On the Consistency of Average Embeddings for Item Recommendation
PROCEEDINGS OF THE 17TH ACM CONFERENCE ON RECOMMENDER SYSTEMS, RECSYS 2023(2023)
摘要
A prevalent practice in recommender systems consists of averaging item embeddings to represent users or higher-level concepts in the same embedding space. This paper investigates the relevance of such a practice. For this purpose, we propose an expected precision score, designed to measure the consistency of an average embedding relative to the items used for its construction. We subsequently analyze the mathematical expression of this score in a theoretical setting with specific assumptions, as well as its empirical behavior on real-world data from music streaming services. Our results emphasize that real-world averages are less consistent for recommendation, which paves the way for future research to better align real-world embeddings with assumptions from our theoretical setting.
更多查看译文
关键词
Recommender Systems,Representation Learning,Embedding Vectors,Average Embeddings
AI 理解论文
溯源树
样例
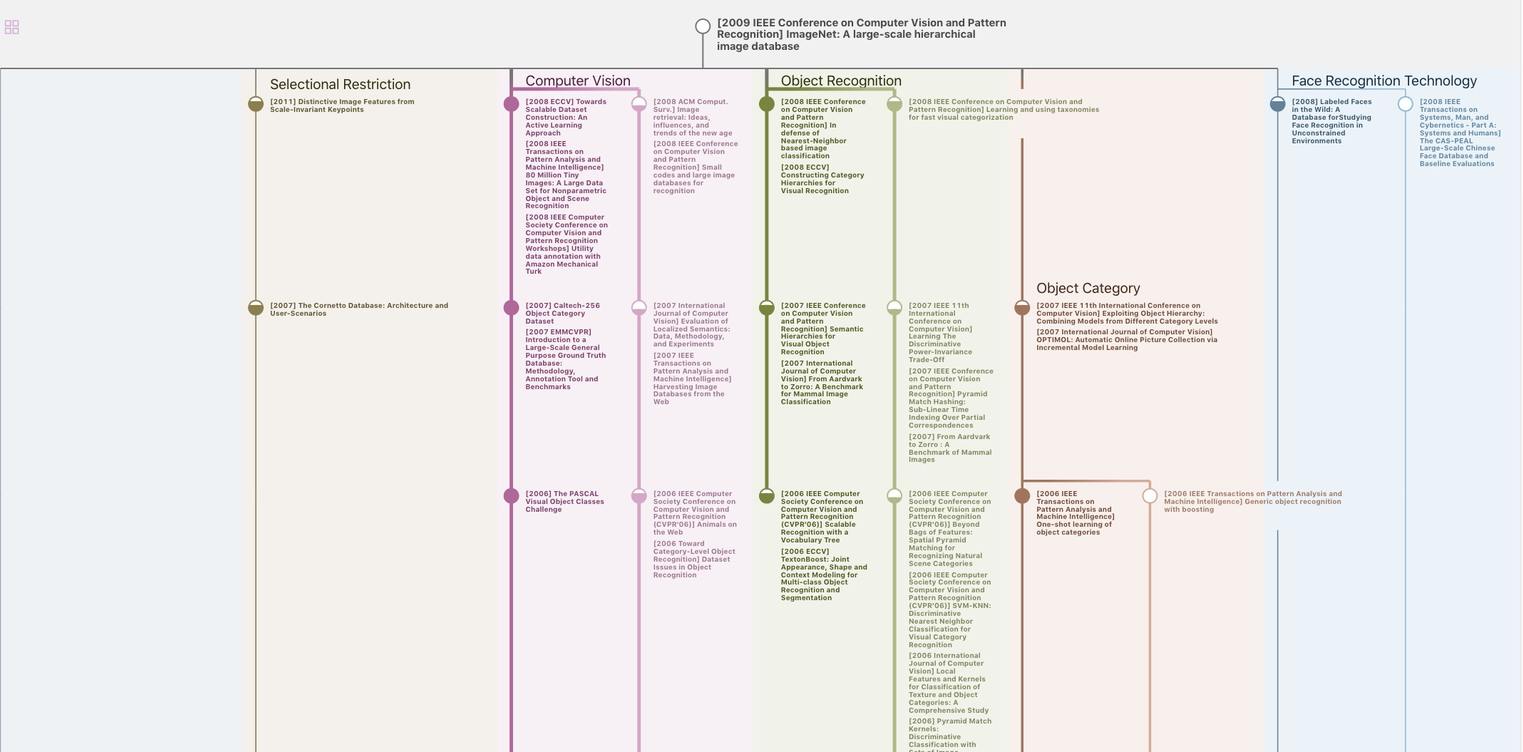
生成溯源树,研究论文发展脉络
Chat Paper
正在生成论文摘要