State of health prediction of lithium-ion batteries using particle swarm optimization with Levy flight and generalized opposition-based learning
JOURNAL OF ENERGY STORAGE(2024)
摘要
As an important energy storage device, accurate prediction of the state of health (SOH) in lithium-ion batteries is necessary to ensure their safe and stable operation. While current data-driven methods for SOH prediction are promising, attaining high accuracy with minimal battery data continues to be a significant challenge. An extreme learning machine (ELM) model based on particle swarm optimization (PSO) integrated with Levy flight and generalized opposition-based learning (GOBL) algorithms is proposed. The Levy flight helps PSO increase particle diversity, thereby improving the accuracy of battery SOH prediction. At the same time, the GOBL algorithm limits the particle boundaries of Levy flight, thereby enhancing the particle convergence. The charging time, discharging time, and time interval of equal voltage drop serve as the input vectors for the prediction model, while the battery SOH is regarded as the output vector. The results show that the proposed SOH prediction ELM method with the integration of PSO modified by Levy flight and GOBL has better applicability than those using a simple ELM or an ELM integrated with PSO for both LiNi0.8Co0.15Al0.05O2 (LI-NCA) and LiFePO4 (LFP) batteries. When the proportion of the training set is 20 %, the maximum absolute error of SOH prediction is <2.85 % for both LI-NCA and LFP batteries.
更多查看译文
关键词
Lithium -ion batteries,State of health prediction,Extreme learning machine,Particle swarm optimization,Levy flight,Generalized opposition -based learning
AI 理解论文
溯源树
样例
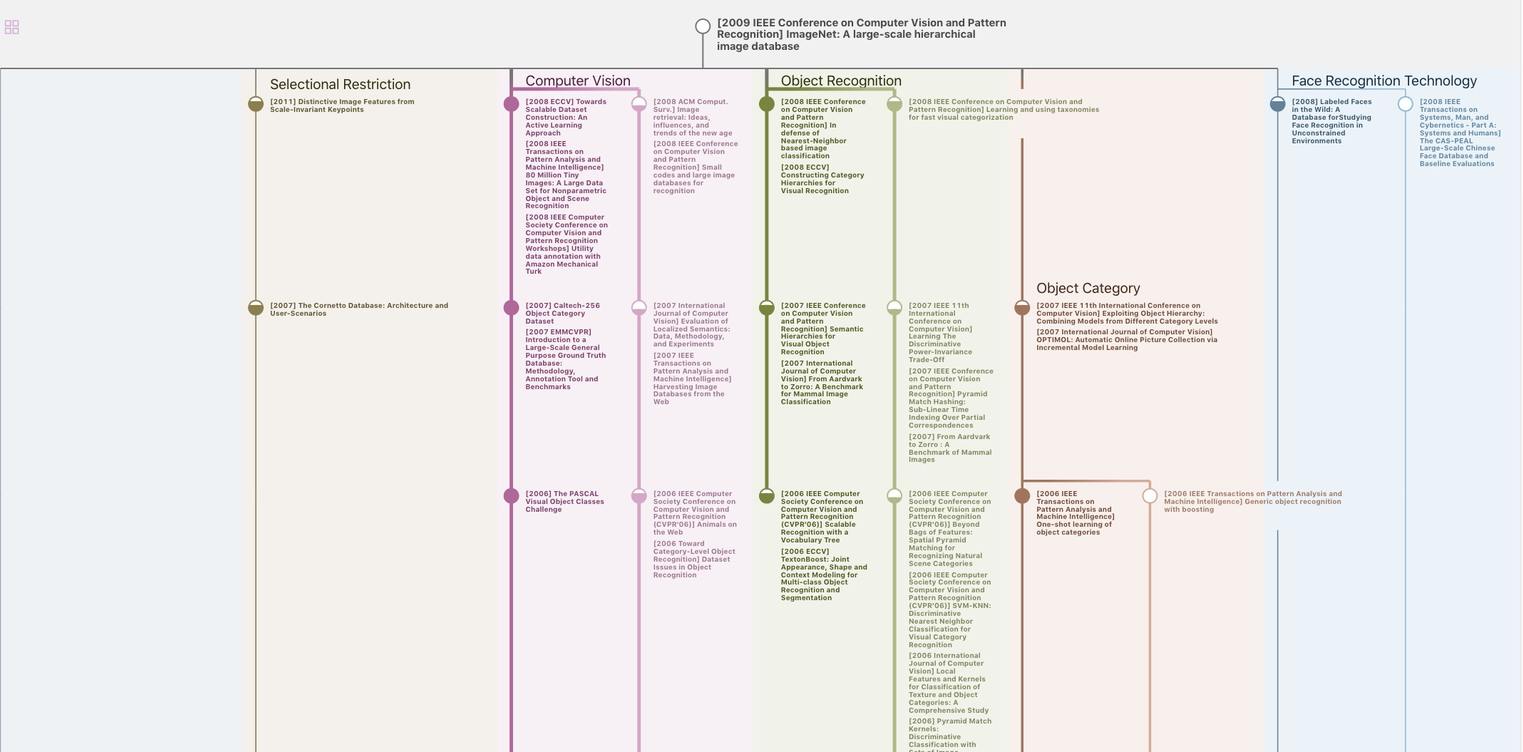
生成溯源树,研究论文发展脉络
Chat Paper
正在生成论文摘要