Data-driven energy efficient speed planning for battery electric industrial vehicles: Forklift as a case study
JOURNAL OF CLEANER PRODUCTION(2024)
Abstract
Battery electric industrial vehicles (BEIVs) offer significant environmental protection and decarbonization potential for rapidly growing logistics and transportation sectors in China. However, the limited driving range remains a major obstacle to the widespread adoption of BEIVs. Eco-driving is an effective solution that can improve energy efficiency and extend the driving range of vehicles by controlling driving behavior and speed. Therefore, this paper introduces an energy efficient speed planning method for BEIVs, in which data-driven models are developed to estimate energy consumption. Real-world driving data collected from a BEIV are used to model the energy consumption by curve fitting, random forest, and LSTM. The speed planning process is considered a Markov Decision Process, which is solved using Dynamic Programming and Q-learning, respectively. The case study of a battery electric forklift shows that speed planning can reduce energy consumption by 13%-27% compared to time-optimal speed, while only increasing the driving time by 10%-20%.
MoreTranslated text
Key words
Electric vehicle,Forklift,Speed planning,Energy consumption,Dynamic Programming,Q-learning,Industrial vehicles
AI Read Science
Must-Reading Tree
Example
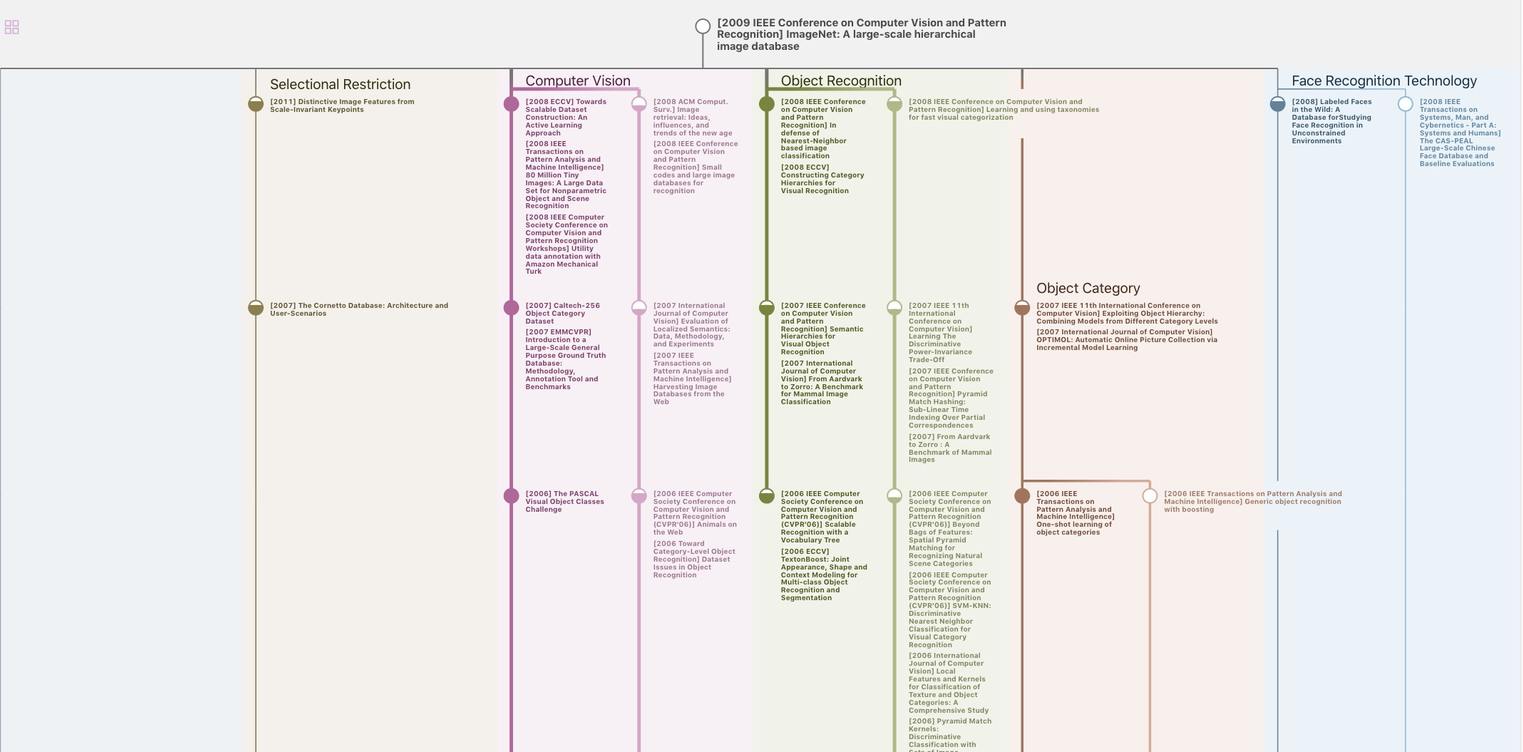
Generate MRT to find the research sequence of this paper
Chat Paper
Summary is being generated by the instructions you defined