FGSSAT : Unsupervised Fine-Grain Attribution of Unknown Speech Synthesizers Using Transformer Networks.
Asilomar Conference on Signals, Systems and Computers(2023)
摘要
Synthetic speech generators can produce high quality speech. It can be difficult for humans to perceptually distinguish between synthesized speech and authentic human speech. Identifying the synthesizer used for generating synthetic speech, known as synthetic speech attribution, is an important problem. An open problem in synthetic speech attribution is attributing speech to new, unknown synthesizers, which are not present in the training set. Existing methods can identify known speech synthesizers but they cannot differentiate an unknown synthesizer from another unknown synthesizer. In this paper, we describe a system for attribution of unknown synthesizers i.e., assigning different labels to different unknown synthesizers. Our system is known as Fine-Grain Synthetic Speech Attribution Transformer (FGSSAT). FGSSAT is unsupervised and uses transformer, dimensionality reduction and clustering for attribution. Our experiments use the ASVspoof2019 dataset. We train on real speech and 6 synthesizers and evaluate on real speech and 17 synthesizers, which include 11 unknown synthesizers. FGSSAT identifies known synthesizers with 99.6% accuracy and classifies all speech generated from unknown synthesizers with 76.5% accuracy, which is an improvement on existing work.
更多查看译文
关键词
Synthetic speech attribution,speech forensics,deepfake speech,transformer,unsupervised clustering
AI 理解论文
溯源树
样例
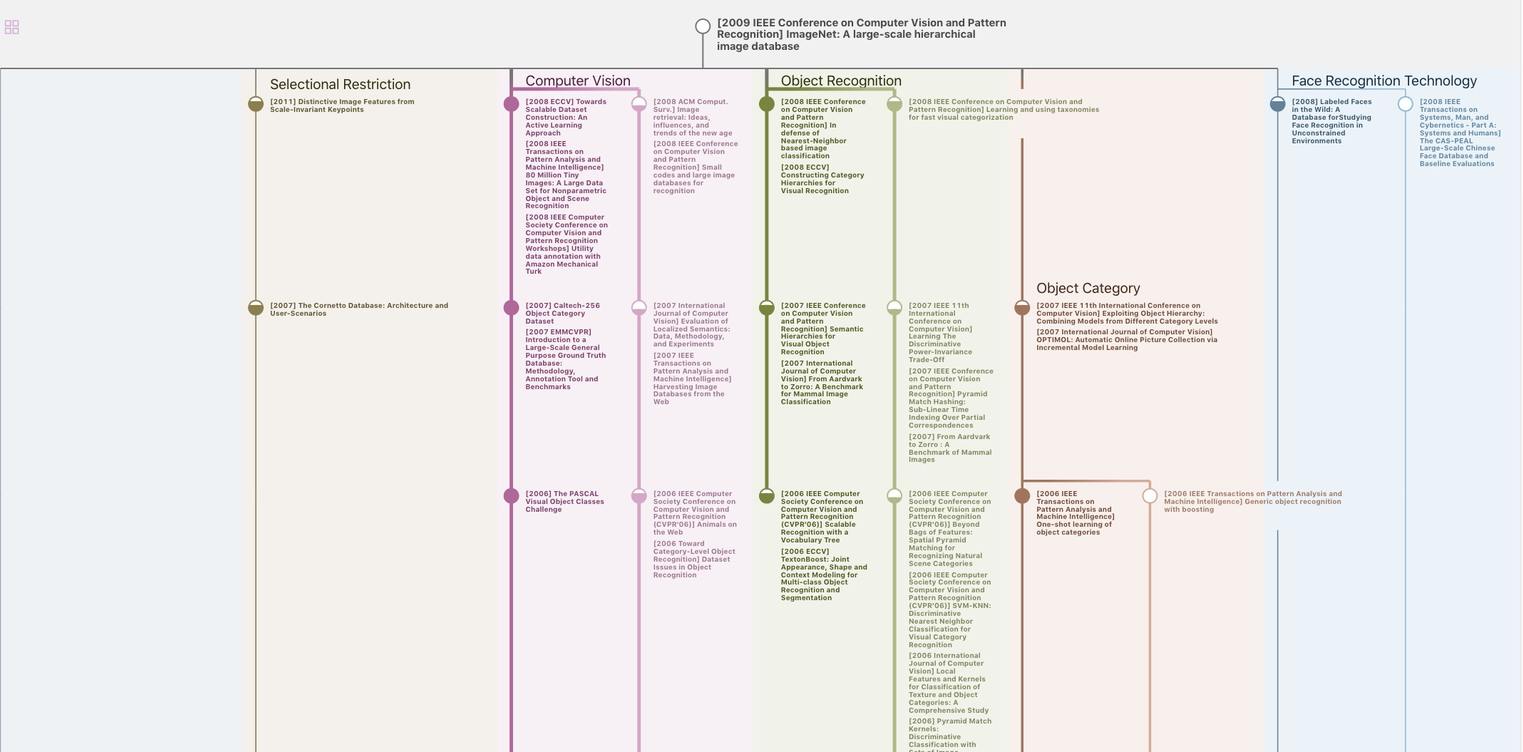
生成溯源树,研究论文发展脉络
Chat Paper
正在生成论文摘要