Towards Motor Imagery Classification of Finger Tapping by Both Hands for Controlling a Finger-Arm Bionic Robot.
International Winter Conference on Brain-Computer Interface(2024)
Abstract
In motor imagery, the greatest attention was paid to decoding signals from large muscles. However, there is a missing piece in helping people with finger issues or paralysis. This study investigates the potential advancements in neurorehabilitation by exploring the discriminative capabilities of electroencephalography (EEG) based classification in finger tapping. To improve neurorehabilitation strategies, we performed a comparative analysis of finger tapping classification during motor imagery and execution tasks. Using EEG signals, we employed machine learning and deep learning techniques, including support vector machines (SVM), linear discriminant analysis (LDA) and EEGNet. Our results indicate promising differences in EEG signatures between the two paradigms, with SVM, LDA, and EEGNet demonstrating varying degrees of efficacy in classification. These findings suggest potential implications for customized neurorehabilitation interventions. The study contributes to the affirmative possibility of effective rehabilitation strategies and is of particular relevance for patients with impaired motor function.
MoreTranslated text
Key words
Electroencephalography,Motor imagery,Finger Tapping,Neurorehabilitation,Machine learning
AI Read Science
Must-Reading Tree
Example
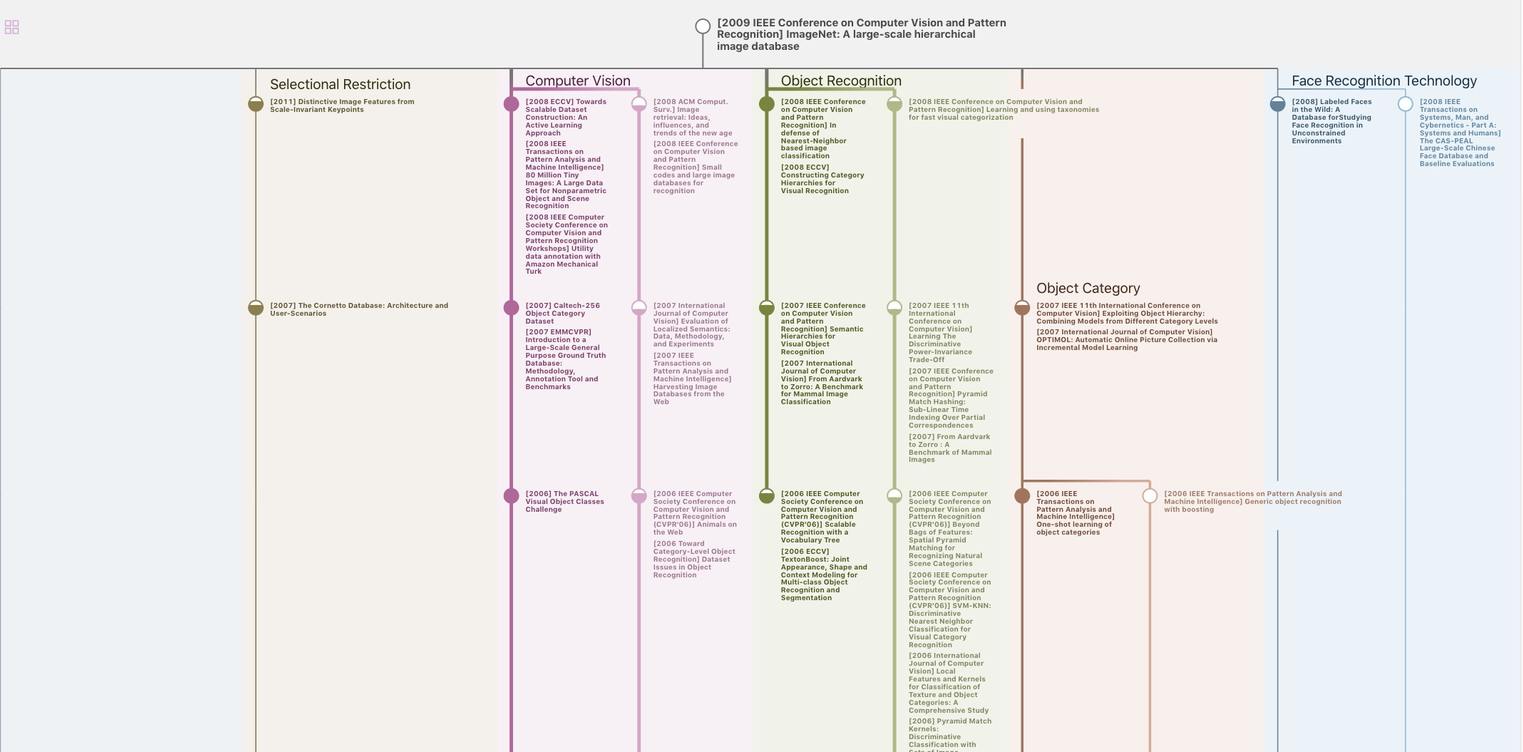
Generate MRT to find the research sequence of this paper
Chat Paper
Summary is being generated by the instructions you defined