Multiattribute E-CARGO Task Assignment Model Based on Adaptive Heterogeneous Residual Networks
IEEE TRANSACTIONS ON COMPUTATIONAL SOCIAL SYSTEMS(2024)
摘要
Mobile crowd sensing (MCS) is an emerging approach to collect data using smart devices. In MCS, task assignment is described as assigning existing tasks to known workers outside the constraints of task demand attributes and worker attributes, and maximizing the profit of the platform. However, workers and tasks often exist in different environments and heterogeneous features such as workers with attributes are not considered, leading to nondeterministic polynomial (NP)-hard task assignment problems. To optimize such problems, this article proposes a multiattribute environments-classes, agents, roles, groups, and objects (E-CARGO) task assignment model based on adaptive heterogeneous residual networks (AHRNets). The AHRNet is integrated into deep reinforcement learning (DRL) to optimize the NP-hard problem, dynamically adjust task assignment decisions and learn the relationship between workers with different attributes and task requirements. Multiattribute E-CARGO uses group task assignment policy to obtain the ideal worker-task assignment relationship. Compared with traditional heuristic algorithms for solving NP-hard, this method has the flexibility and applicability of adaptive networks, enabling the solver to interact with and adapt to new environments and generalize its experience to different situations. Under various experimental conditions, a large number of numerical results show that this method can achieve better results than the reference scheme.
更多查看译文
关键词
Task analysis,Data models,Resource management,Adaptation models,Sensors,Residual neural networks,Collaboration,Adaptive heterogeneous residuals network (AHRNet),group task assignment (GTA),multiattribute environments-classes,agents,roles,groups,and objects (E-CARGO) model,task assignment
AI 理解论文
溯源树
样例
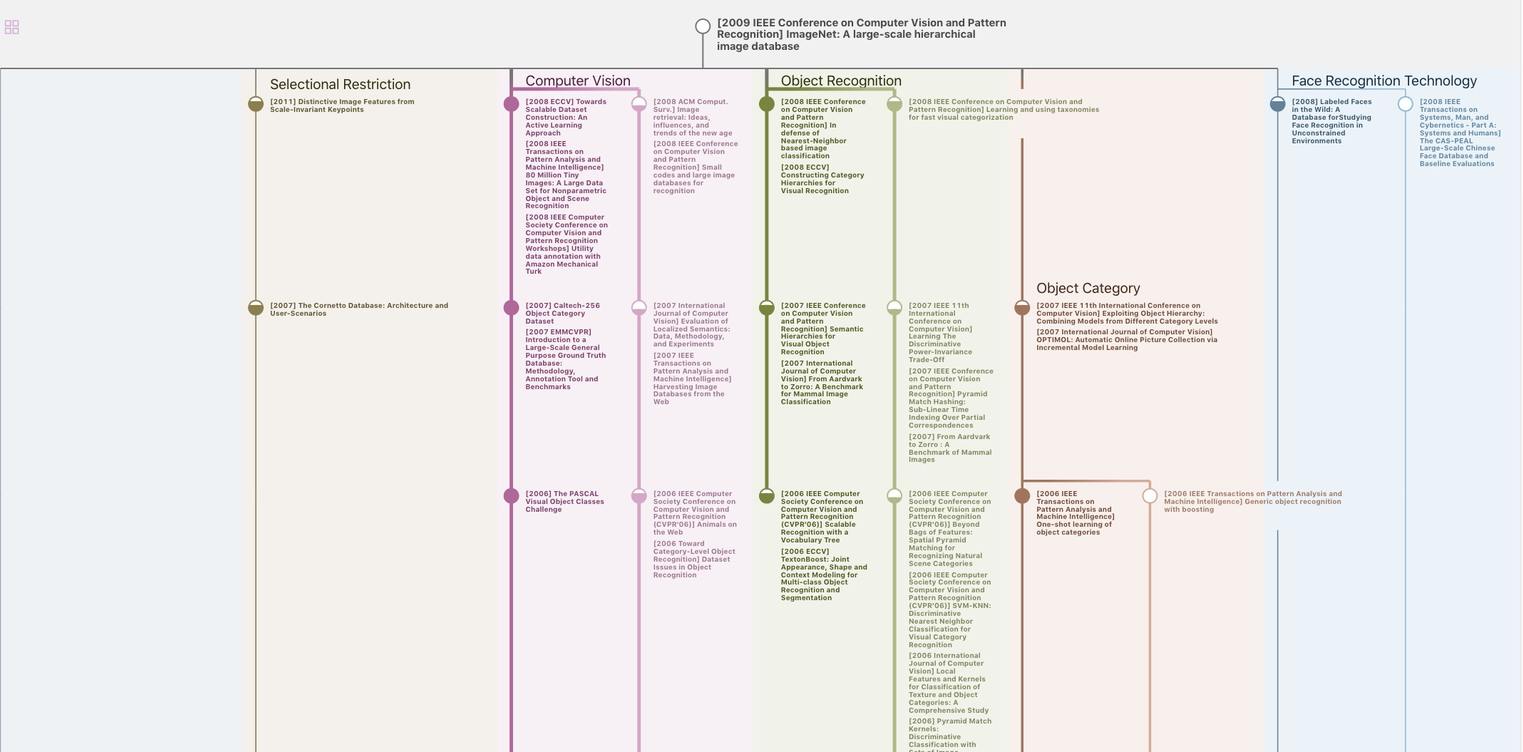
生成溯源树,研究论文发展脉络
Chat Paper
正在生成论文摘要