InsightLens: Discovering and Exploring Insights from Conversational Contexts in Large-Language-Model-Powered Data Analysis
arxiv(2024)
摘要
The proliferation of large language models (LLMs) has revolutionized the
capabilities of natural language interfaces (NLIs) for data analysis. LLMs can
perform multi-step and complex reasoning to generate data insights based on
users' analytic intents. However, these insights often entangle with an
abundance of contexts in analytic conversations such as code, visualizations,
and natural language explanations. This hinders efficient identification,
verification, and interpretation of insights within the current chat-based
interfaces of LLMs. In this paper, we first conduct a formative study with
eight experienced data analysts to understand their general workflow and pain
points during LLM-powered data analysis. Then, we propose an LLM-based
multi-agent framework to automatically extract, associate, and organize
insights along with the analysis process. Based on this, we introduce
InsightLens, an interactive system that visualizes the intricate conversational
contexts from multiple aspects to facilitate insight discovery and exploration.
A user study with twelve data analysts demonstrates the effectiveness of
InsightLens, showing that it significantly reduces users' manual and cognitive
effort without disrupting their conversational data analysis workflow, leading
to a more efficient analysis experience.
更多查看译文
AI 理解论文
溯源树
样例
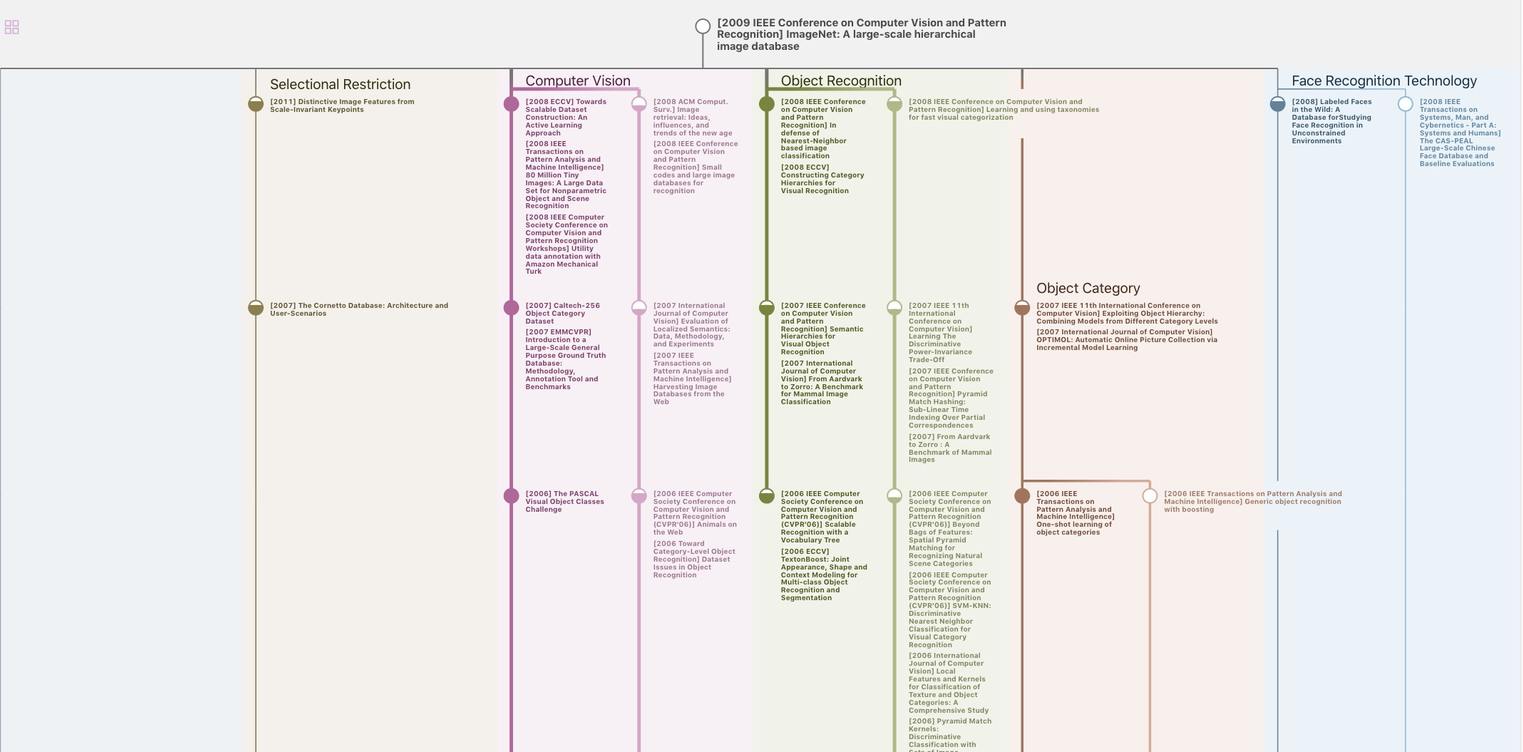
生成溯源树,研究论文发展脉络
Chat Paper
正在生成论文摘要