Multi-modal zero-shot dynamic hand gesture recognition
EXPERT SYSTEMS WITH APPLICATIONS(2024)
摘要
Zero-Shot Learning (ZSL) has rapidly advanced in recent years. Towards overcoming the annotation bottleneck in the Dynamic Hand Gesture Recognition (DHGR), we explore the idea of Zero-Shot Dynamic Hand Gesture Recognition (ZS-DHGR) with no annotated visual examples, by leveraging their textual descriptions. In this way, we propose a multi-modal Zero-Shot Dynamic Hand Gesture Recognition (ZS-DHGR) model harnessing from the complementary capabilities of deep features fused with the skeleton-based ones. A Transformerbased model along with a C3D model is used for hand detection and deep features extraction, respectively. To make a trade -off between the dimensionality of the skeleton-based and deep features, we use an AutoEncoder (AE) on top of the Long Short Term Memory (LSTM) network. Finally, a semantic space is used to map the visual features to the lingual embedding of the class labels, achieved via the Bidirectional Encoder Representations from Transformers (BERT) model. Results on four large-scale datasets, RKS-PERSIANSIGN, First-Person, ASLVID, and isoGD, show the superiority of the proposed model compared to state-of-the-art alternatives in ZS-DHGR. The proposed model obtains an accuracy of 74.6% , 67.2% , 68.8% , 60.2% on the RKS-PERSIANSIGN, First-Person, ASLVID, and isoGD datasets, respectively.
更多查看译文
关键词
Hand gesture recognition,Zero-shot learning,Deep learning,Transformer,Multi-modal
AI 理解论文
溯源树
样例
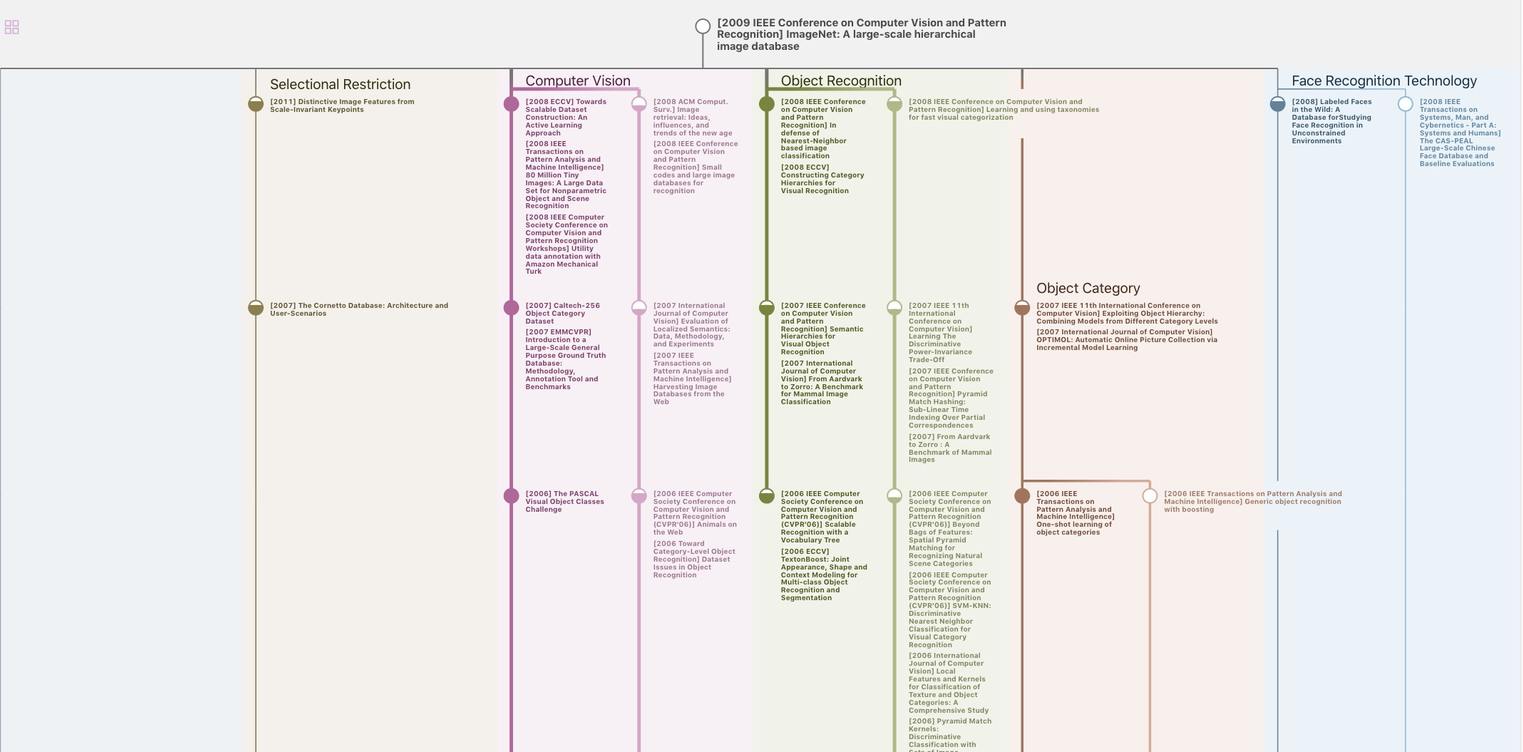
生成溯源树,研究论文发展脉络
Chat Paper
正在生成论文摘要