Optimizing E-waste management: Deep learning classifiers for effective planning
JOURNAL OF CLEANER PRODUCTION(2024)
Abstract
E-waste demands urgent attention to devise effective and sustainable waste management solutions. In response to this challenge, the integration of deep learning classifiers emerges as a promising avenue to optimize the planning of e-waste collection. By harnessing the capabilities of deep learning, these classifiers yield enriched navigational insights, facilitating more informed asset allocation and strategic interventions. This synthesis of technology-driven planning holds the potential to address the intricate intricacies of e-waste in a sustainable environmental landscape. Furthermore, the identification of important parts in e-waste helps in improving recycling processes, adding to a more maintainable and harmless ecosystem. To improve the potential efficiency and accuracy in waste collection the proposed methodology of Adaptive V3 and Federated Learning (Adaptive V3 and FL) are proposed to optimize e-waste collecting planning. The Inception V3 structure can enhance the classification of the e-waste, also the L2 regularization is utilized to overcome the overfitting issues. Also, federated learning is employed to improve the performance of the Inception V3 with the L2 Regularization approach. The datasets like the Starter e-waste dataset and the Compressed E-waste dataset are employed for the e-waste classification. The performance metrics like accuracy, precision, recall, specificity, and f1-score are utilized for the classification. The overall performance achieved by the proposed method is 98.9 % and this maximized the performance than existing approaches such as DCNN, DLRNN, LSTM as well as BSFC which attained 78.4 %, 78.1 %, 58.6 %, and 39.4 % respectively. This research overcomes the proposed strategy in revolutionizing e-waste collecting, planning, and advancing a technology-driven approach to e-waste management. Further, it is performed to perform the effective e-waste classification with multiple wastes using large equipment.
MoreTranslated text
Key words
Electronic waste,Deep learning,E-Waste management,Waste collection,Inception V3 structure,Federated learning
AI Read Science
Must-Reading Tree
Example
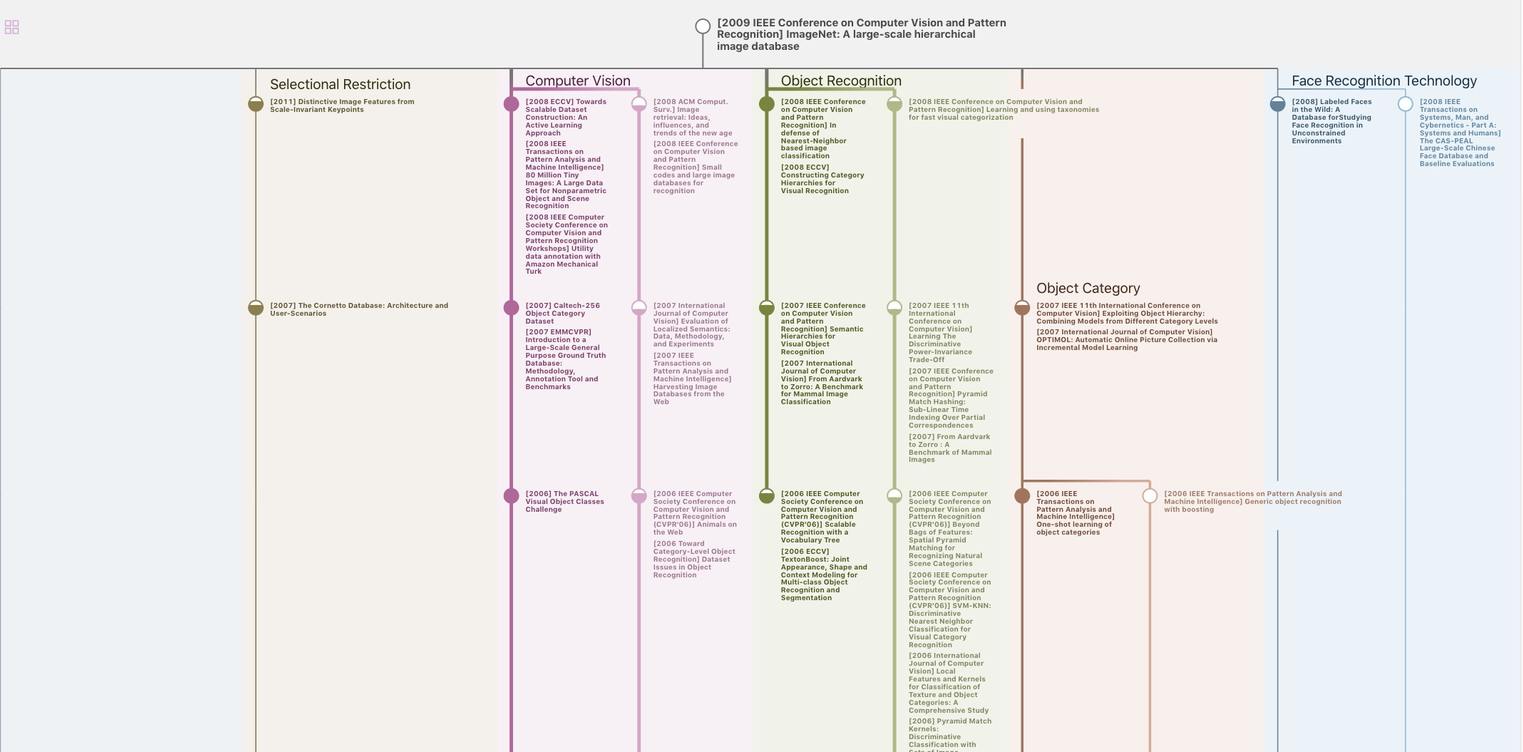
Generate MRT to find the research sequence of this paper
Chat Paper
Summary is being generated by the instructions you defined