A Data-Model Fusion Strategy to Improve Detection Performance in the Presence of Target Signal Model Mismatch
IEEE Transactions on Aerospace and Electronic Systems(2024)
摘要
Improving the detection performance of targets embedded in clutter environments has gained significant importance in the radar community over the past few decades. Once the clutter model is determined, detectors for the specific target signal model can be derived, which have been extensively studied in the literature. However, these methods require a priori information about the target signal model and may fail to detect targets when the target signal model is mismatched. In order to improve detection performance when the target signal model mismatches, we propose a data-model fusion (DMF) strategy, which can exploit the information of the actual target signal model in the measured data to correct the mismatched one. By applying the DMF strategy to the conventional method, the robustness of the method can be improved. In this paper, we apply the proposed DMF strategy to the traditional adaptive normalized matched filter (ANMF) and adaptive subspace detector (ASD) to obtain robust DMF-ANMF and DMF-ASD detectors. The detection performance of the proposed methods is evaluated by Monte Carlo simulation and real sea clutter data, and the experimental results verify the effectiveness of the proposed methods.
更多查看译文
关键词
Adaptive signal detection,radar detection
AI 理解论文
溯源树
样例
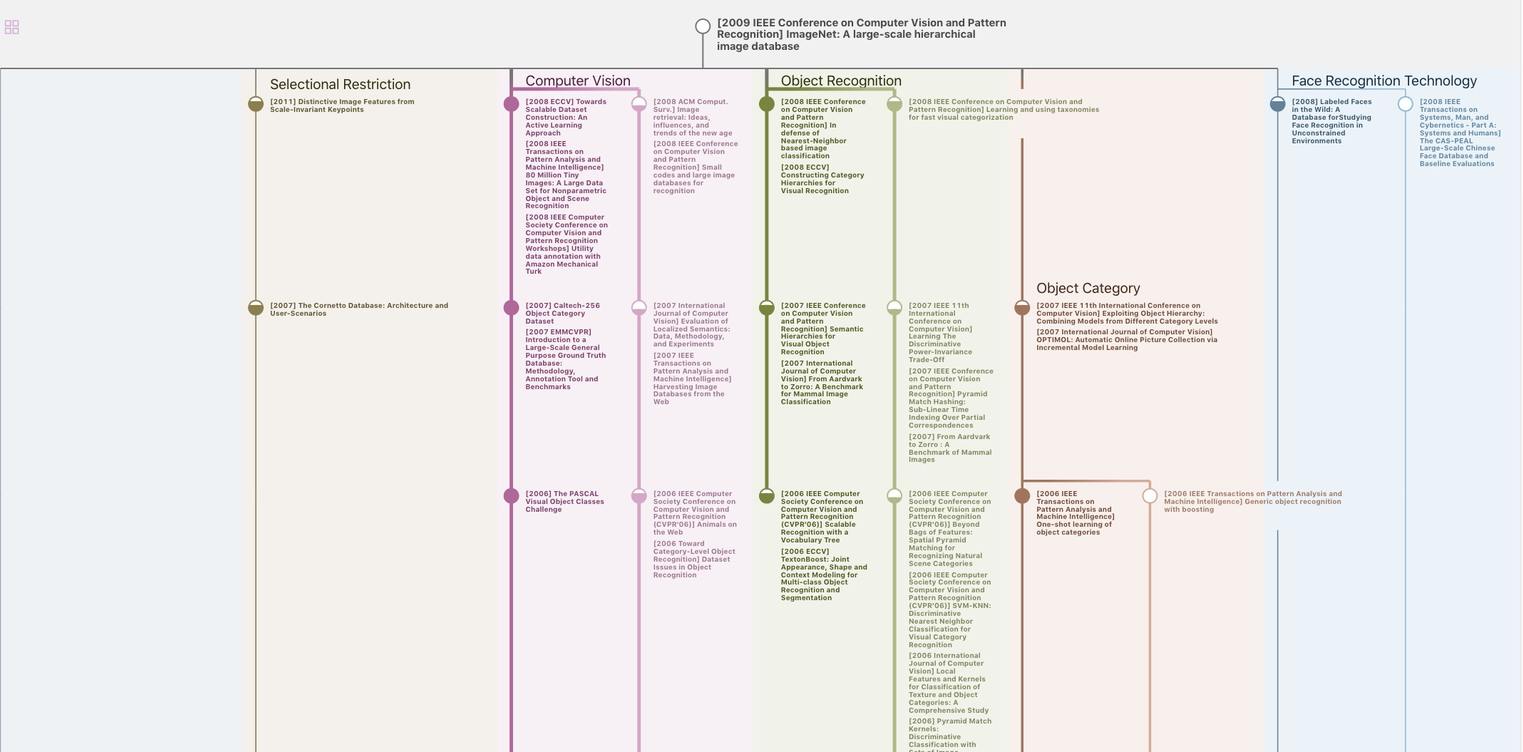
生成溯源树,研究论文发展脉络
Chat Paper
正在生成论文摘要