Probabilistic Topological Models over Simplicial Complexes.
Asilomar Conference on Signals, Systems and Computers(2023)
摘要
Probabilistic graphical models are a fundamen-tal tool for modeling uncertainty and statistical dependencies in various domains, making them indispensable for decision-making, machine learning, and understanding complex systems. Graphical models provide a formal tool to capture the statistical dependencies among a set of random variables, where each vertex of the graph is associated to a variable whereas each edge carries information about the conditional independence between the random variables associated to its endpoints, given all the other random variables. However, there are many situations in which each random variable is associated to a pair of nodes or, more generally, to a subset of entities of cardinality grater than two. In this paper, we generalize graphical models to deal with these situations and provide algorithms to capture the conditional dependencies from this kind of data.
更多查看译文
关键词
Topological lasso,topology inference,topological signal processing,probabilistic topological models,simplicial complexes
AI 理解论文
溯源树
样例
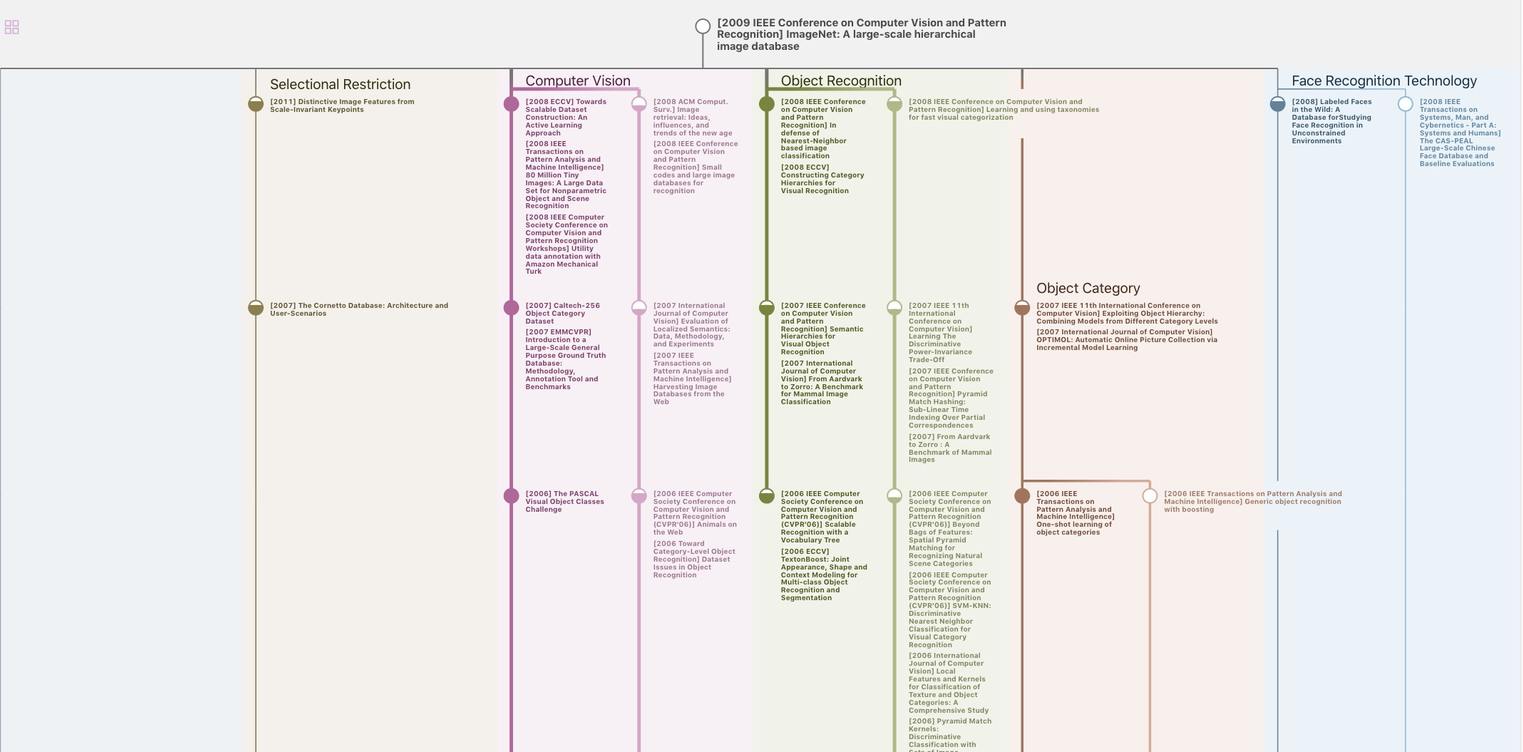
生成溯源树,研究论文发展脉络
Chat Paper
正在生成论文摘要