Deep Learning for AGILE Anticoincidence System's Background Prediction from Orbital and Attitude Parameters
arxiv(2024)
摘要
AGILE is an Italian Space Agency (ASI) space mission launched in 2007 to
study X-ray and gamma-ray phenomena in the energy range from ∼20 keV to
∼10 GeV. The AGILE AntiCoincidence System (ACS) detects hard-X photons in
the 50 - 200 keV energy range and continuously stores each panel's count rates
in the telemetry. We developed a new Deep Learning (DL) model to predict the
background of the AGILE ACS top panel using the satellite's orbital and
attitude parameters. This model aims to learn how the orbital and spinning
modulations of the satellite impact the background level of the ACS top panel.
The DL model executes a regression problem, and is trained with a supervised
learning technique on a dataset larger than twenty million orbital parameters'
configurations. Using a test dataset, we evaluated the trained model by
comparison of the predicted count rates with the real ones. The results show
that the model can reconstruct the background count rates of the ACS top panel
with an accuracy of 96.7%, considering the orbital modulation and spinning of
the satellite. Starting from these promising results, we are developing an
anomaly detection method to detect Gamma-ray Bursts when the differences
between predicted and real count rates exceed a predefined threshold.
更多查看译文
AI 理解论文
溯源树
样例
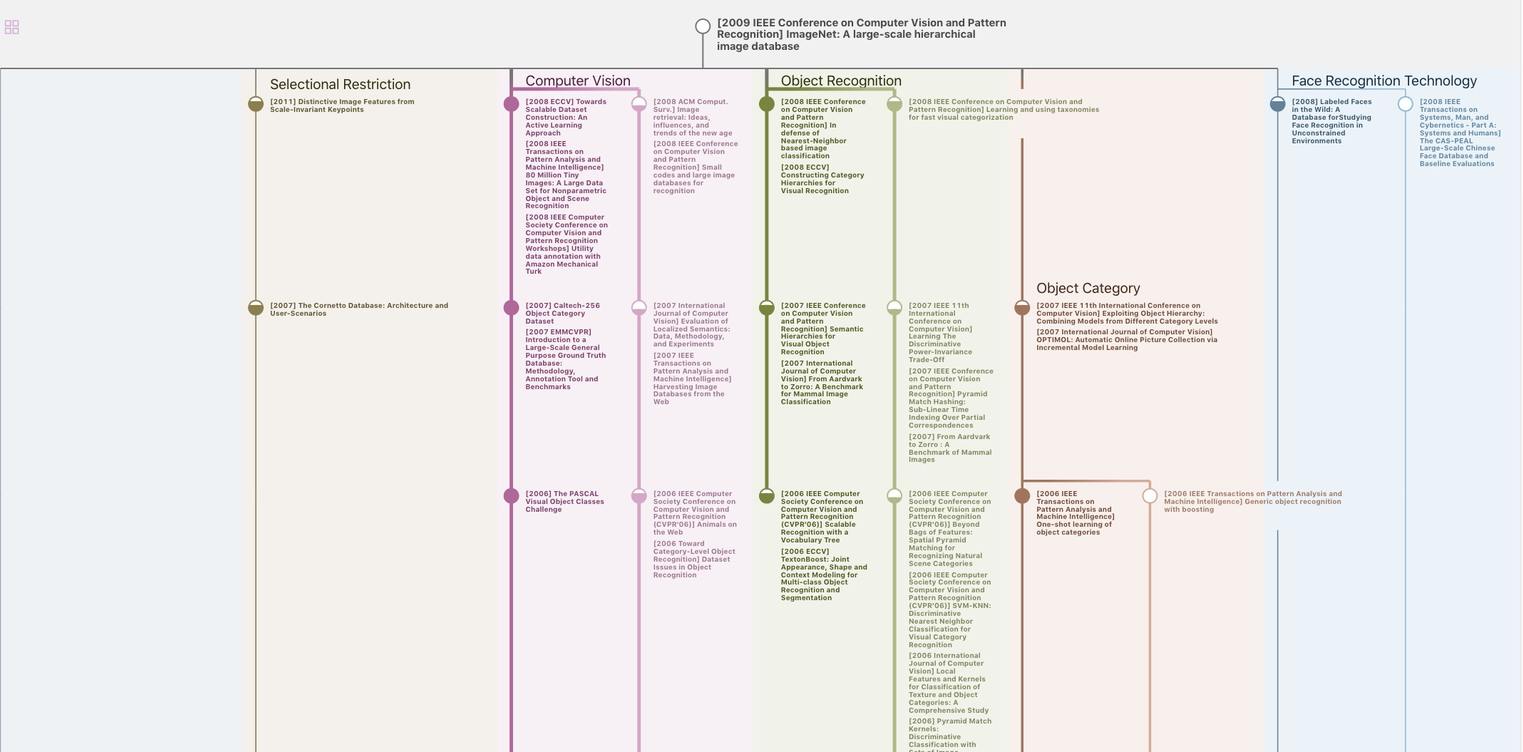
生成溯源树,研究论文发展脉络
Chat Paper
正在生成论文摘要