Privacy-aware crowd counting by decentralized learning with parallel transformers
INTERNET OF THINGS(2024)
Abstract
With the rapid advancement of deep learning, the performance of crowd counting has improved significantly. Nonetheless, existing crowd counting models primarily depend on a broad dataset gathered from a variety of individuals for model training. However, this diverse dataset comes at the cost of compromising people's privacy. Hence, the need to address privacy concerns when counting crowds in dense scenes is becoming increasingly apparent. To tackle this issue, we propose a novel framework called the Decentralized Learning with Parallel Transformer network (DLPTNet). Based on the federated learning mechanism, the DLPTNet adopts a decentralized learning framework that implements parameter sharing instead of data sharing. The DLPTNet consists of two pivotal modules, namely Halo Attention (HA) module and the Density -aware Transformer (DAT) module. The HA module has a large perception radius, which enhances its ability to perceive the context around the objects and extract more extensive information from local regions to address the occlusion issue in dense scenes. Meanwhile, the DAT module leverages the parallel mechanism of Density -aware Attention (DDA) to further capture long-range dependencies between different positions and thus gains learning of the correlations and density distributions of various regions within dense crowds globally.
MoreTranslated text
Key words
Federated learning,Decentralized learning,Crowd counting,Attention mechanism,Deep learning
AI Read Science
Must-Reading Tree
Example
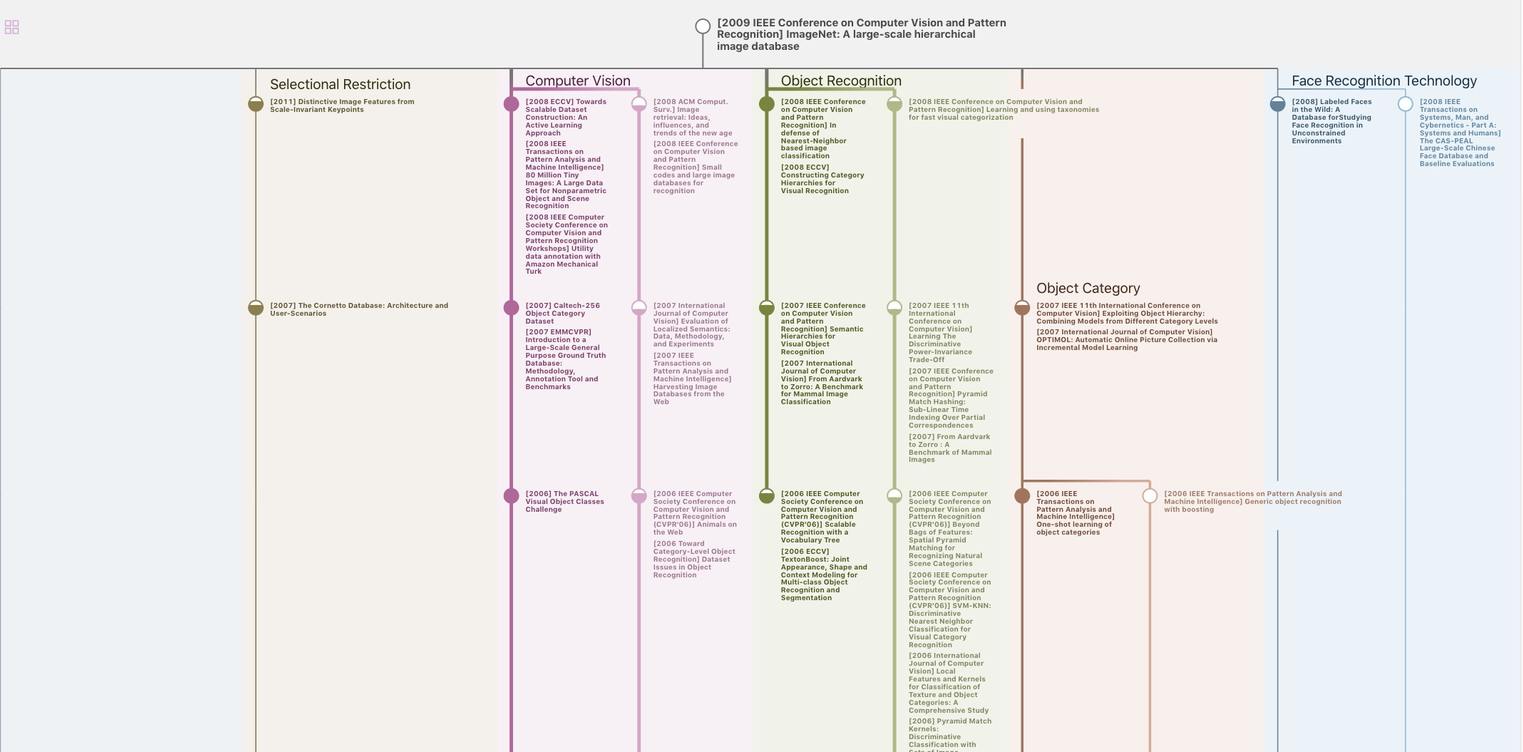
Generate MRT to find the research sequence of this paper
Chat Paper
Summary is being generated by the instructions you defined