Applying enhanced active learning to predict formation energy
COMPUTATIONAL MATERIALS SCIENCE(2024)
摘要
The challenge of accurately predicting the formation energies of complex crystal structure compounds is central to our understanding of the stability of phases and inherent microstructures in materials. To address this issue, we introduced a meticulously curated multi -scale feature set that considered the inherent properties and crystal structure of materials. By applying our novel feature set, we achieved substantial accuracy enhancements across several datasets. Specifically, the Mean Absolute Error (MAE) on open -source dataset of 9974 samples decreased from 1254 to 631 J/(mol center dot atom). On our proprietary Cr-Fe-Co-Ni magnetic dataset, MAE was further reduced to just 244 J/(mol center dot atom). Our research introduced an enhanced active learning workflow, using the enhanced -query -by -committee (EQBC) strategy, which accurately and efficiently predicted formation energies while reducing the computational demands of Density Functional Theory (DFT) calculations. In our study, we incorporated the Mean Absolute Percentage Error Estimation (MAPEE) to illustrate the error distribution among unidentified samples. This approach significantly bolstered the interpretability of the results produced by the machine learning (ML) models in question. Notably, after the fifth iteration of enhanced active learning, our model reached an anticipated accuracy of 500 J/(mol center dot atom) on the test set. Our research culminated in the application of these results to construct a Cr-Fe-Co-Ni multi -component thermodynamic database (TDB), leading to a strong alignment between the computed and experimental occupancy data for the Cr-Fe-Co system. The proposed framework significantly reduced the computational demands of DFT while maintaining accurate predictions for formation energy.
更多查看译文
关键词
Formation energy,Active learning,Density functional theory,Mean absolute percentage error estimation,Machine learning,Thermodynamic database,Site occupancy
AI 理解论文
溯源树
样例
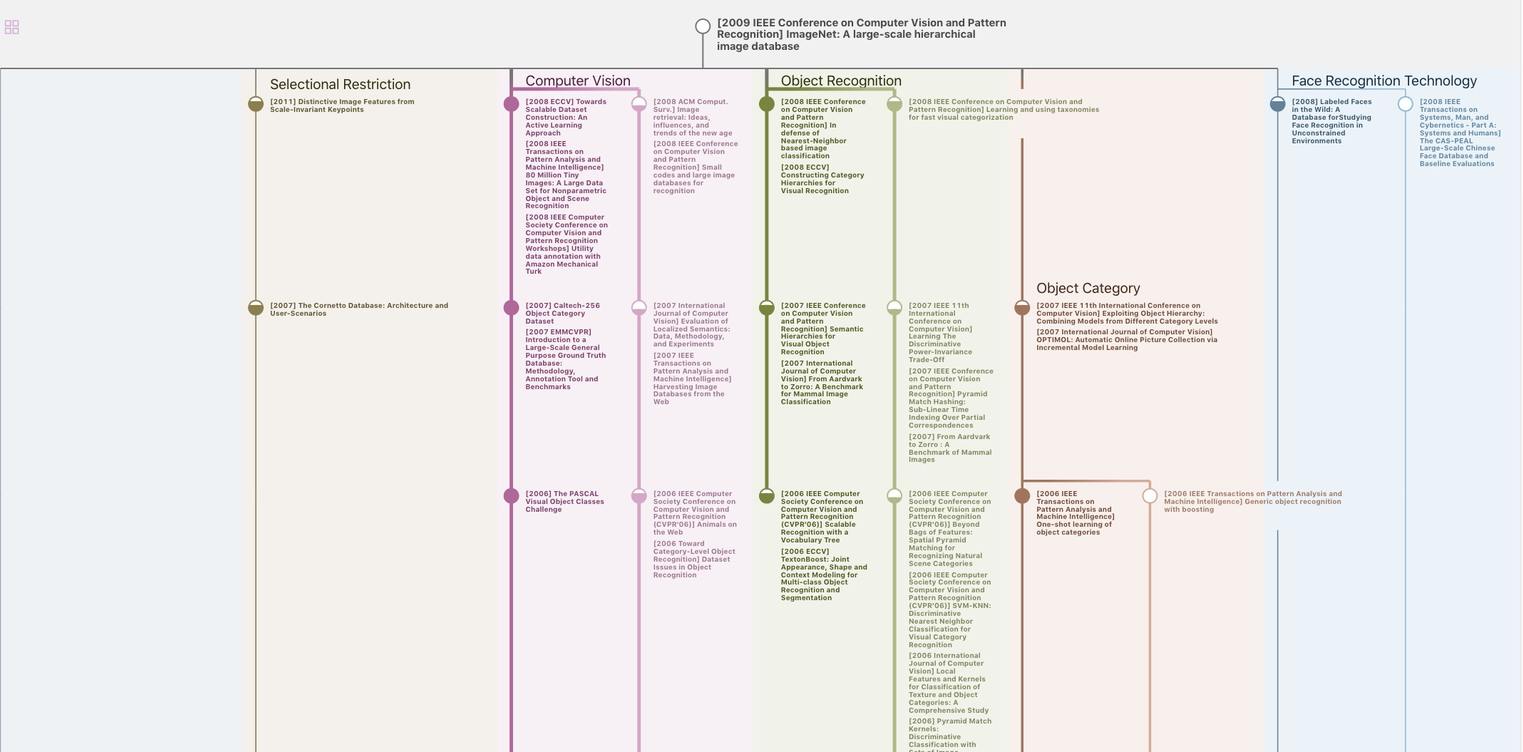
生成溯源树,研究论文发展脉络
Chat Paper
正在生成论文摘要