Distributed proportional likelihood ratio model with application to data integration across clinical sites
ANNALS OF APPLIED STATISTICS(2024)
摘要
Real -world evidence synthesis through integration of data from distributed research networks has gained increasing attention in recent years. Due to privacy concerns and restrictions of sharing patient -level data, distributed algorithms that do not require sharing patient level information are in great need for facilitating multisite collaborations. On the other hand, data collected at multiple sites often come from diverse populations, and there exists a substantial amount of heterogeneity across sites in patient characteristics. Most of the existing distributed algorithms have ignored such betweensite heterogeneity. In this paper we aim to fill this methodological gap by proposing a general distributed algorithm. We develop our distributed algorithm based on a general semiparametric model, namely, the proportional likelihood ratio model (Biometrika 99 (2012) 211-222), which is a semiparametric extension of generalized linear model. We devise the proportional likelihood ratio model with site -specific baseline function, to account for between -site heterogeneity, and shared regression parameters to borrow information across sites. Under this flexible formulation, our distributed algorithm is designed to be privacy -preserving and communication -efficient (i.e., only one round of communication across sites is needed). We validate our method via simulation studies and demonstrate the utility of our method via a multisite study of pediatric avoidable hospitalization based on electronic health record data from a total of 354,672 patients across 26 different clinical sites within the Children's Hospital of Philadelphia health system.
更多查看译文
关键词
Distributed research network,heterogeneity-aware distributed algorithms,noniterative distributed algorithm,privacy-preserving,real-world evidence
AI 理解论文
溯源树
样例
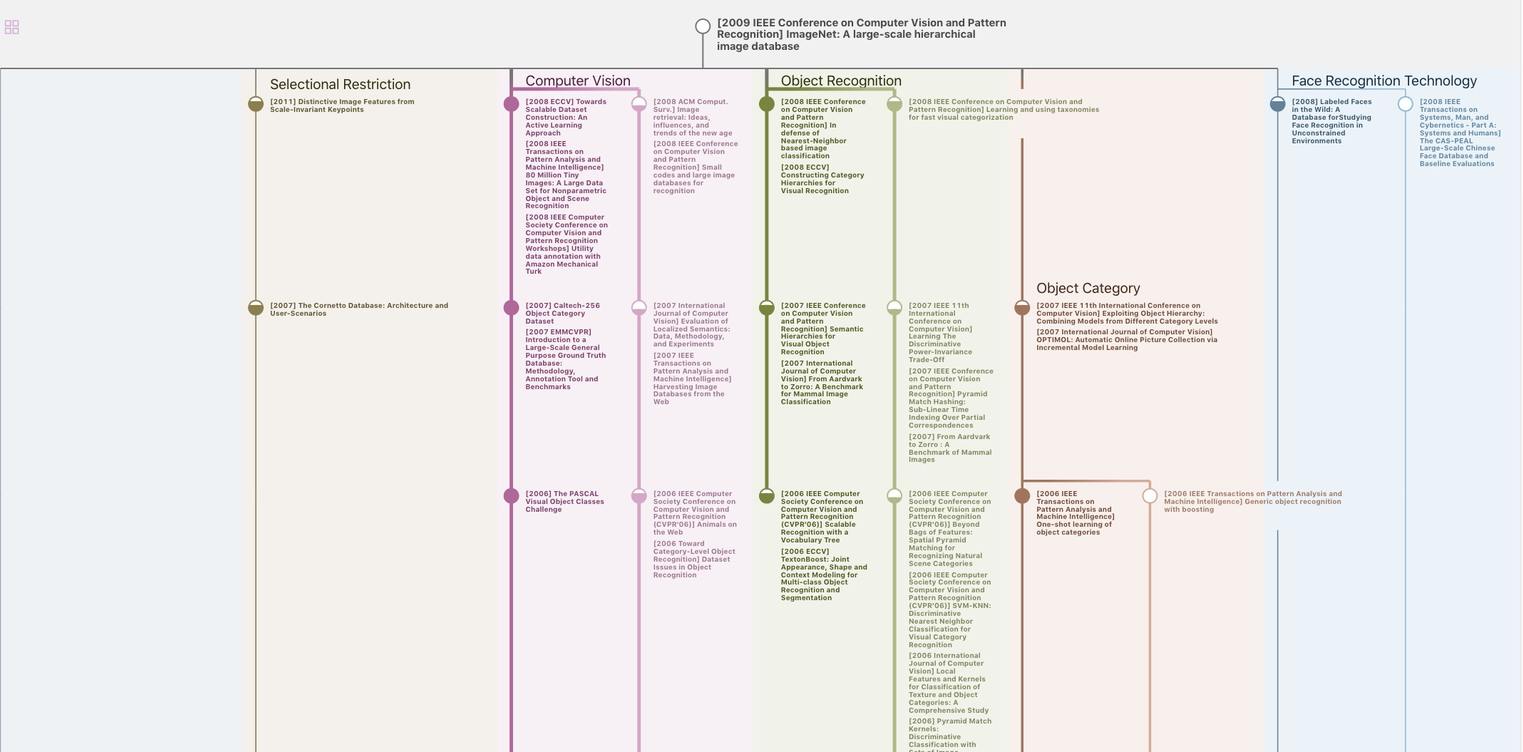
生成溯源树,研究论文发展脉络
Chat Paper
正在生成论文摘要