Spatial-spectral graph convolutional extreme learning machine for hyperspectral image classification
INTERNATIONAL JOURNAL OF REMOTE SENSING(2024)
摘要
Hyperspectral image has excellent spectral information and abundant spatial information, and its feature quality is one of the key factors affecting the classification performance. Extreme learning machine (ELM) is widely used in hyperspectral classification problems. However, traditional ELM is usually based on regular Euclidean data, ignoring the inherent structured information between hyperspectral pixels, resulting in poor robustness. In this paper, we propose a new supervised extreme learning machine framework, termed spatial-spectral graph convolutional ELM (SSG-ELM), based on using graph convolution to extend ELM into the non-Euclidean domain. The method inherits all the advantages from ELM, and consists of a random graph convolutional layer followed by a graph convolutional regression layer, enabling it to model complex intra class variations. Otherwise, a local spectral-spatial context integration and reshaping mechanism is incorporated into the hidden layer feature representation by using a context-aware bilateral filtering procedure. Experimental results show that the proposed algorithm obtains a competitive performance and outperforms other state-of-the-art ELM-based methods.
更多查看译文
关键词
Hyperspectral image,supervised classification,graph convolutional network,extreme learning machine (ELM)
AI 理解论文
溯源树
样例
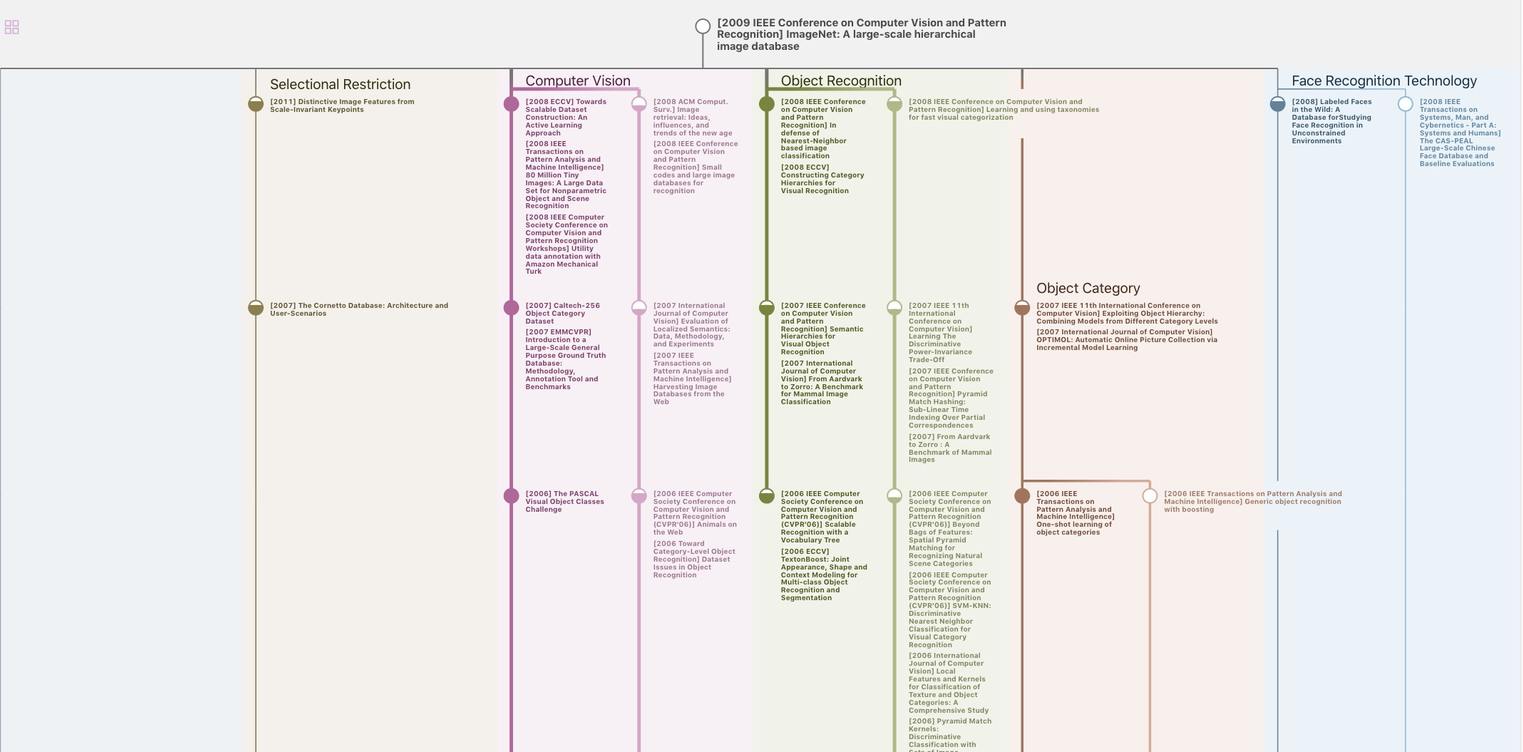
生成溯源树,研究论文发展脉络
Chat Paper
正在生成论文摘要