Depth_ASPP_UNet plus +: a novel 2D ESPI phase unwrapping based on convolutional neural network
MEASUREMENT SCIENCE AND TECHNOLOGY(2024)
摘要
Phase unwrapping is a key step in optical metrology and physical optics to obtain accurate phase distributions. In practice, phase images obtained from electronic speckle pattern interferometry (ESPI) exhibit diverse and complex morphology, with significant shape variations and non-uniform densities among different individuals. This takes challenges for accurately extracting phase information and unwrapping the phase. With the progress of deep learning technology in optical image processing, real-time performance and accuracy have become concerned issues. In this paper, an ESPI phase unwrapping method based on convolutional neural network UNet++ is proposed. The proposed network combines the depthwise separable convolution (DSC), atrous spatial pyramid pooling (ASPP), defined as Depth_ASPP_UNet++. In this model, the use of DSC improves network computational efficiency and provides better feature representation capability. In addition, ASPP is introduced to pay more attention to the phase information of the phase image, and then obtain better phase unwrapping results. The experimental results show that our proposed method can obtain excellent results, especially with various of variable density, different noise levels, and different speckle sizes.
更多查看译文
关键词
phase unwrapping,deep learning,convolutional network,electronic speckle pattern interferometry,optical metrology
AI 理解论文
溯源树
样例
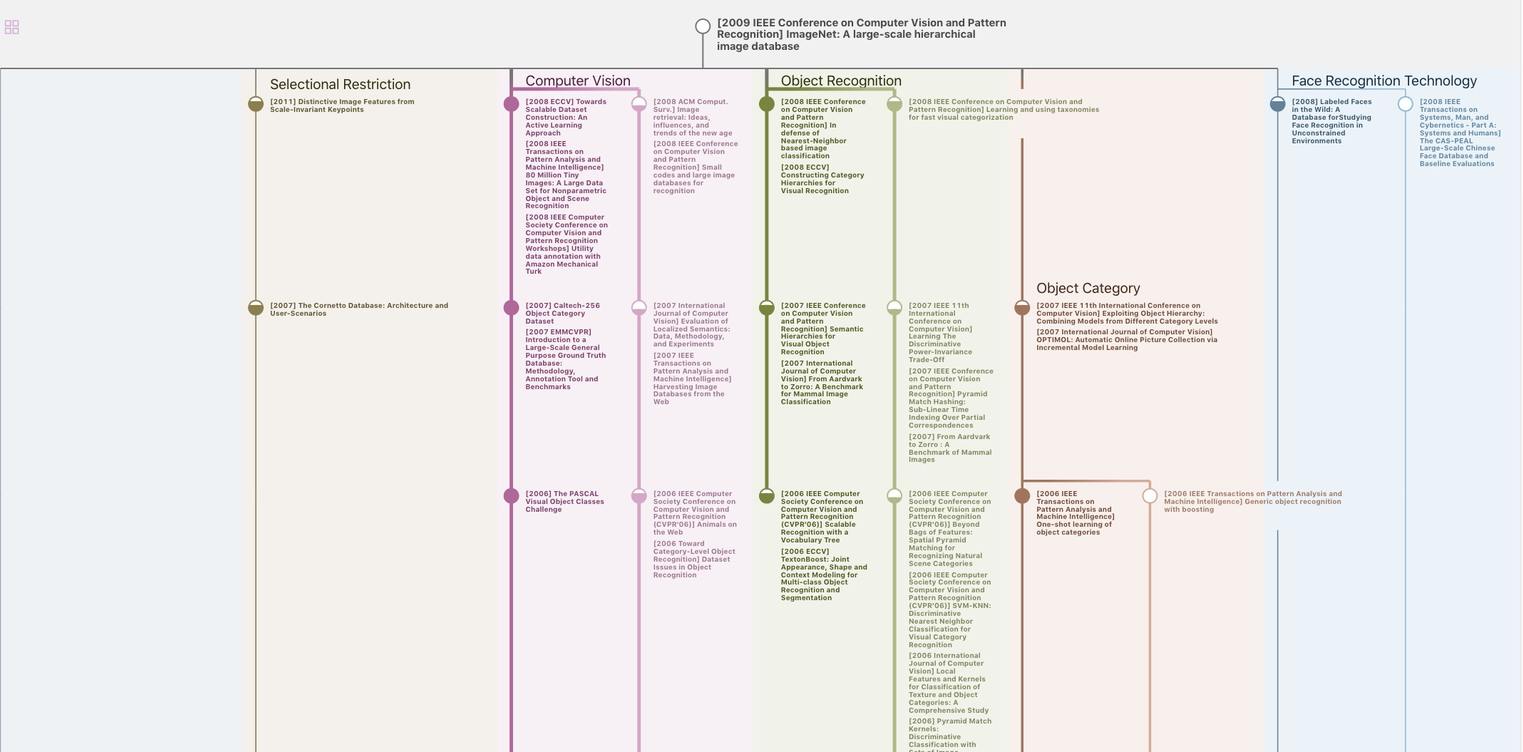
生成溯源树,研究论文发展脉络
Chat Paper
正在生成论文摘要