Enhancing Cybersecurity: A Review and Comparative Analysis of Convolutional Neural Network Approaches for Detecting URL-Based Phishing Attacks
e-Prime - Advances in Electrical Engineering, Electronics and Energy(2024)
摘要
Phishing attempts to mimic the official websites of businesses, including banks, e-commerce, government offices, and financial institutions. Phishing websites aim to collect and retrieve sensitive data from users, including passwords, credit card numbers, email addresses, personal information, and so on. The growing frequency of phishing attacks has prompted the development of numerous anti-phishing technologies. Because machine learning (ML) techniques perform better in categorization problems, they are used extensively. But the most crucial features are not extracted by the algorithms in use today, which could result in a false categorization. In addition, the complex algorithms contribute to the long reaction time. To solve these issues, this study suggests using a Bidirectional Long Short-Term Memory-based Gated Highway Attention Block Convolutional Neural Network (BiLSTM-GHA-CNN) to detect phishing URLs.
更多查看译文
关键词
URL,phishing,features,neural network,machine learning,deep learning,CNN,BiLSTM,APWG
AI 理解论文
溯源树
样例
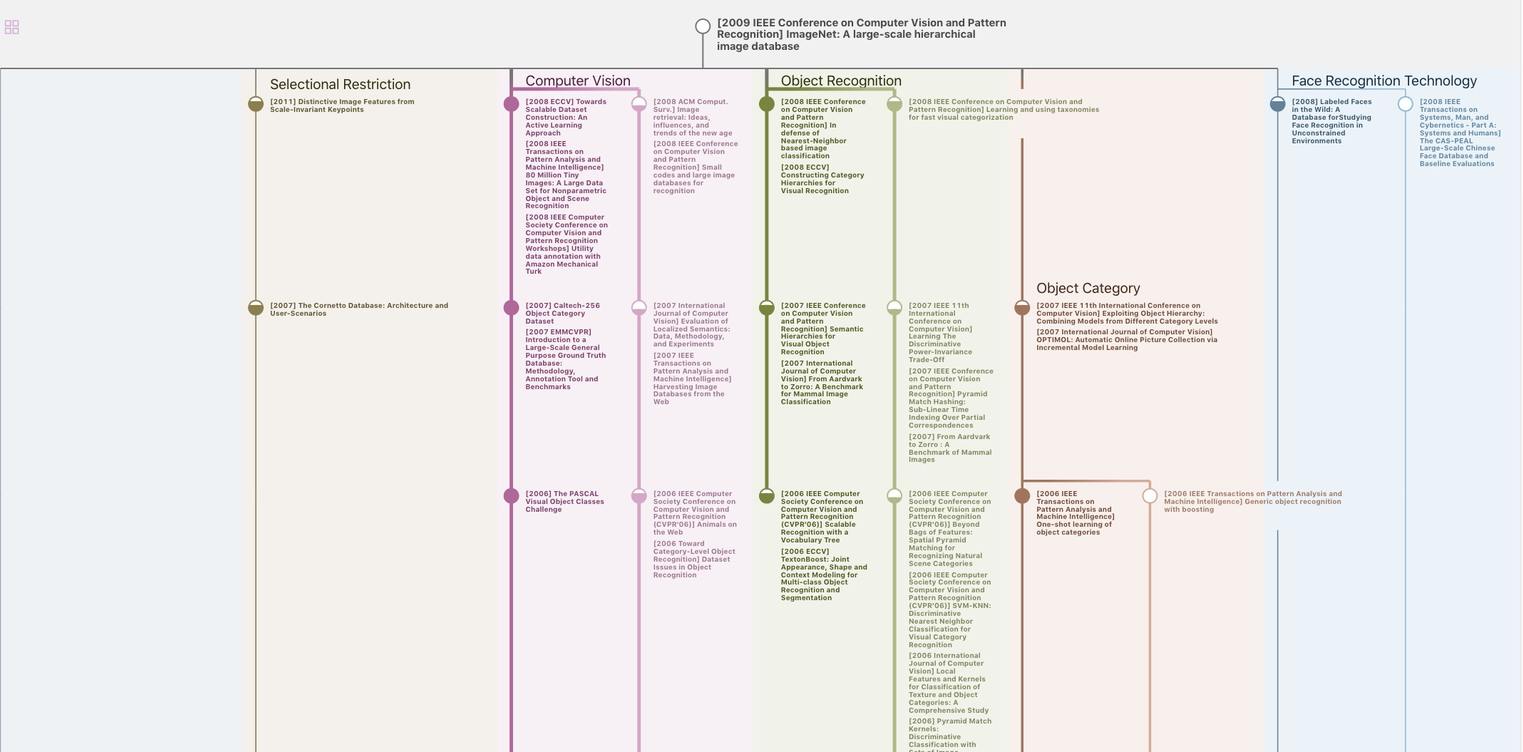
生成溯源树,研究论文发展脉络
Chat Paper
正在生成论文摘要