PCL: Point Contrast and Labeling for Weakly Supervised Point Cloud Semantic Segmentation
IEEE Transactions on Multimedia(2024)
摘要
Point cloud semantic segmentation is a fundamental task in 3D scene understanding and has recently achieved remarkable progress. The success of existing approaches is attributed to recent advanced deep networks for point clouds and the availability of a large amount of labeled training data. However, creating such fully annotated training datasets for supervised point cloud semantic segmentation methods is a time-consuming and labor-intensive process, which increases the difficulty of extending supervised approaches to new application scenarios. To alleviate the data-hungry nature of deep learning, we propose PCL, the point contrast and labeling framework for weakly supervised point cloud semantic segmentation with small percentages of point-level annotations. The core idea of this method is to exploit contrastive learning to help learn a larger number of discriminative feature representations with limited annotations. By introducing two types of contrastive relationships, cross-sample point contrast and low-level similarity-based point contrast, our proposed framework can directly regularize the learned feature space, considering not only the low-level similarity within each point cloud but also the discriminative semantics within and across point clouds on both labeled and unlabeled points via pseudo labels. In addition, we propose a pseudo label refinery module to generate robust and reliable pseudo labels online, reducing the negative impact of incorrect pseudo labels. Our method achieves state-of-the-art performance on a diverse set of label-efficient semantic segmentation tasks.
更多查看译文
关键词
Point cloud,semantic segmentation,weakly supervised learning,contrastive learning
AI 理解论文
溯源树
样例
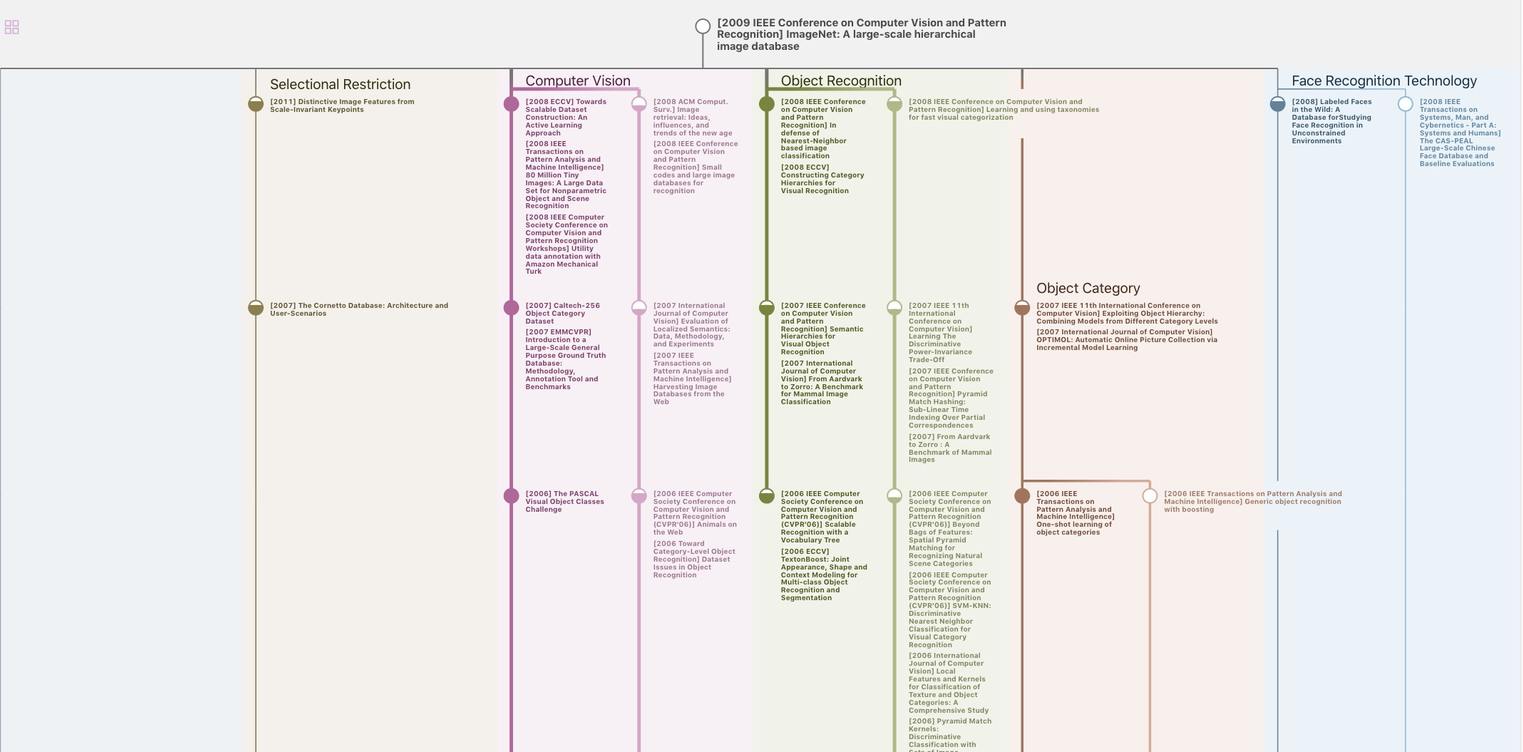
生成溯源树,研究论文发展脉络
Chat Paper
正在生成论文摘要