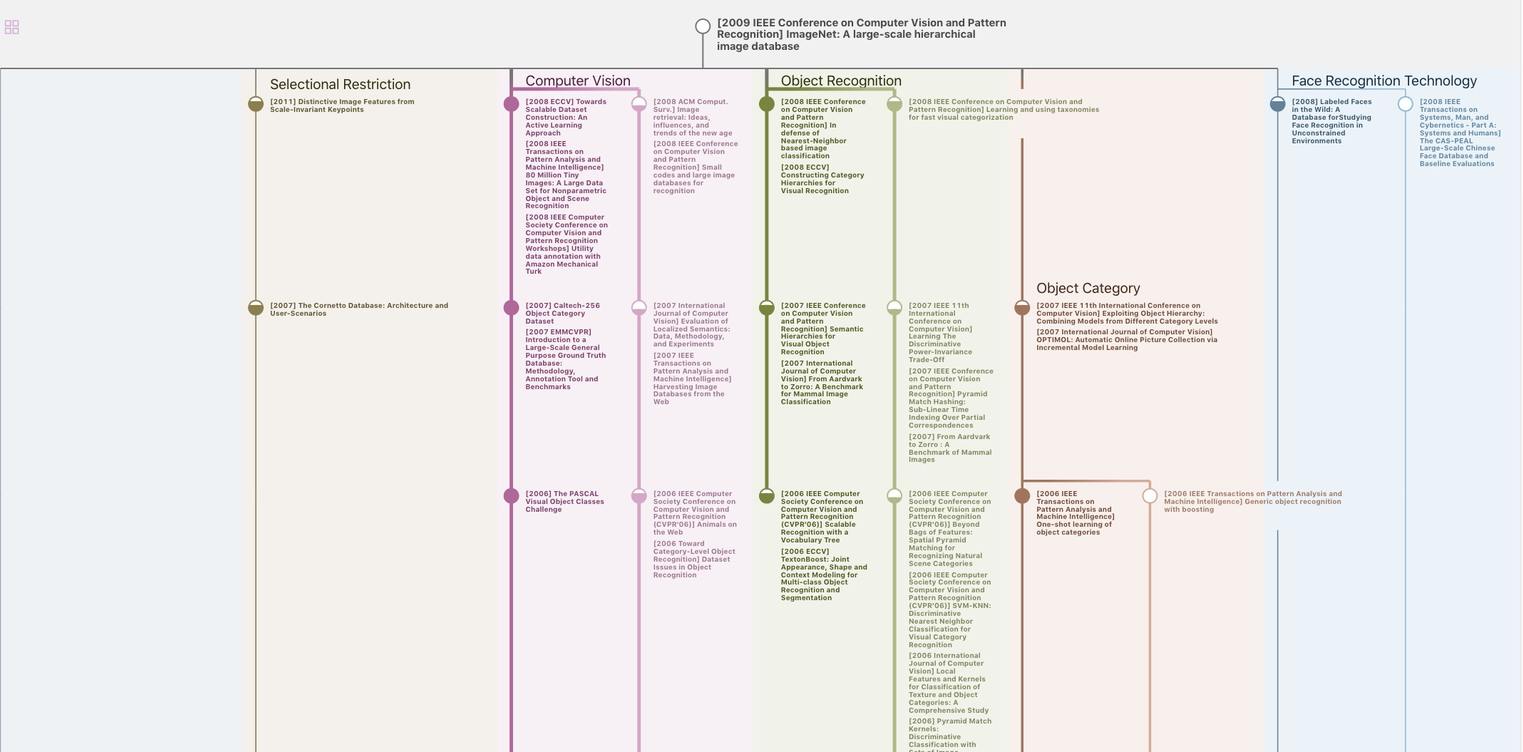
生成溯源树,研究论文发展脉络
Chat Paper
正在生成论文摘要