CardioGPT: An ECG Interpretation Generation Model
IEEE ACCESS(2024)
摘要
Numerous supervised learning models aimed at classifying 12-lead electrocardiograms into different groups have shown impressive performance by utilizing deep learning algorithms. However, few studies are dedicated to applying the Generative Pre-trained Transformer (GPT) model in interpreting electrocardiogram (ECG) using natural language. Thus, we are pioneering the exploration of this uncharted territory by employing the CardioGPT model to tackle this challenge. We used a dataset of ECGs (standard 10s, 12-channel format) from adult patients, with 60 distinct rhythms or conduction abnormalities annotated by board-certified, actively practicing cardiologists. The ECGs were collected from The First Affiliated Hospital of Ningbo University and Shanghai East Hospital. The dataset is partitioned into training (80%), validation (10%), and test (10%) cohorts for comprehensive evaluation. Each cohort contains ECGs from distinct patients, considering some patients took repeated ECG measurements. The proposed algorithm is evaluated in two levels, self-performance measurement and comparison with the residual neural network classification model. Two scores are used for self-performance measurement, including Bilingual Evaluation Understudy (BLEU) and Recall-Oriented Understudy for Gisting Evaluation (ROUGE). To compare the performance of the proposed model with the residual neural network model, we assessed the F1 score and area under the receiver operating characteristic curve (AUC). We have observed promising performance metrics across multiple evaluation criteria through an extensive evaluation of a large 12-lead ECG database comprising 1,128,553 ECG readings from 754,920 patients. The CardioGPT model exhibited high BLEU and ROUGE scores with 0.68 (95% CI: 0.66, 0.71) and 0.81 (95% CI: 0.79, 0.84). Furthermore, in the classification performance measurement setting, the CardioGPT achieved an average F1-score of 0.91(95% CI: 0.89, 0.93) and AUC of 0.82(95% CI: 0.79, 0.84) and has higher scores than that of the convolutional neural network model, indicating its proficiency in accurately classifying ECG recordings. By leveraging the power of transformer structure model and natural language processing, the GPT model addresses the challenge of imbalanced learning commonly encountered in ECG classification tasks. The results indicate that the GPT model can accurately interpret ECG using natural language, providing valuable insights into the underlying patterns and abnormalities present in the data. Significance: The pioneering application of the GPT model for interpreting ECGs with natural language demonstrates its potential to address ECG classification challenges and offer valuable insights into cardiac health.
更多查看译文
关键词
Electrocardiogram,machine learning,generative model,arrhythmia,classification,generative pre-trained transformer
AI 理解论文
溯源树
样例
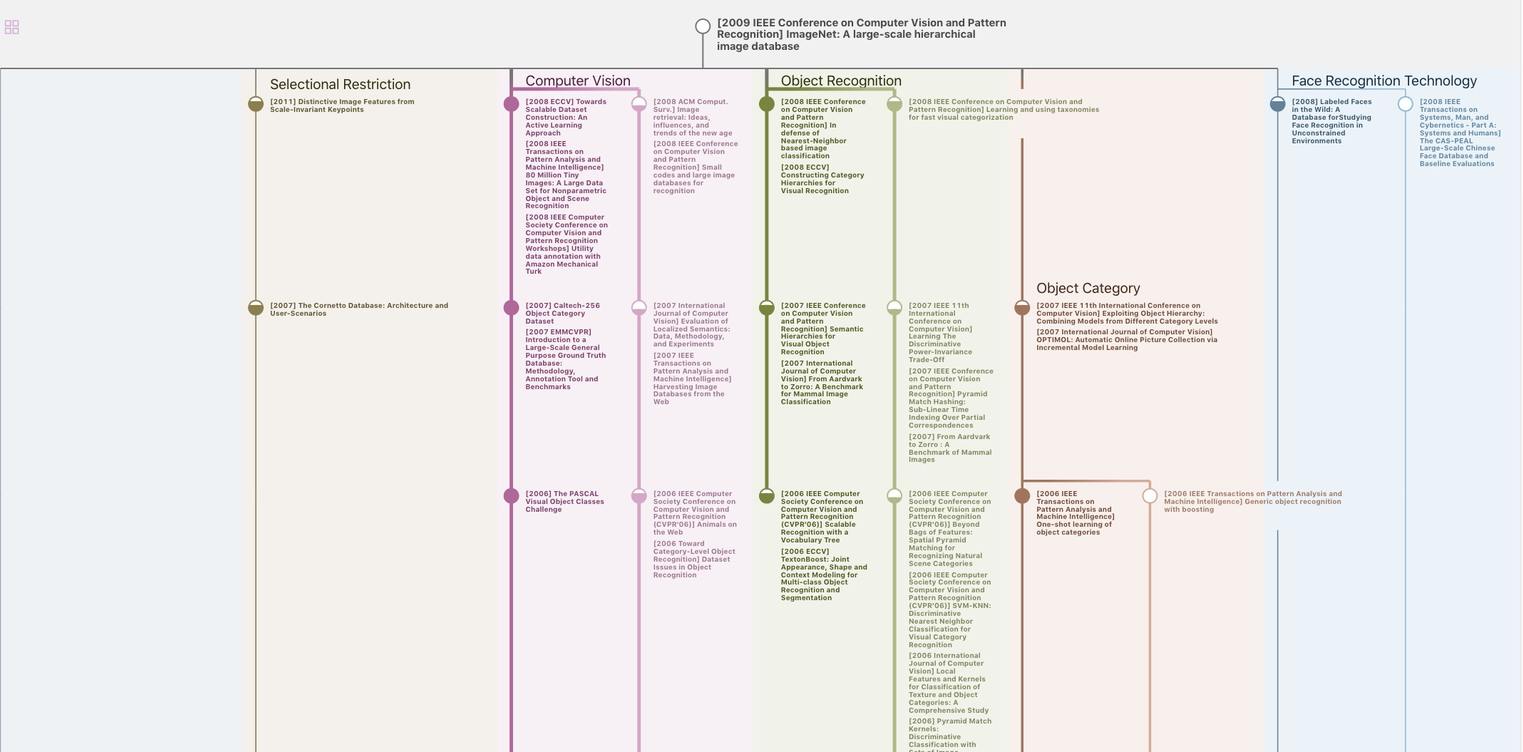
生成溯源树,研究论文发展脉络
Chat Paper
正在生成论文摘要