Rectifying inaccurate unsupervised learning for robust time series anomaly detection
INFORMATION SCIENCES(2024)
摘要
Unsupervised time series anomaly detection is a challenging task. Data contamination brings more challenges for the existing methods that rely on completely clean training data. Moreover, sparse anomaly knowledge leads to the deviation of the learned normality boundary. In this work, we propose a time series anomaly detection method, namely, Rectified Inaccurate Anomaly Detection (RiAD), for training an anomaly detector under data contamination. Specifically, to improve the normality description learned from the data, we propose two key components: an Augmented Uncertainty Estimation Module and Adaptive Reconstruction Loss. These components adaptively penalize uncertainty prediction and anomalous outliers to enforce the learning of valid normality description from normal samples instead of anomalous ones. Furthermore, we design an Anomaly Injection Module, which injects anomaly knowledge into the model by generating different types of simulated anomaly examples to learn accurate normality boundary and utilizes the Awareness Memory Module to prevent unexpected generalization of anomalous information. Extensive experiments on ten real -world datasets demonstrate the superiority of the proposed method over state-of-the-art methods and achieve superior performance in settings with different levels of anomaly contamination.
更多查看译文
关键词
Uncertainty estimation,Adaptive reconstruction,Anomaly injection,Awareness memory module,Time series,Anomaly detection
AI 理解论文
溯源树
样例
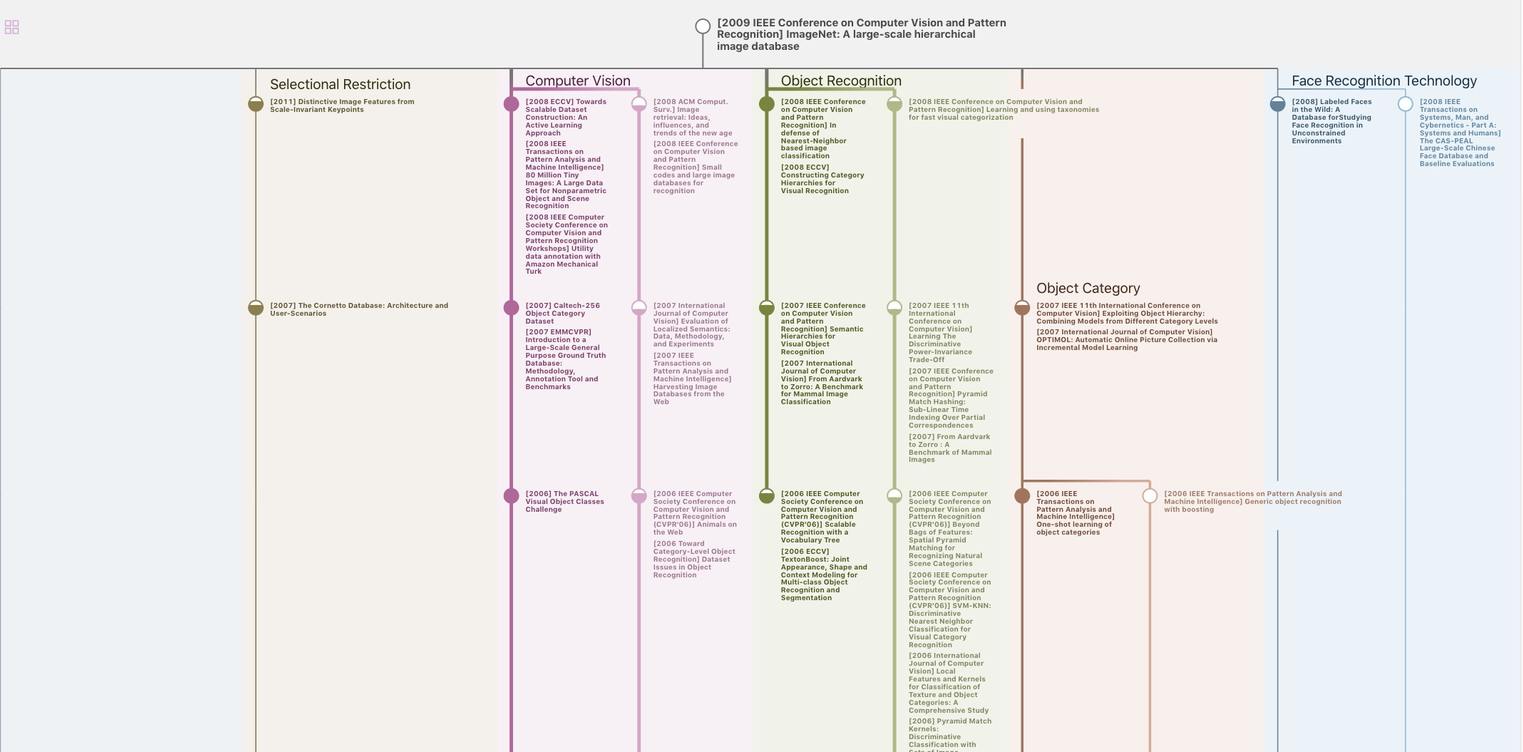
生成溯源树,研究论文发展脉络
Chat Paper
正在生成论文摘要