Machine-learning topology optimization with stochastic gradient descent optimizer for heat conduction problems
INTERNATIONAL JOURNAL OF HEAT AND MASS TRANSFER(2024)
Abstract
Recently, efforts have been paid to integrate machine learning (ML) into topology optimization (TO) mainly for structural problems. Here, oriented to the heat conduction problems with concentrated source originated from electronics cooling, we study the utility of ML to accelerate the density -based TO method. A TO framework with online training for the ML -based sensitivity predictor is built to obtain the high-fidelity optimal solutions from the low -fidelity simulations. Two schemes to suppress the negative effect of the sensitivities' prediction errors are proposed in this case: a novel optimizer is developed through combing the optimality criteria and stochastic gradient descent methods; the convergence analysis suggests that gradually increasing the dataset size for training during the iteration process is also beneficial. The acceleration of TO through integrating the ML is significant (total computation time reduced by up to 70 %), with the minor optimization degradation (maximum deviations of objective function lower than 4 %). Using this ML -assisted TO method, the influence of heat source size on the optimal topologies is investigated. It demonstrates the heat spreading effect drives the filler from the tree -like distributions to the direct highly -thermal -conductive bridge from the hot spot to heat sink, which could provide helpful guidance for practical electronics cooling designs.
MoreTranslated text
Key words
Topology optimization,Machine learning,Stochastic gradient descent,Heat conduction,Heat spreading
AI Read Science
Must-Reading Tree
Example
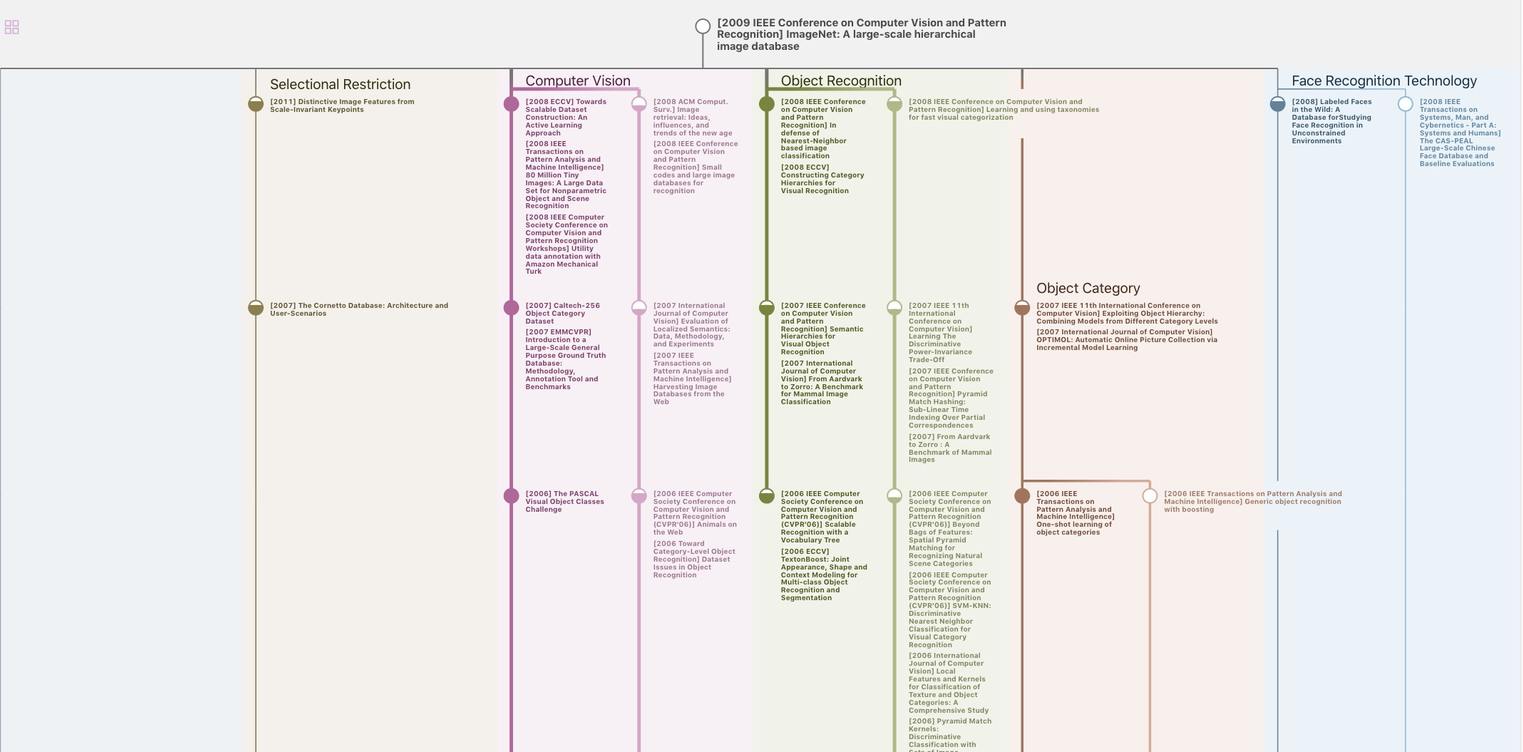
Generate MRT to find the research sequence of this paper
Chat Paper
Summary is being generated by the instructions you defined