Predicting the dynamic behavior of a magnetocaloric cooling prototype via artificial neural networks
Applied Thermal Engineering(2024)
摘要
Although magnetocaloric cooling is considered a promising long-term alternative to vapor compression, recent prototype developments have not yet made this technology commercially competitive, primarily due to its high energy consumption and lack of cost-effective, long-term mechanically-chemically stable materials. To address the first issue and understand how the efficiency of magnetocaloric systems can be improved, dynamic models can offer valuable insights into their transient operation. This work focuses on the development of an artificial neural network with experimental data to model the dynamic operation of a magnetic refrigeration system. Through a design of experiments approach, we propose excitation signals for the identification experiment, involving five manipulated variables and one selected disturbance as inputs, with the output temperature of the cold manifold and power consumption as the target parameters. We chose a nonlinear autoregressive artificial neural network with exogenous inputs to model the transient operation of the system. The temperature model achieved R2 values of 0.995 and 0.955 for the 1-step and 90-step ahead predictions, respectively. Similarly, the power consumption model achieved R2 values of 0.988 and 0.949 for the 1-step and 90-step ahead predictions, respectively. These performance metrics were evaluated on the test sets that were not used for training the models, highlighting the robustness and accuracy of the models in both short-term and long-term predictions.
更多查看译文
关键词
Magnetocaloric refrigeration,Artificial Intelligence,Machine learning,Recurrent neural networks,Active magnetic regenerator
AI 理解论文
溯源树
样例
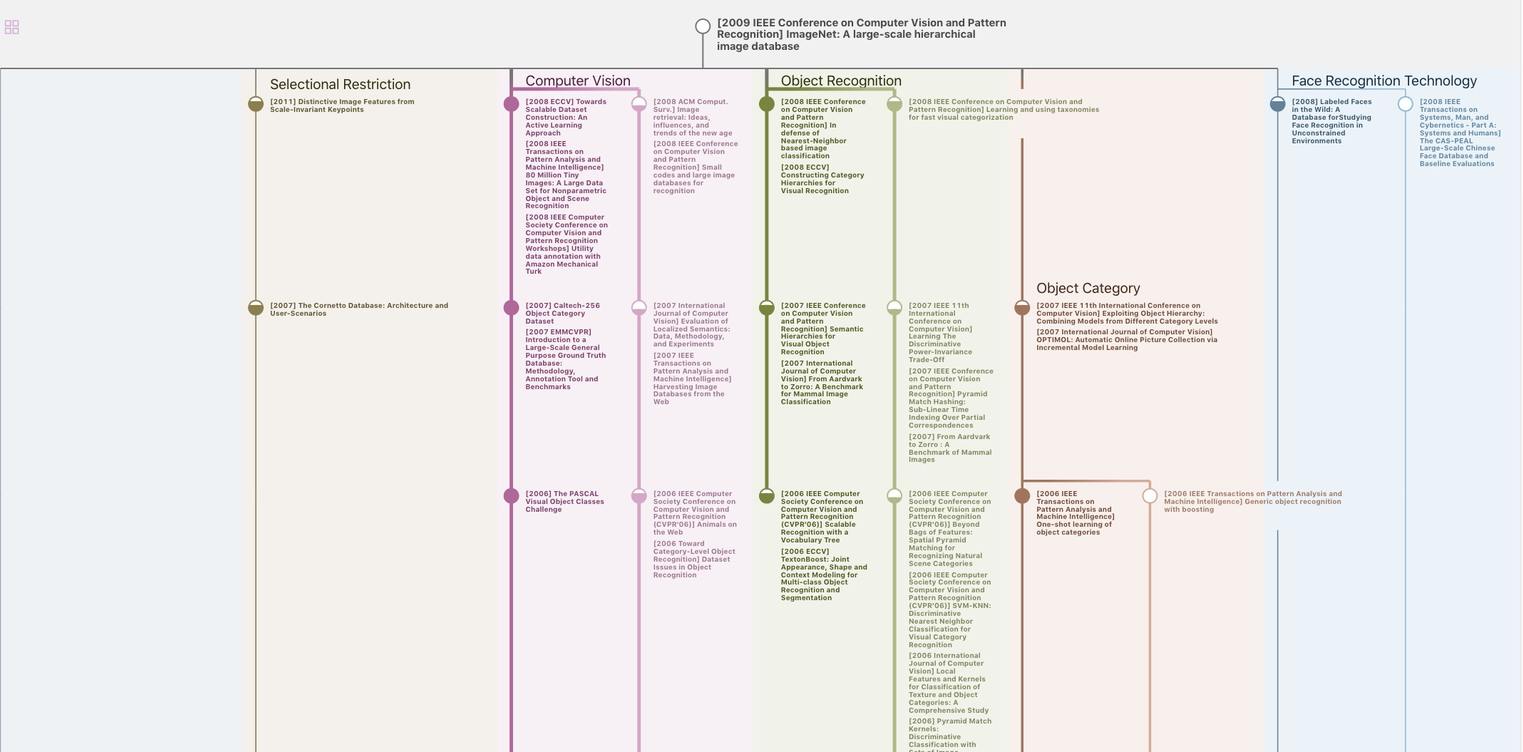
生成溯源树,研究论文发展脉络
Chat Paper
正在生成论文摘要