Complexity-Optimized Sample Selection for Fast Adaptation of Digital Predistorter
IEEE Transactions on Microwave Theory and Techniques(2024)
摘要
Sample selection methods have recently achieved success in low-complexity digital predistortion (DPD) parameter identification. However, obtaining appropriate sample sets still requires either large computing costs or careful tuning of hyperparameters. In this research, we present some novel and simplified strategies for selecting suitable samples in few-sample learning (FSL) DPD. First, as current approaches mostly rely on the maximum distance in the feature space, we provide a new centroid-based initialization method. This method effectively decreases the computational complexity and storage resource usage during sample selection. Then, in order to enhance the effectiveness of distinguishing samples, we suggest substituting the conventional Euclidean distance with the Manhattan distance. This modification enhances the robustness of sample selection to a certain degree, while eliminating the need for any multiplication operations. Finally, a memory feature weighting procedure has been devised to more accurately characterize the gradual decline of memory effects in radio frequency (RF) power amplifiers (PAs). Experimental results confirm that the suggested methods can improve the linearity performance of FSL DPD and facilitate rapid adjustment of the digital predistorter.
更多查看译文
关键词
Behavior modeling,digital predistortion (DPD),few-sample learning (FSL),linearization of power amplifiers (PAs),low complexity,sample selection
AI 理解论文
溯源树
样例
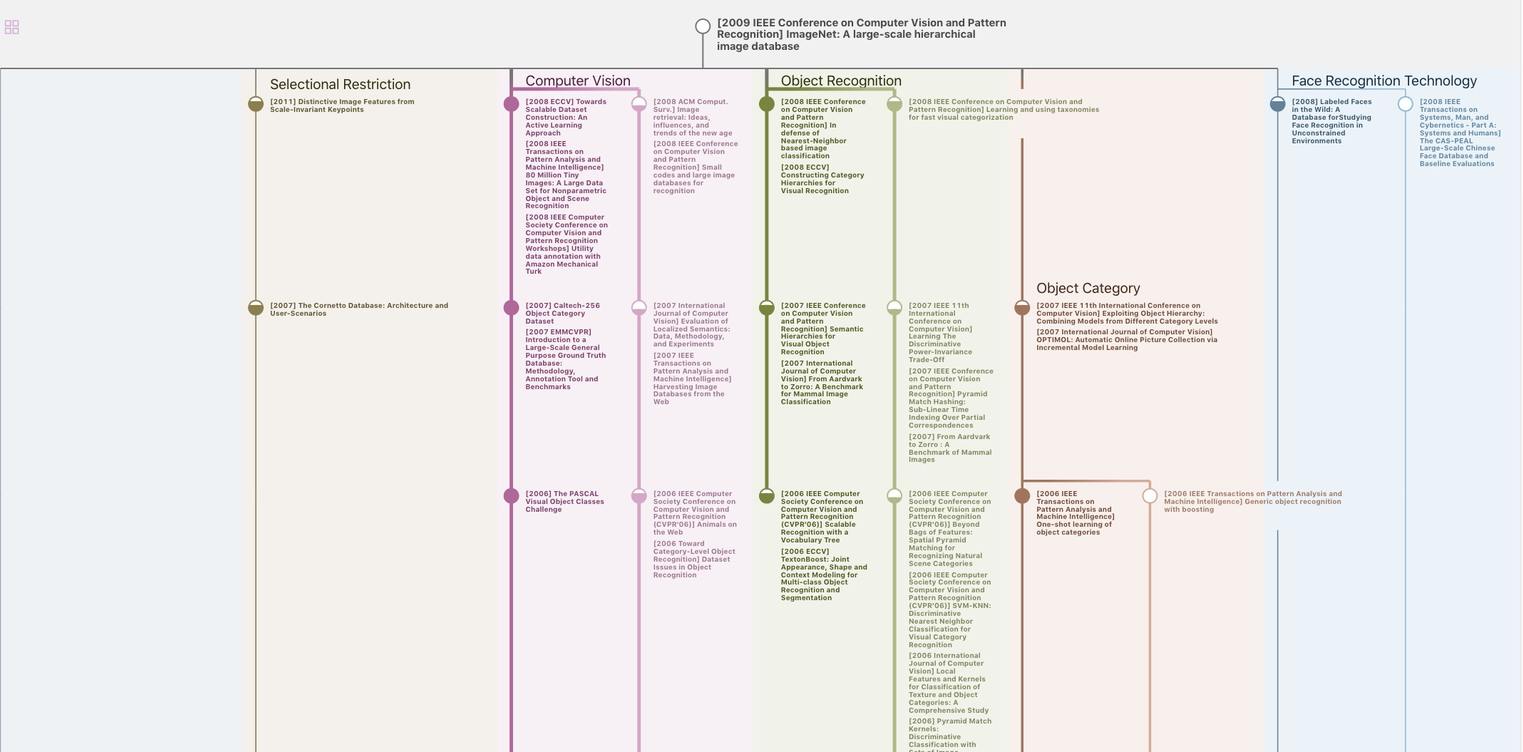
生成溯源树,研究论文发展脉络
Chat Paper
正在生成论文摘要