Prediction of Non-Muscle Invasive Papillary Urothelial Carcinoma Relapse from Hematoxylin-Eosin Images Using Deep Multiple Instance Learning in Patients Treated with Bacille Calmette-Gurin Immunotherapy
BIOMEDICINES(2024)
摘要
The limited reproducibility of the grading of non-muscle invasive papillary urothelial carcinoma (NMIPUC) necessitates the search for more robust image-based predictive factors. In a cohort of 157 NMIPUC patients treated with Bacille Calmette-Guerin (BCG) immunotherapy, we explored the multiple instance learning (MIL)-based classification approach for the prediction of 2-year and 5-year relapse-free survival and the multiple instance survival learning (MISL) framework for survival regression. We used features extracted from image patches sampled from whole slide images of hematoxylin-eosin-stained transurethral resection (TUR) NPMIPUC specimens and tested several patch sampling and feature extraction network variations to optimize the model performance. We selected the model showing the best patient survival stratification for further testing in the context of clinical and pathological variables. MISL with the multiresolution patch sampling technique achieved the best patient risk stratification (concordance index = 0.574, p = 0.010), followed by a 2-year MIL classification. The best-selected model revealed an independent prognostic value in the context of other clinical and pathologic variables (tumor stage, grade, and presence of tumor on the repeated TUR) with statistically significant patient risk stratification. Our findings suggest that MISL-based predictions can improve NMIPUC patient risk stratification, while validation studies are needed to test the generalizability of our models.
更多查看译文
关键词
digital image analysis,bladder cancer,deep learning,cancer prognosis,survival prediction,feature extraction
AI 理解论文
溯源树
样例
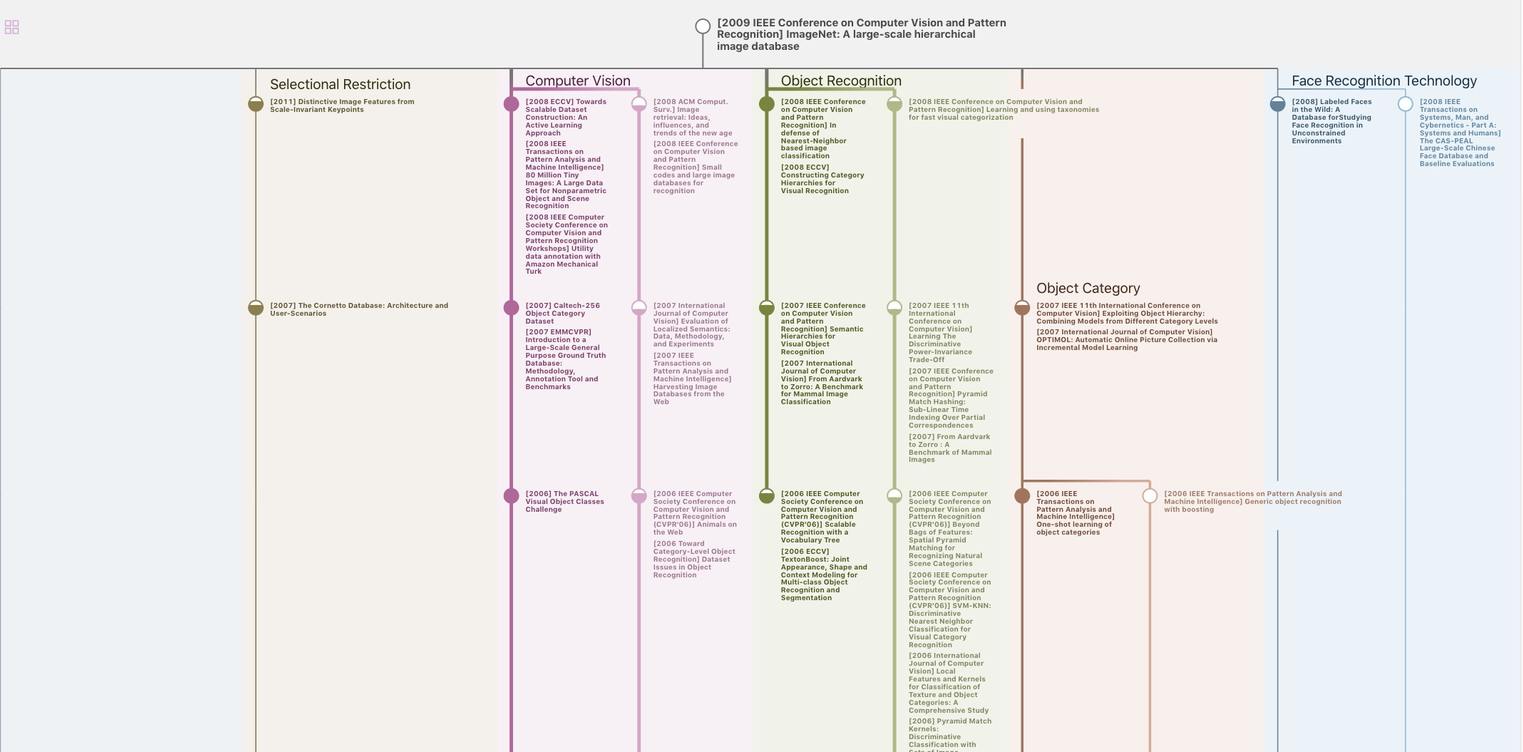
生成溯源树,研究论文发展脉络
Chat Paper
正在生成论文摘要