An insight into viable machine learning models for early diagnosis of cardiovascular disease
SCALABLE COMPUTING-PRACTICE AND EXPERIENCE(2024)
摘要
Cardiovascular diseases (CVD) are a prominent source of death across the globe, and these deaths are taking place in low-to middle-income nations. Due to this, CVD prevention is a pressing issue that has already been the subject of extensive research. Innovative methodologies in machine learning (ML) can have a greater impact on the diagnosis of CVD, yet the research on CVD is more challenging and attracting more research indeed. In this paper, we investigate the differences between four distinct machine learning models, support vector machine (SVM), logistic regression, decision trees (DT), and artificial neural networks (ANN) in their classification accuracy and possible practicality in CVD classification. techniques such as ensemble learning and other model-specific optimizations are not part of this study, but more basic implementations of the various models were used. To implement abovementioned ML models, a subset of 14 features from the original heart disease dataset is considered and deemed relevant for classification where no individual feature data are missing. From the results, it is observed that there is no clear winner in the comparison of models. There is no significant difference in the average accuracy of models. The highest average hit rate is observed in SVM and ANN, however it is slightly lower in ANN. Even though the DT had lower accuracy, the fully trained model can be easily visualized and interpreted by humans. Hence, the DT is possibly the most practical model to use as a complement to doctors in their current methods of diagnosis.
更多查看译文
关键词
Average Classification Accuracy,Cardiovascular Disease,Computer Aided Diagnostics,Machine Learning Algo-rithms,Test Data Split
AI 理解论文
溯源树
样例
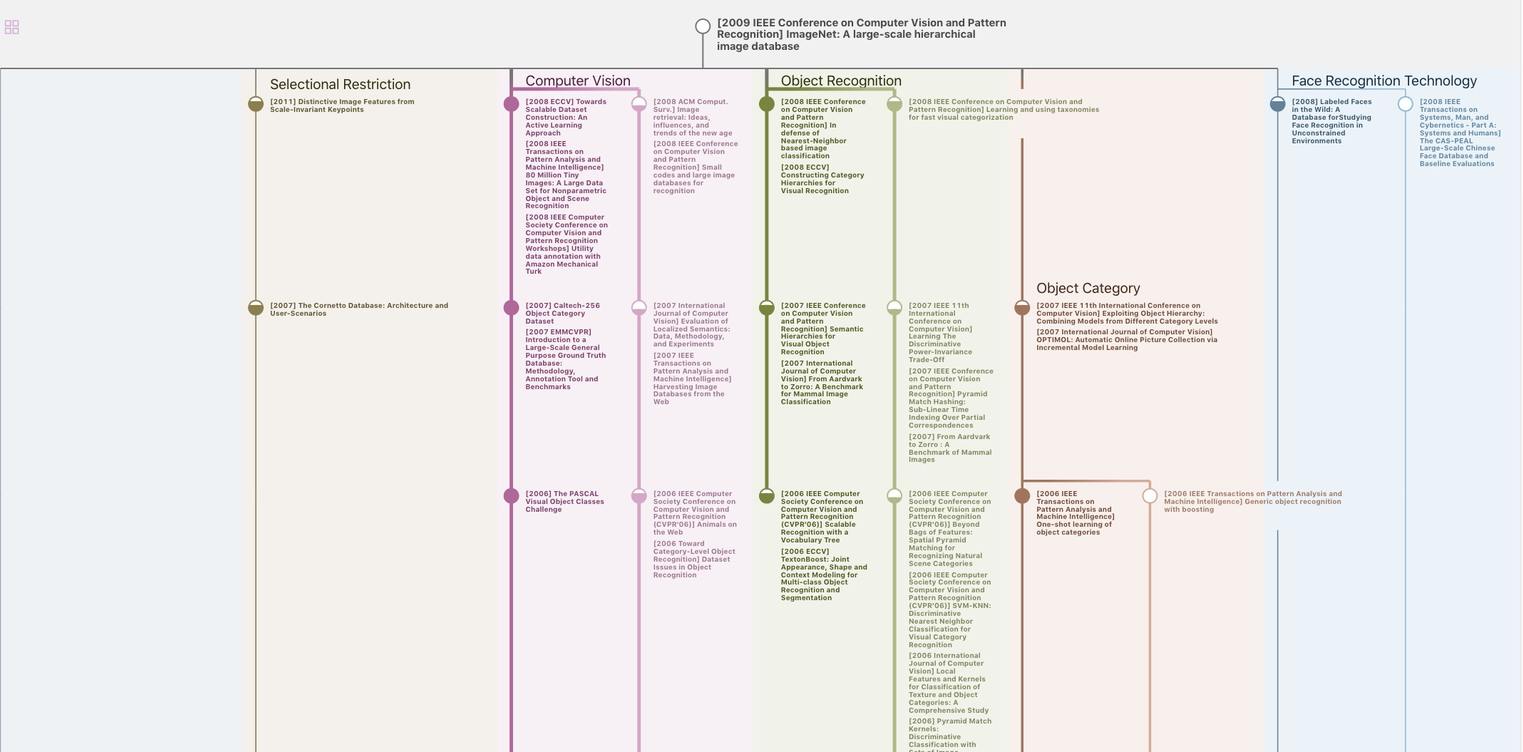
生成溯源树,研究论文发展脉络
Chat Paper
正在生成论文摘要