Comparative Performance Assessment of Physical-Based and Data-Driven Machine-Learning Models for Simulating Streamflow: A Case Study in Three Catchments across the US
JOURNAL OF HYDROLOGIC ENGINEERING(2024)
摘要
Recent developments in computational techniques and data-driven machine-learning models (MLMs) have shown great potential in capturing the rainfall-runoff relationship. However, whether MLMs outperform the classical physical-based models (PBMs) in streamflow simulation is still controversial. In this study, we chose three representative catchments across the continental United States for a comparative analysis of these two model categories, including two PBMs, i.e., a conceptual hydrological model (EXP-HYDRO) and a classical semidistributed hydrological model (SWAT), and three MLMs, i.e., support vector regression (SVR), backpropagation artificial neural networks (BP-ANN), and a deep learning model, termed long short-term memory (LSTM). Results indicate that the bias of SVR and BP-ANN models is greater than PBMs under the baseline input scenario, while LSTM outperforms other models for the rainfall-runoff relationship. For delayed input scenarios, MLMs have great potential and perform satisfactorily. In addition, MLMs show better performance in the high-flow regime, while PBMs perform better in the low-flow regime, implying that both PBMs and MLMs have their own merits and should be jointly employed holistically to analyze the streamflow. Our comparison analysis demonstrates that the performance of MLMs and PBMs is variable under different seasonal, climatic, and topographic conditions, and conclude that MLMs can better capture the rainfall-runoff relationship than PBMs, when the coefficient of variation (COV) is large.
更多查看译文
关键词
Streamflow simulation,Hydrological models,Data-driven machine-learning model (MLM),Physical-based hydrological model
AI 理解论文
溯源树
样例
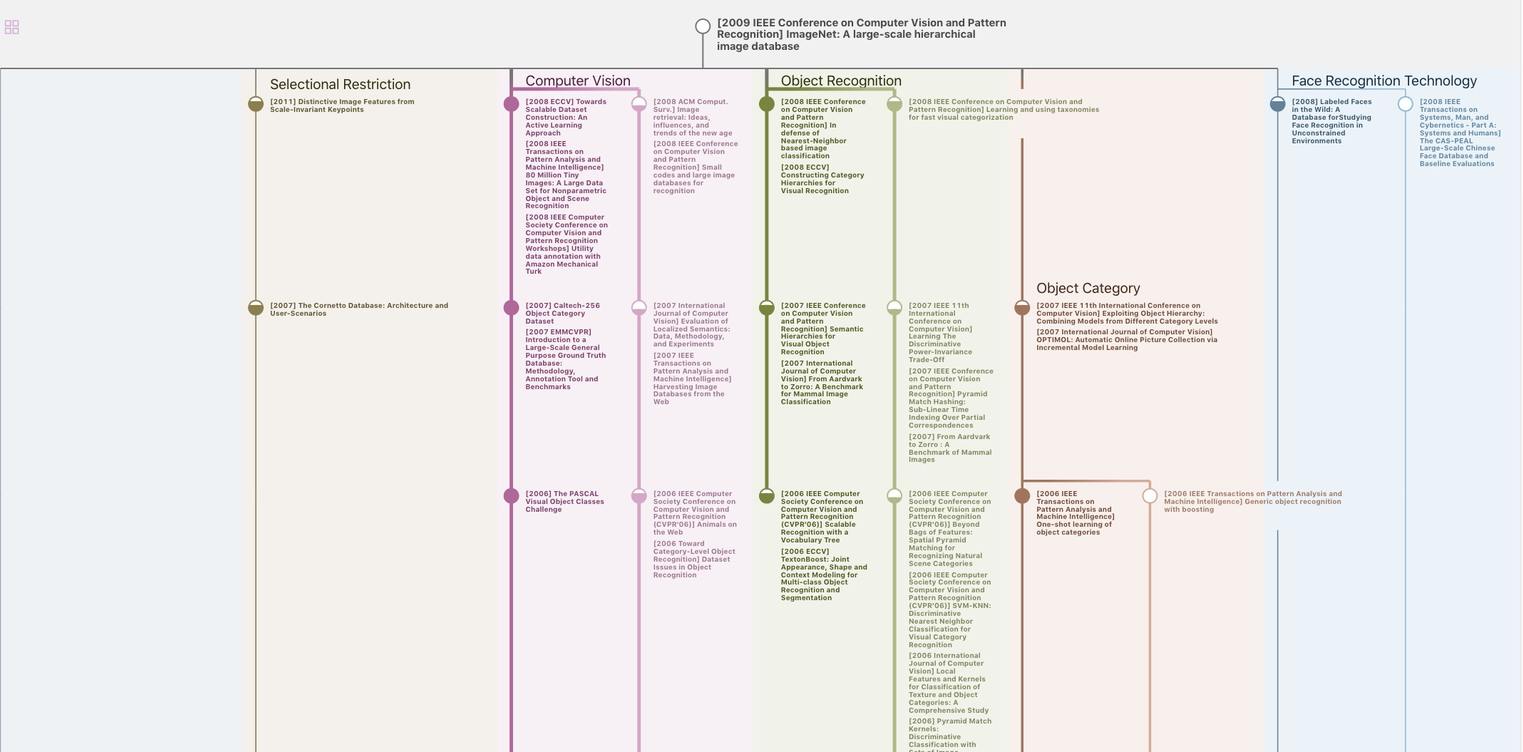
生成溯源树,研究论文发展脉络
Chat Paper
正在生成论文摘要