A novel data augmentation method for steering mechanism fault diagnosis based on variational autoencoding generative adversarial networks with self-attention
MEASUREMENT SCIENCE AND TECHNOLOGY(2024)
摘要
Learning the original data distribution and generating new samples has proven to be an effective approach in addressing the issue of data imbalance. This paper combines the strengths of generative adversarial networks and variational autoencoder, proposing a novel data augmentation method named variational autoencoding generative adversarial networks with self-attention. Specifically, an encoding-decoding process is introduced during the generative adversarial process to provide distribution information for the generator's sampling space, thereby accelerating the model's convergence speed and simultaneously improving the quality of generated samples. Additionally, a self-attention module is incorporated into the discriminator to capture global information from the input data, guiding the generator. During the training process, overlapping sampling and feature-layer matching are employed. Comparative experiments with other advanced algorithms on both public and engineering datasets with multiple imbalanced cases demonstrate that the proposed method can generate high-quality samples, effectively enhance original imbalanced data, and exhibit strong generalization and robustness.
更多查看译文
关键词
rolling bearing,data augmentation,variational autoencoding generative adversarial networks,self-attention module,overlapping sampling
AI 理解论文
溯源树
样例
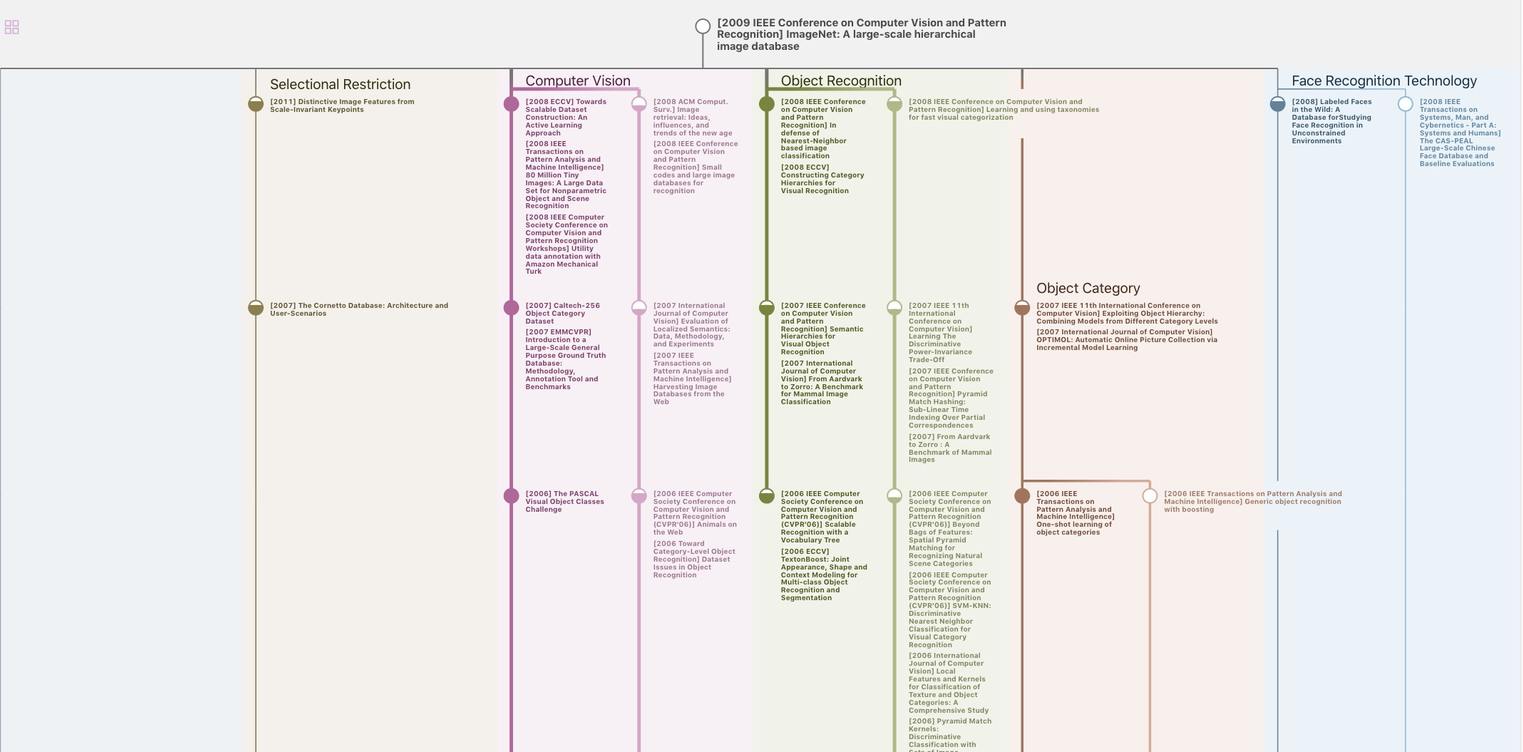
生成溯源树,研究论文发展脉络
Chat Paper
正在生成论文摘要