Aspect-Level sentiment analysis based on fusion graph double convolutional neural networks
INTERNATIONAL JOURNAL OF PARALLEL EMERGENT AND DISTRIBUTED SYSTEMS(2024)
摘要
Aspect-based sentiment analysis (ABSA) aims to analyze the emotional color contained in sentences or documents in more detail by classifying and evaluating different aspects and emotions in the text. However, the current research methods cannot effectively analyze the relationship between aspect words and context and extract grammatical information about sentences. Additionally, the extracted syntactic information is insufficient, and the combination of syntactic and semantic information is inefficient, leaving the model unable to correctly determine aspects' emotional orientations. This paper proposes an aspect-level sentiment analysis based on Fusion Graph Double Convolutional Neural Networks (FGD-GCN) to address these issues. Firstly, FGD-GCN proposes a multi-feature extraction module. Using BERT and bidirectional long-short-term memory models, this module extracts the hidden context between words. In addition, the positional attention module is used to capture important features in sentences, reducing noise and bias. Then, a semantic enhancement module is proposed, which fuses attention-focused information and feature information extracted from graphs to emphasize aspect words and context, and uses CNN model to classify on feature vectors. According to experiment results on three benchmark datasets, the model outperforms previous GCN methods for context-based aspect-level sentiment analysis.
更多查看译文
关键词
Graph convolutional network,convolutional neural network,aspect-based sentiment analysis,BERT
AI 理解论文
溯源树
样例
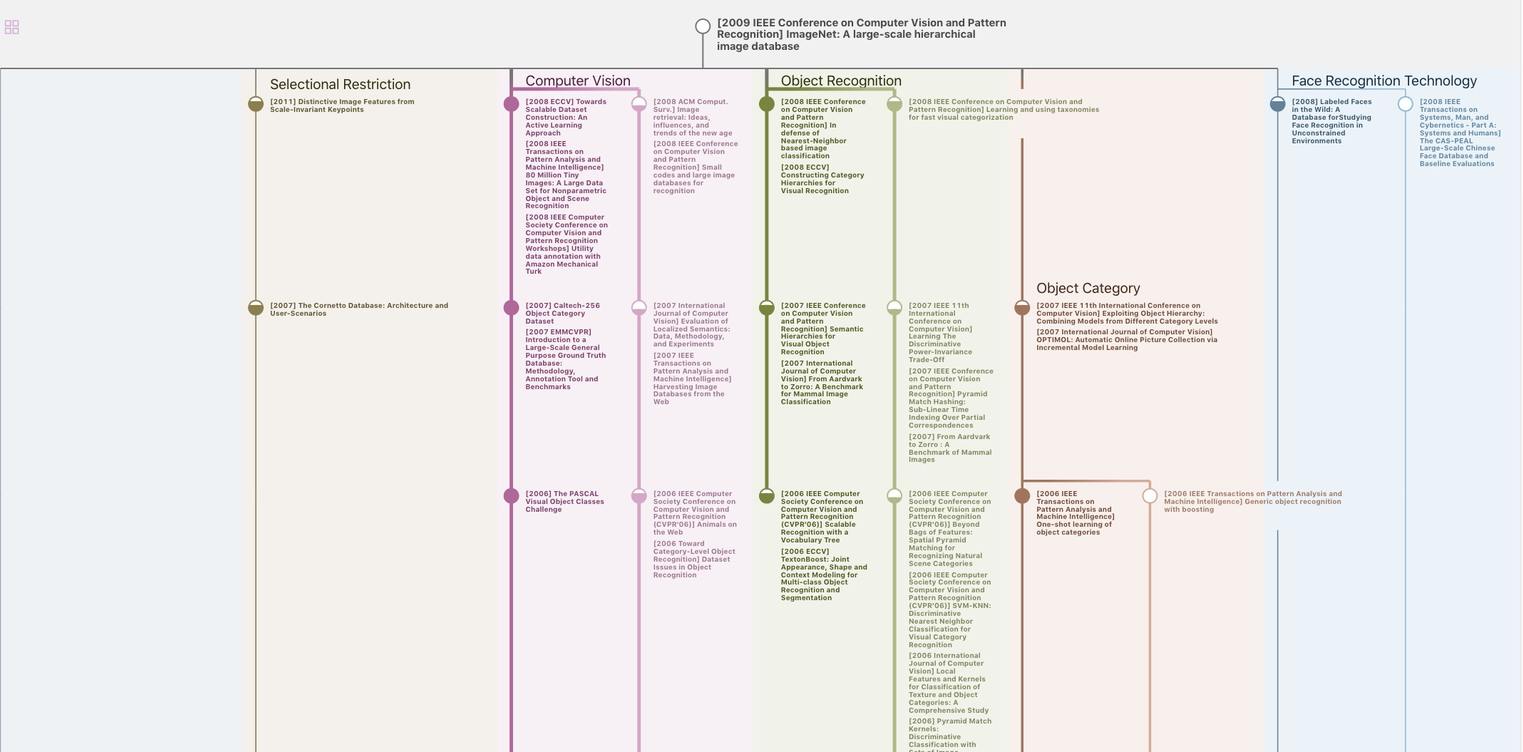
生成溯源树,研究论文发展脉络
Chat Paper
正在生成论文摘要