OEDASA: Optimization Enabled Error-Diluted Approximate Systolic Array Design for an Image Processing Application.
International Conference on VLSI Design(2024)
摘要
Systolic Array (SA) architecture is a hardware accelerator for running Artificial Intelligence (AI) workloads. Although approximate computing offers hardware and performance benefits, it often sacrifices accuracy, limiting its application to error-resilient tasks. Many inexact multipliers in SA exhibit one-sided Error Distribution (ErD), resulting in significant accumulated errors. Approximate Computing proves valuable in error-resilient image processing applications, fostering research into various approximation techniques for enhanced hardware performance. This paper explores SA architecture with different configurations of approximate multipliers (AMs) featuring distinct ErDs. It employs a meta-heuristic optimization algorithm, Particle Swarm optimization (PSO), for image smoothing tasks. The study delves into trade-offs between hardware acceleration and accuracy, offering insights for advancing approximate computing in SA-based AI workloads. The PSO-evolved SA configuration showcases a 3.05% performance improvement, 10.63% silicon footprint reduction, and 10.32% power savings compared to the exact SA. The PSO-derived SA structure also offers notable hardware gains when compared with other state-of-the-art (SOTA) benchmark designs. Additionally, it demonstrates a better Structural Similarity Index (SSIM) compared to SA with one-sided error-distributed AMs. The proposed spatially optimized SAs with AMs of different ErDs represent a step towards reliable hardware design and establishing a nearly exact AI accelerator system. All design files and results are shared openly with the research and design community for easy adoption and further exploration.
更多查看译文
关键词
Systolic Array,Convolution,Approximate Computing,Image Processing,Particle Swarm Optimisation (PSO)
AI 理解论文
溯源树
样例
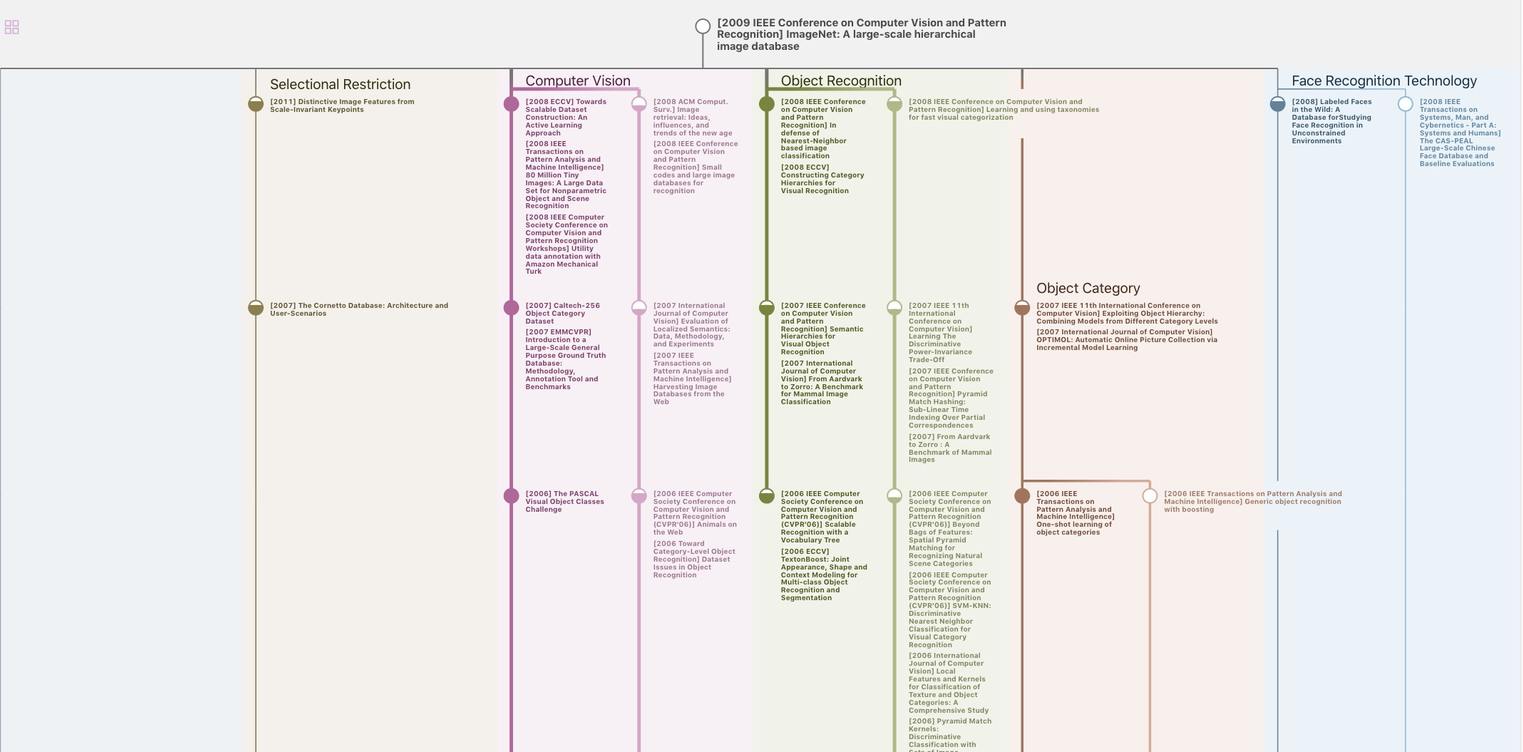
生成溯源树,研究论文发展脉络
Chat Paper
正在生成论文摘要