Predicting heart failure outcomes by integrating breath-by-breath measurements from cardiopulmonary exercise testing and clinical data through a deep learning survival neural network
EUROPEAN HEART JOURNAL - DIGITAL HEALTH(2024)
摘要
Aims Mathematical models previously developed to predict outcomes in patients with heart failure (HF) generally have limited performance and have yet to integrate complex data derived from cardiopulmonary exercise testing (CPET), including breath-by-breath data. We aimed to develop and validate a time-to-event prediction model using a deep learning framework using the DeepSurv algorithm to predict outcomes of HF.Methods and results Inception cohort of 2490 adult patients with high-risk cardiac conditions or HF underwent CPET with breath-by-breath measurements. Potential predictive features included known clinical indicators, standard summary statistics from CPETs, and mathematical features extracted from the breath-by-breath time series of 13 measurements. The primary outcome was a composite of death, heart transplant, or mechanical circulatory support treated as a time-to-event outcomes. Predictive features ranked as most important included many of the features engineered from the breath-by-breath data in addition to traditional clinical risk factors. The prediction model showed excellent performance in predicting the composite outcome with an area under the curve of 0.93 in the training and 0.87 in the validation data sets. Both the predicted vs. actual freedom from the composite outcome and the calibration of the prediction model were excellent. Model performance remained stable in multiple subgroups of patients.Conclusion Using a combined deep learning and survival algorithm, integrating breath-by-breath data from CPETs resulted in improved predictive accuracy for long-term (up to 10 years) outcomes in HF. DeepSurv opens the door for future prediction models that are both highly performing and can more fully use the large and complex quantity of data generated during the care of patients with HF. Graphical Abstract
更多查看译文
关键词
Heart failure,Prognosis,Machine learning,Survival analysis,Exercise testing
AI 理解论文
溯源树
样例
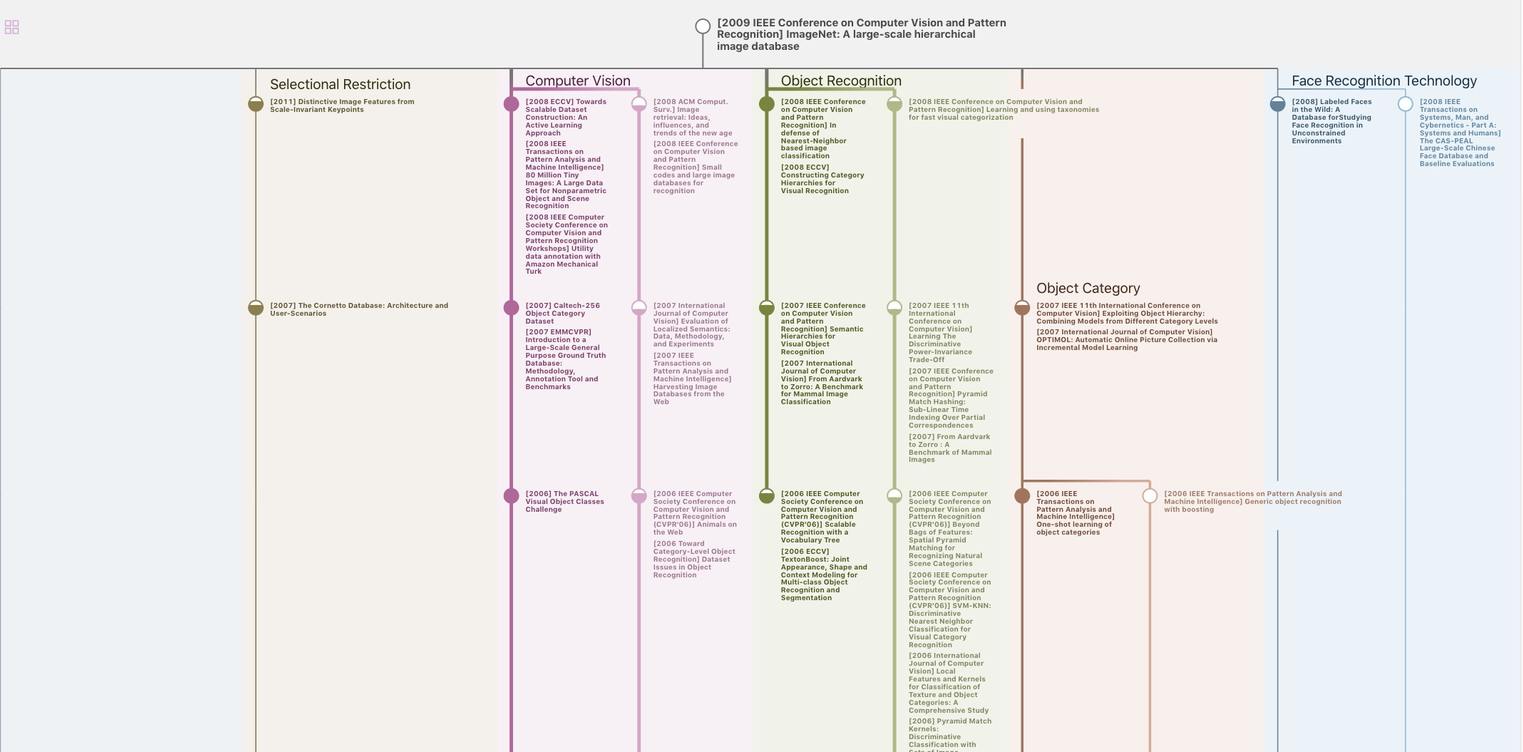
生成溯源树,研究论文发展脉络
Chat Paper
正在生成论文摘要