Nonlinear network-based quantitative trait prediction from biological data
JOURNAL OF THE ROYAL STATISTICAL SOCIETY SERIES C-APPLIED STATISTICS(2024)
摘要
Quantitatively predicting phenotypic variables using biomarkers is a challenging task for several reasons. First, the collected biological observations might be heterogeneous and correspond to different biological mechanisms. Second, the biomarkers used to predict the phenotype are potentially highly correlated since biological entities (genes, proteins, and metabolites) interact through unknown regulatory networks. In this paper, we present a novel approach designed to predict multivariate quantitative traits from biological data which address the 2 issues. The proposed model performs well on prediction but it is also fully parametric, with clusters of individuals and regulatory networks, which facilitates the downstream biological interpretation.
更多查看译文
关键词
block-diagonal covariance matrix,clustering,mixture of regressions,network inference,nonlinear regression,slope heuristics
AI 理解论文
溯源树
样例
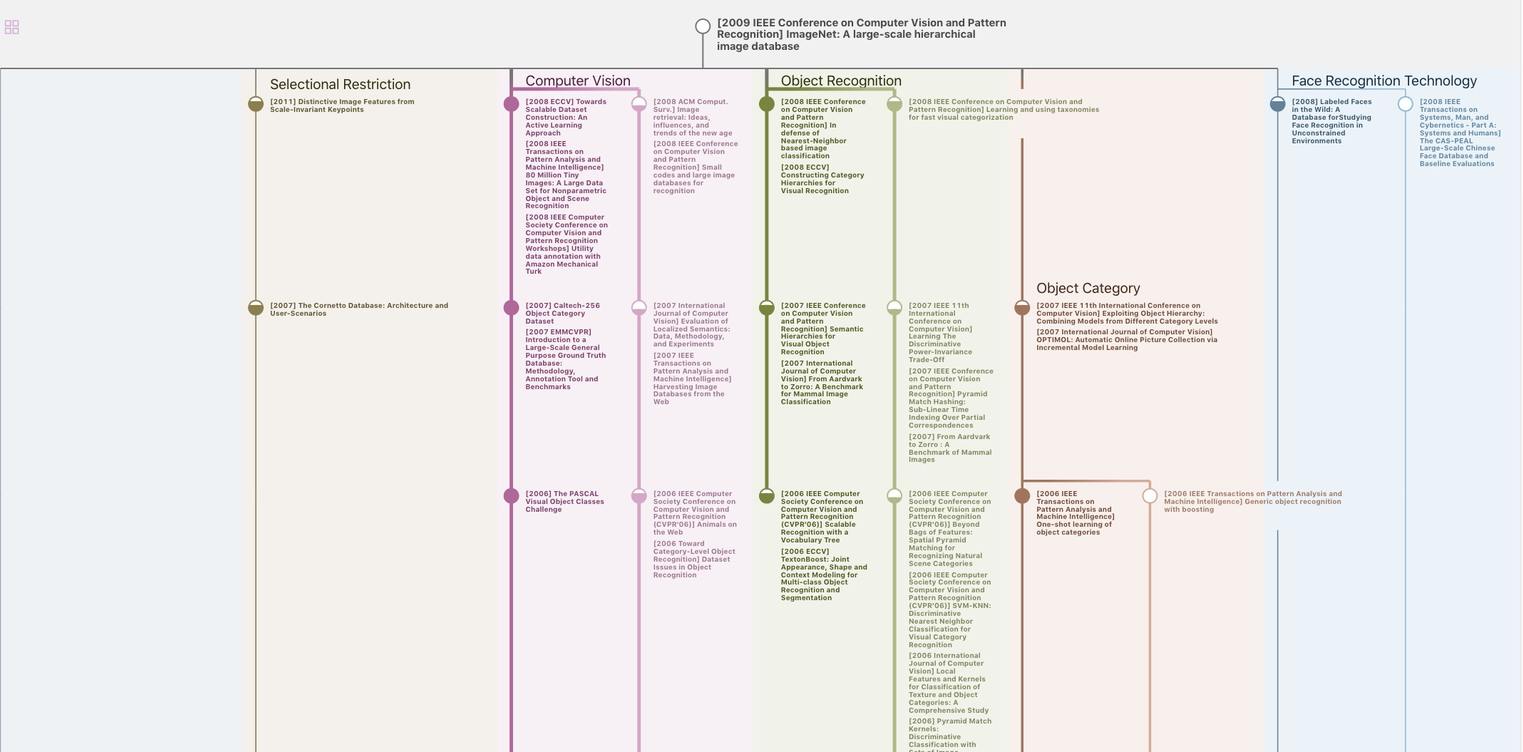
生成溯源树,研究论文发展脉络
Chat Paper
正在生成论文摘要