On the asymptotic linear convergence of gradient descent for non-symmetric matrix completion.
Asilomar Conference on Signals, Systems and Computers(2023)
摘要
This paper studies a factorization-based gradient descent approach for non-symmetric matrix completion. We introduce an objective that includes an orthogonality regularization for one of the factors. Additionally, we introduce a scaling term to ensure that the two factors are of equal magnitude to improve the convergence speed. For the proposed objective, we analyze the exact linear convergence rate of gradient descent via the asymptotically linear update equation for the error matrix. Our proposed result is the first closed-form expression of the exact linear rate. To illustrate the correctness and tightness of our analysis, we compare the empirical convergence rate against the analytical rate. Additional numerical experiments are done to verify the efficacy of the scaling approach.
更多查看译文
关键词
Gradient Descent,Convergence Rate,Matrix Completion,Numerical Experiments,Linear Rate,Error Matrix,Exact Rate,Gradient Descent Approach,Step Size,Error Term,Symmetric Matrix,Standard Normal Distribution,Structural Constraints,Convergence Of Algorithm,Orthogonal Matrix,Rank Of Matrix,Space Of Matrices,Tangent Space,Permutation Matrix,Local Convergence,Asymptotic Rate,Objective Terms,Rank Constraint
AI 理解论文
溯源树
样例
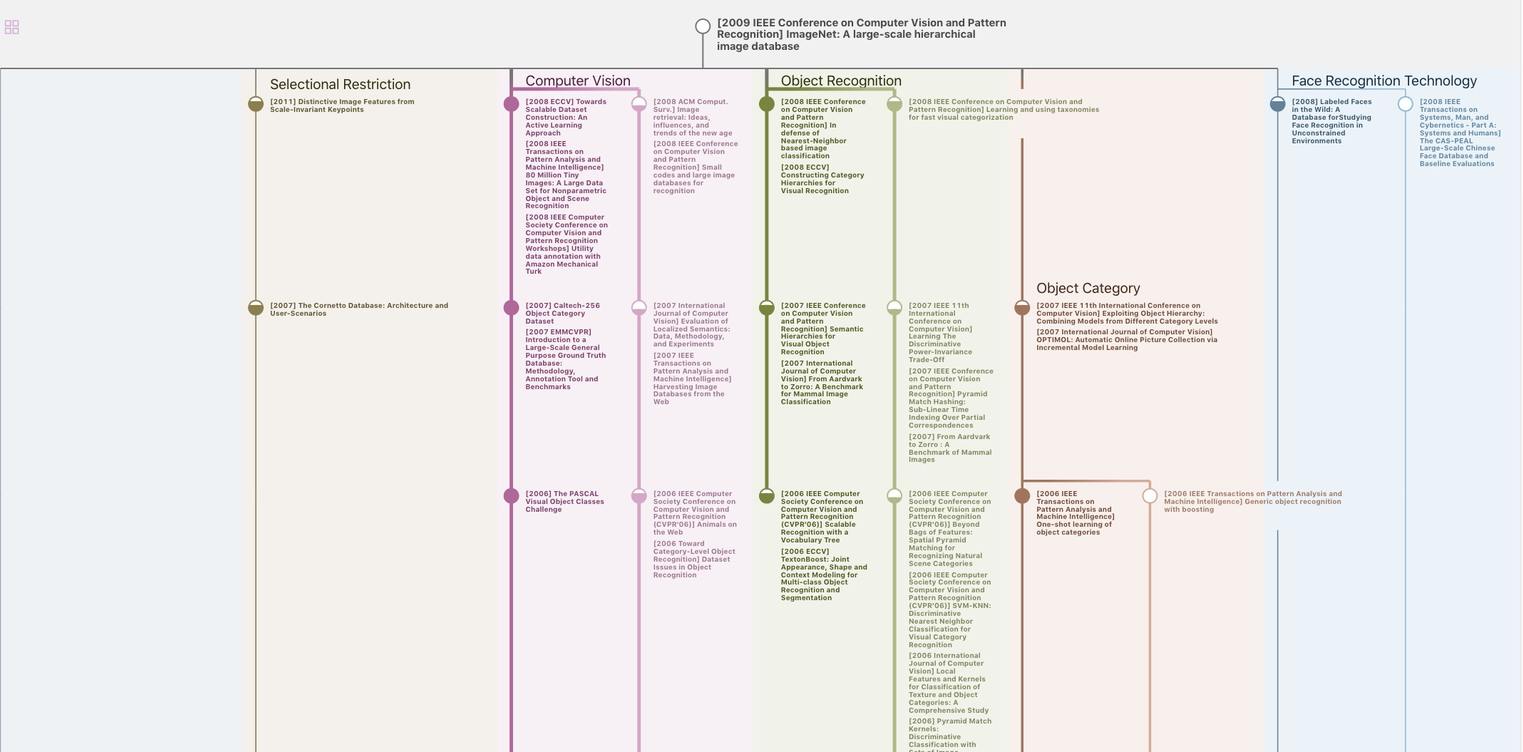
生成溯源树,研究论文发展脉络
Chat Paper
正在生成论文摘要