Predicting asphaltene adsorption on Fe3O4 nanoparticle using machine learning algorithms
Arabian Journal of Geosciences(2024)
摘要
Asphaltene precipitation and its subsequent deposition are considered as one of the most problematic issues in the oil industry because it cause frequent production interruptions and shutdowns and require highly technical and expensive methods for treatment. The problem could arise anywhere in the hydrocarbon production system, thus making it extremely difficult to treat. Though various solutions have been applied for treatment in past, the application of nanoparticles has emerged as one of the most recent and effective treatment methods. To date, nanotechnology in this field is in the experimental phase, and very few field applications exist. Nanoparticles due to their exceptionally high surface area and strong affinity towards asphaltenes causes to adsorbed its high quantity and thus prevent asphaltenes deposition. Therefore, the purpose of this study is to predict the asphaltene adsorption on Fe3O4 nanoparticles using machine learning (ML) algorithms including gradient boosting machine (GBM), decision tree (DT), and random forest (RF). Nanoparticles can quickly adsorb large asphaltene amounts and thus prevent it from deposition. Therefore, this research on adsorption was of utmost importance in terms of engineering concerns. Intelligent models were developed using data presented in the literature, regarding asphaltene adsorption on Fe3O4 nanoparticles. The prediction of models was compared with the experimental results. GBM is found to be more accurate than other models, as proved by the observation of applied statistical metrics both in the training and testing phase. All three applied models, took the weight of concentration equilibrium highest (0.75–0.80) followed by the surface area of inhibitor (0.145–0.094), Temperature (0.077–0.058) and inhibitor dosage (0.064–0.053) respectively during the training process. It was also observed that mostly concentration equilibrium, surface area, and inhibitor dosage support the prediction for all models while temperature contradicts the prediction process.
更多查看译文
关键词
Nanoparticle,Adsorption,Asphaltene,Machine learning Algorithm,Prediction
AI 理解论文
溯源树
样例
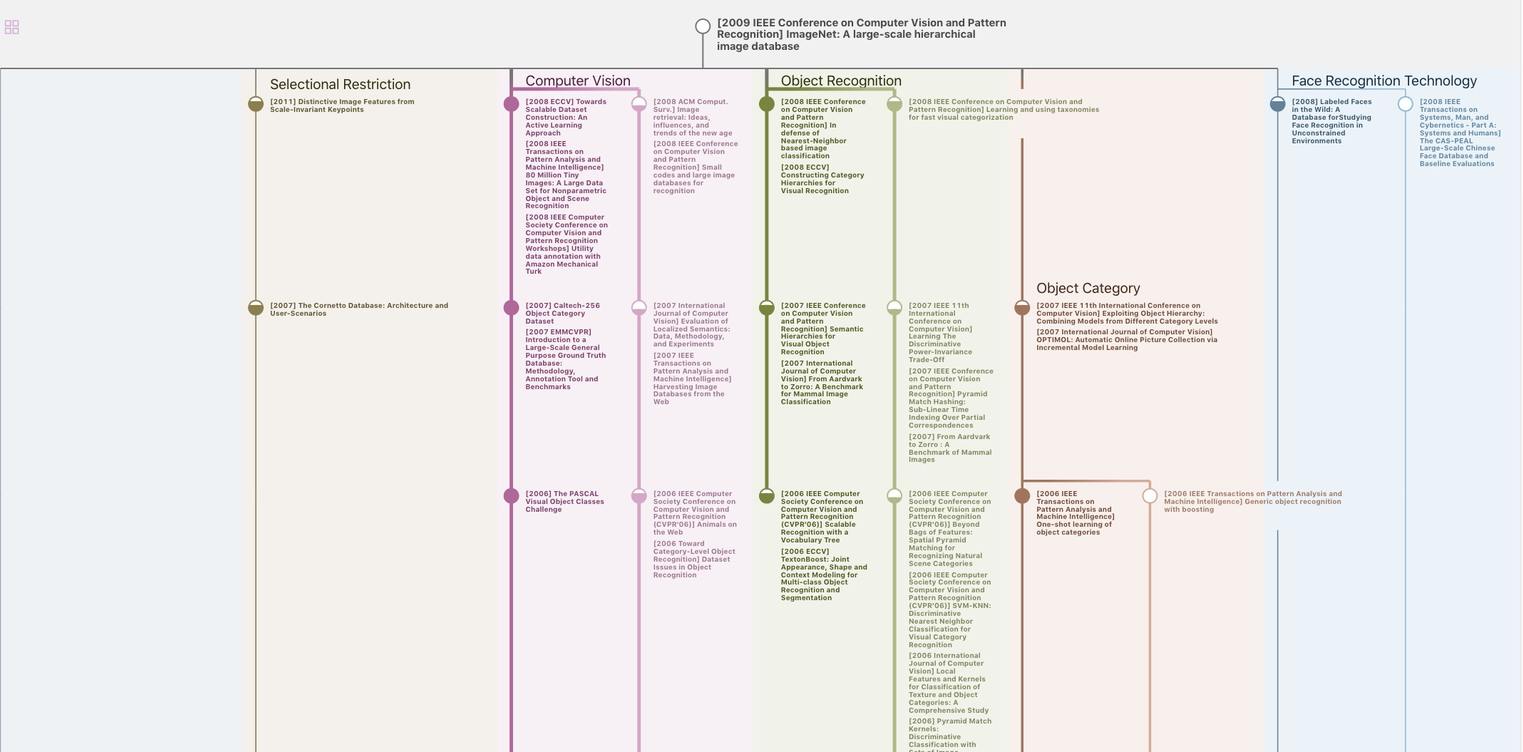
生成溯源树,研究论文发展脉络
Chat Paper
正在生成论文摘要