Unraveling human social behavior motivations via inverse reinforcement learning-based link prediction
Computing(2024)
摘要
Link prediction aims to capture the evolution of network structure, especially in real social networks, which is conducive to friend recommendations, human contact trajectory simulation, and more. However, the challenge of the stochastic social behaviors and the unstable space-time distribution in such networks often leads to unexplainable and inaccurate link predictions. Therefore, taking inspiration from the success of imitation learning in simulating human driver behavior, we propose a dynamic network link prediction method based on inverse reinforcement learning (DN-IRL) to unravel the motivations behind social behaviors in social networks. Specifically, the historical social behaviors (link sequences) and a next behavior (a single link) are regarded as the current environmental state and the action taken by the agent, respectively. Subsequently, the reward function, which is designed to maximize the cumulative expected reward from expert behaviors in the raw data, is optimized and utilized to learn the agent’s social policy. Furthermore, our approach incorporates the neighborhood structure based node embedding and the self-attention modules, enabling sensitivity to network structure and traceability to predicted links. Experimental results on real-world dynamic social networks demonstrate that DN-IRL achieves more accurate and explainable of prediction compared to the baselines.
更多查看译文
关键词
Social network,Link prediction,Inverse reinforcement learning,Node embedding method,68T42t
AI 理解论文
溯源树
样例
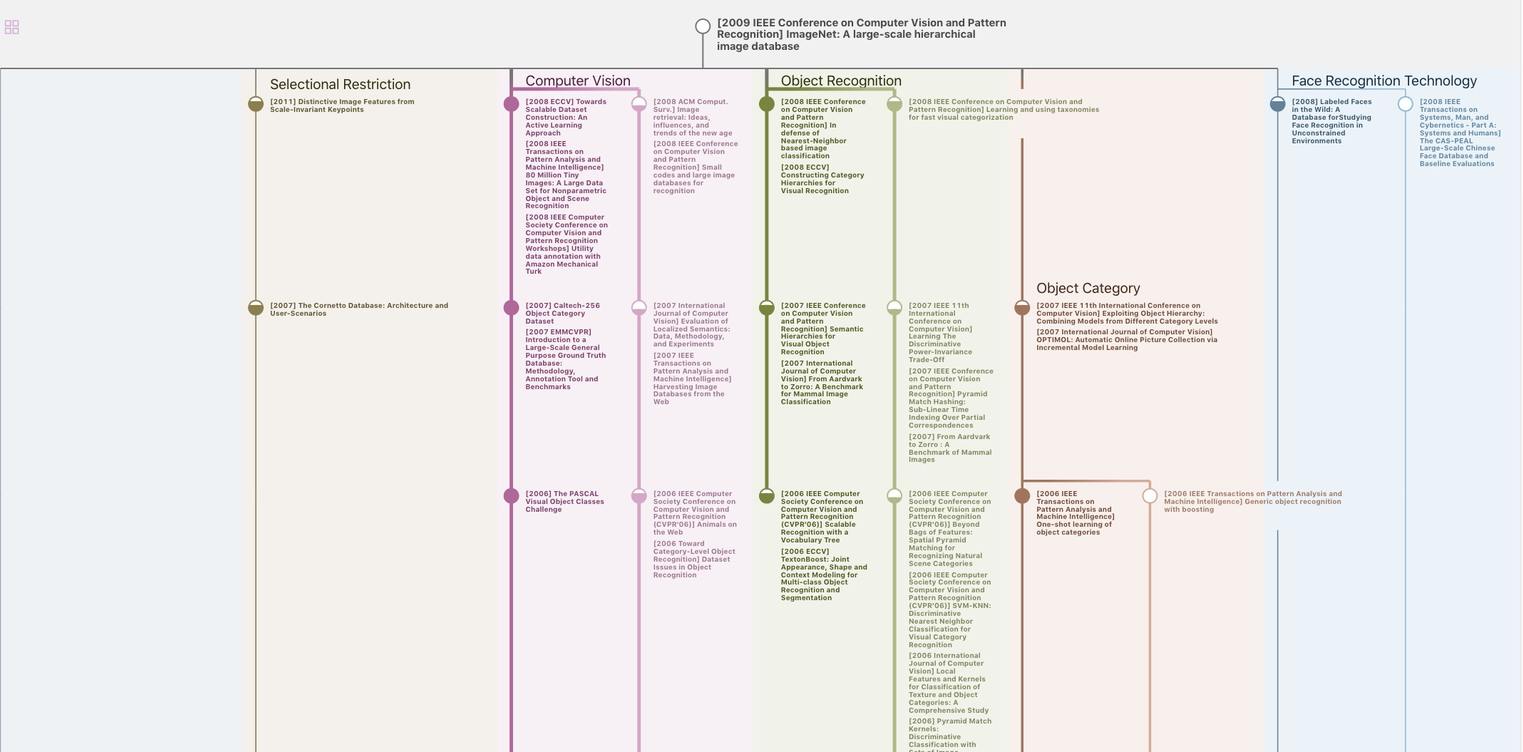
生成溯源树,研究论文发展脉络
Chat Paper
正在生成论文摘要