Homomorphic Evaluation Friendly Vision Transformer Design.
Asilomar Conference on Signals, Systems and Computers(2023)
摘要
The widespread adoption of neural networks has yielded remarkable success across diverse tasks. However, the increasing security concerns associated with state-of-the-art model deployment underscore the need for Privacy-Preserving Machine Learning (PPML). Homomorphic Encryption (HE) is one of the popular security primitives that empower PPML. Such primitives allow the secure computation over ciphertext without decryption and send the result back to the data owner, protecting the privacy of user data. In this work, we explore the possible solutions to apply the trending vision transformer in the homomorphic domain by modifying the nonlinear activation functions to make them compatible with homomorphic-friendly operations.
更多查看译文
关键词
Neural Network,Homomorphic Encryption,Privacy-Preserving Machine learning,Vision Transformer
AI 理解论文
溯源树
样例
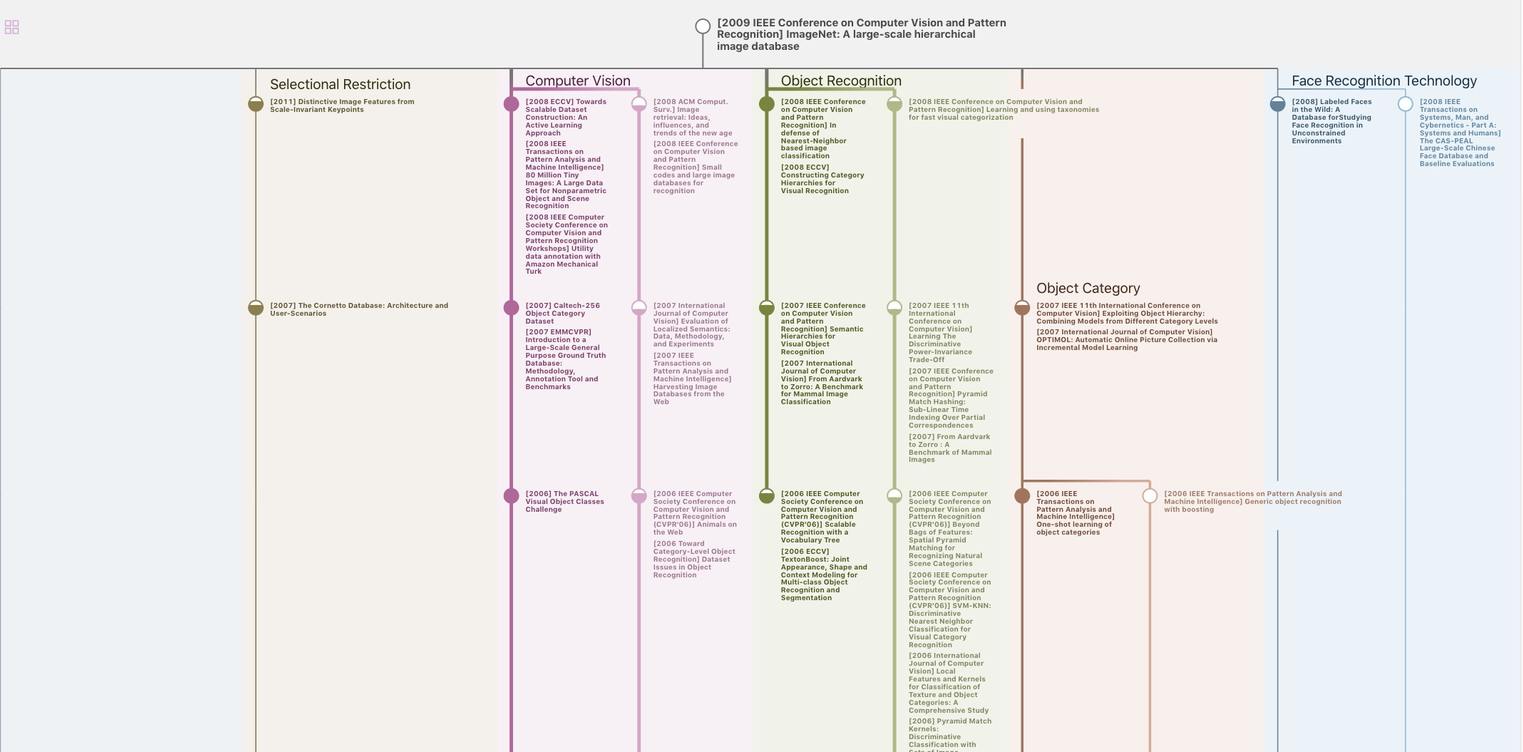
生成溯源树,研究论文发展脉络
Chat Paper
正在生成论文摘要