Point Cloud Completion Network Based on Multiencoders and Residual-Transformer
LASER & OPTOELECTRONICS PROGRESS(2023)
摘要
Point cloud data has the characteristics of disorder and sparsity. The three-dimensional (3D) point cloud completion task of recovering the missing 3D geometric shapes through incomplete point cloud data is a challenging issue in 3D vision technology. The existing 3D point cloud completion network predicts the complete point cloud shape directly from a subset of the point cloud using the Encoder-Decoder model, which interferes with the original part of the point cloud, resulting in noise and geometric displacement loss. In this study, an end-to-end network model is proposed, which focuses on generating a smooth and uniformly distributed point cloud object. The proposed network model mainly consists of the following three parts: missing point cloud prediction, point cloud fusion, and point cloud smoothing. The first module mainly uses multiencoders to extract local information and global information from incomplete point cloud objects to predict the missing geometric parts. The second module merges point cloud objects by sampling algorithm. The third module is based on a Residual-Transformer (RT) to predict the displacement of the points, which can make the point distribution more uniform without destroying the spatial structure of the original input point cloud. On the benchmark dataset, Shapenet-Part, several experimental results indicate that the proposed network has achieved better quantitative results and visual effects in 3D shape completion.
更多查看译文
关键词
point cloud,three-dimensional point cloud completion,self-attention mechanism,multiencoders,residual network
AI 理解论文
溯源树
样例
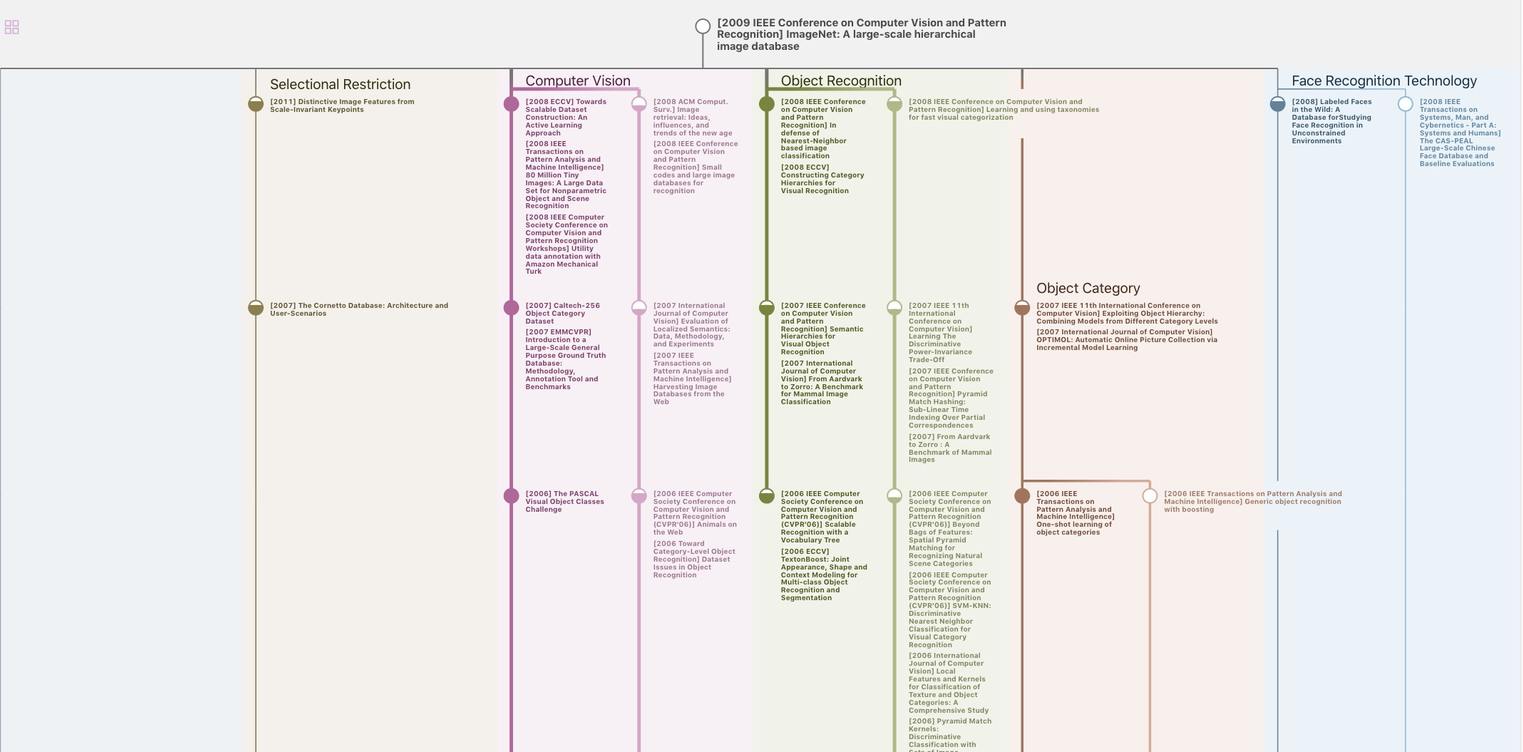
生成溯源树,研究论文发展脉络
Chat Paper
正在生成论文摘要