Density Functional Theory-Assisted Active Learning-Driven Organic Ligand Design for CsPbBr3 Nanocrystals
JOURNAL OF PHYSICAL CHEMISTRY C(2024)
摘要
Surface modification with organic ligands is pivotal in enhancing the stability and passivation of perovskite nanocrystals. Traditionally, the design of these ligands has predominantly been dependent on the expertise and intuition of researchers. We develop a density functional theory-assisted active learning framework to screen potential surface ligands for CsPbBr3 nanocrystals in a large chemical space using dual-objective Bayesian optimization. Our approach has successfully identified a stable Pareto front after seven optimization iterations, with the resulting surrogate model demonstrating accurate predictions for the adsorption energies of newly proposed molecules. Six promising candidates in Pareto solutions without electronic traps are obtained through a mere 80 calculations from 161,900 molecule spaces. Conspicuous enrichment of featured fragments (halogen, ketone, imine, sulfide, benzene, and their combinations) is observed in the promising data set near the Pareto front, which coincides with the features of most reported excellent ligands. Our work demonstrates a highly efficient active learning framework to accelerate the ligand design for PNCs by rapidly screening the large-scale molecule space using the data-driven workflow.
更多查看译文
AI 理解论文
溯源树
样例
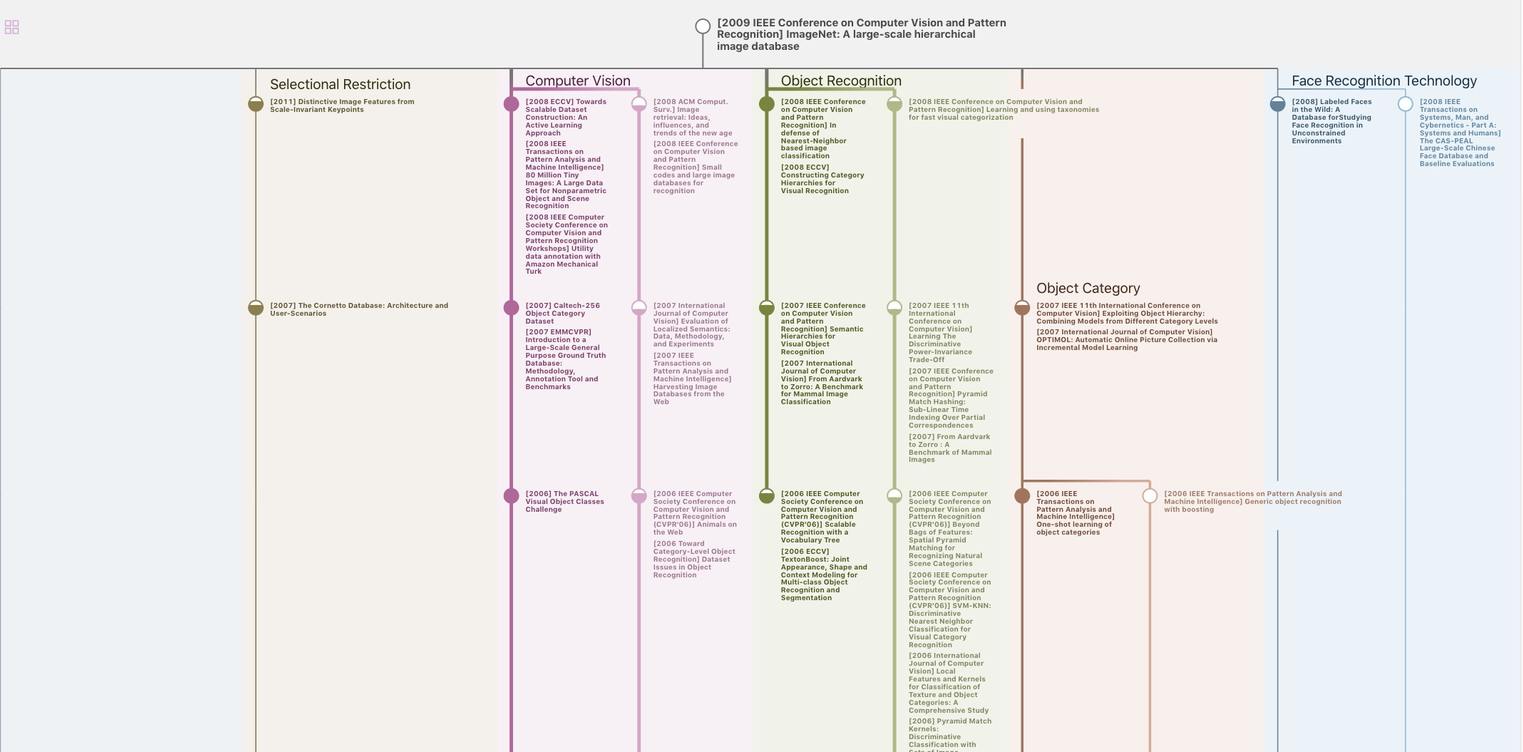
生成溯源树,研究论文发展脉络
Chat Paper
正在生成论文摘要