Decoding Missense Variants by Incorporating Phase Separation via Machine Learning
crossref(2024)
摘要
Computational models have made significant progress in predicting the effect of protein variants. However, deciphering numerous variants of unknown significance (VUS) located within intrinsically disordered regions (IDRs) remains challenging. To address this issue, we introduced liquid-liquid phase separation (LLPS), which is tightly linked to IDRs, into the analysis of missense variants. LLPS is vital for multiple physiological processes. By leveraging missense variants that alter phase separation propensity, we developed an innovative machine-learning approach called PSMutPred to predict the impact of missense mutations on phase separation. PSMutPred shows robust performance in predicting missense variants that affect natural phase separation. In vitro experiment results further underscore its validity. By applying PSMutPred to over 522,000 ClinVar missense variants, we identified that PSMutPred significantly contributes to decoding the pathogenesis of disease variants, especially those in IDRs. Our work provides invaluable insights into the understanding of a vast number of VUSs in IDRs, thereby accelerating clinical interpretation and diagnosis of disease variants.
### Competing Interest Statement
The authors have declared no competing interest.
更多查看译文
AI 理解论文
溯源树
样例
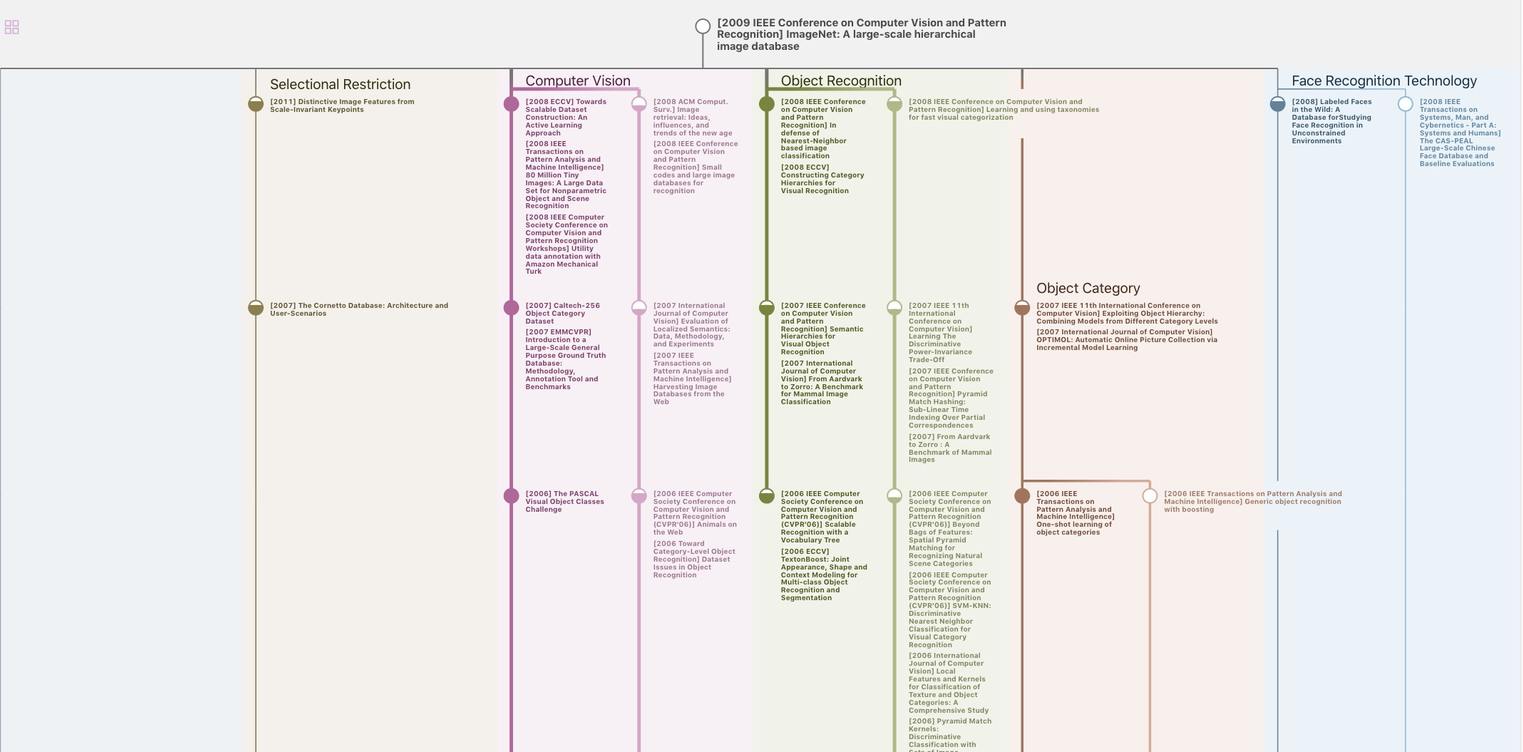
生成溯源树,研究论文发展脉络
Chat Paper
正在生成论文摘要