Novel Node Category Detection Under Subpopulation Shift
CoRR(2024)
Abstract
In real-world graph data, distribution shifts can manifest in various ways,
such as the emergence of new categories and changes in the relative proportions
of existing categories. It is often important to detect nodes of novel
categories under such distribution shifts for safety or insight discovery
purposes. We introduce a new approach, Recall-Constrained Optimization with
Selective Link Prediction (RECO-SLIP), to detect nodes belonging to novel
categories in attributed graphs under subpopulation shifts. By integrating a
recall-constrained learning framework with a sample-efficient link prediction
mechanism, RECO-SLIP addresses the dual challenges of resilience against
subpopulation shifts and the effective exploitation of graph structure. Our
extensive empirical evaluation across multiple graph datasets demonstrates the
superior performance of RECO-SLIP over existing methods.
MoreTranslated text
AI Read Science
Must-Reading Tree
Example
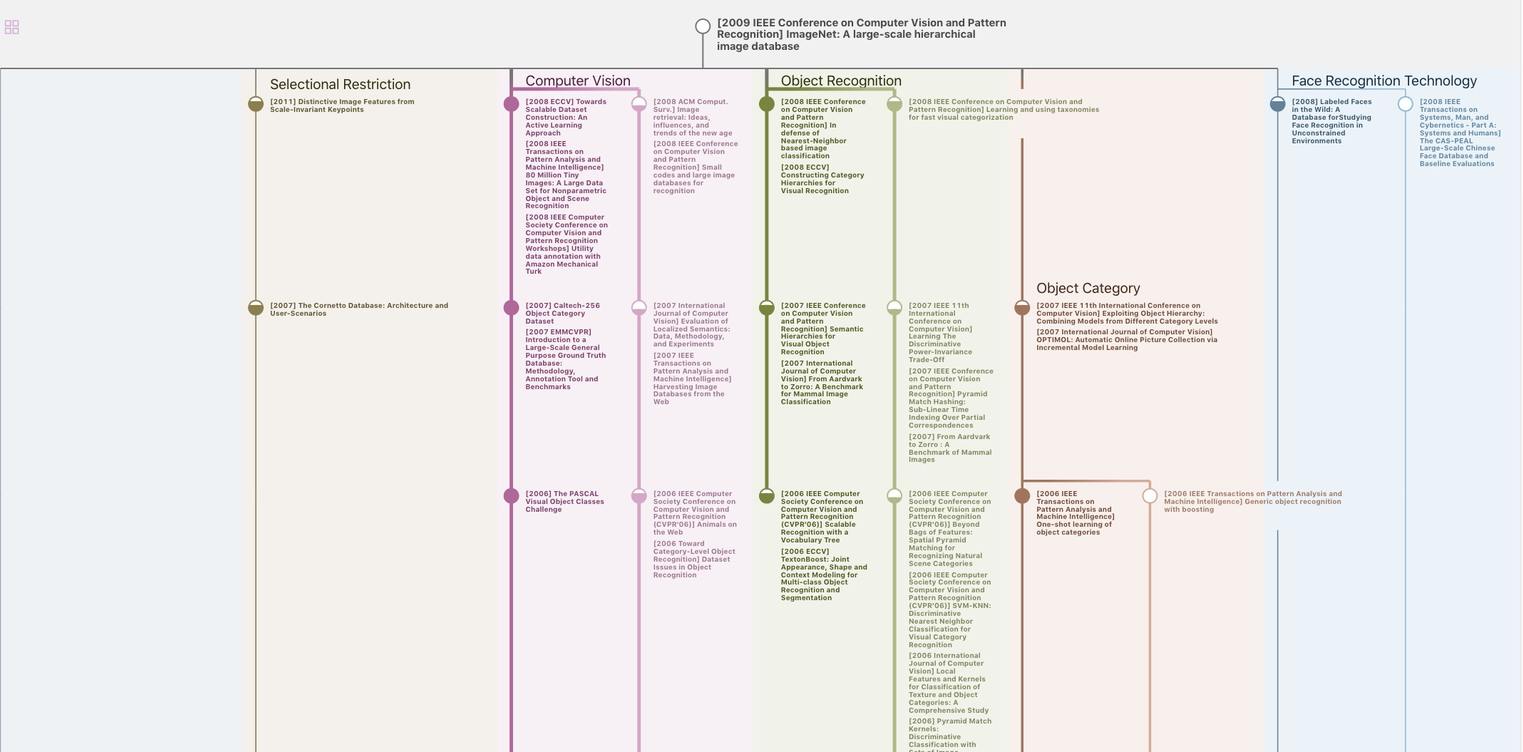
Generate MRT to find the research sequence of this paper
Chat Paper
Summary is being generated by the instructions you defined